A soft introduction to rough volatility
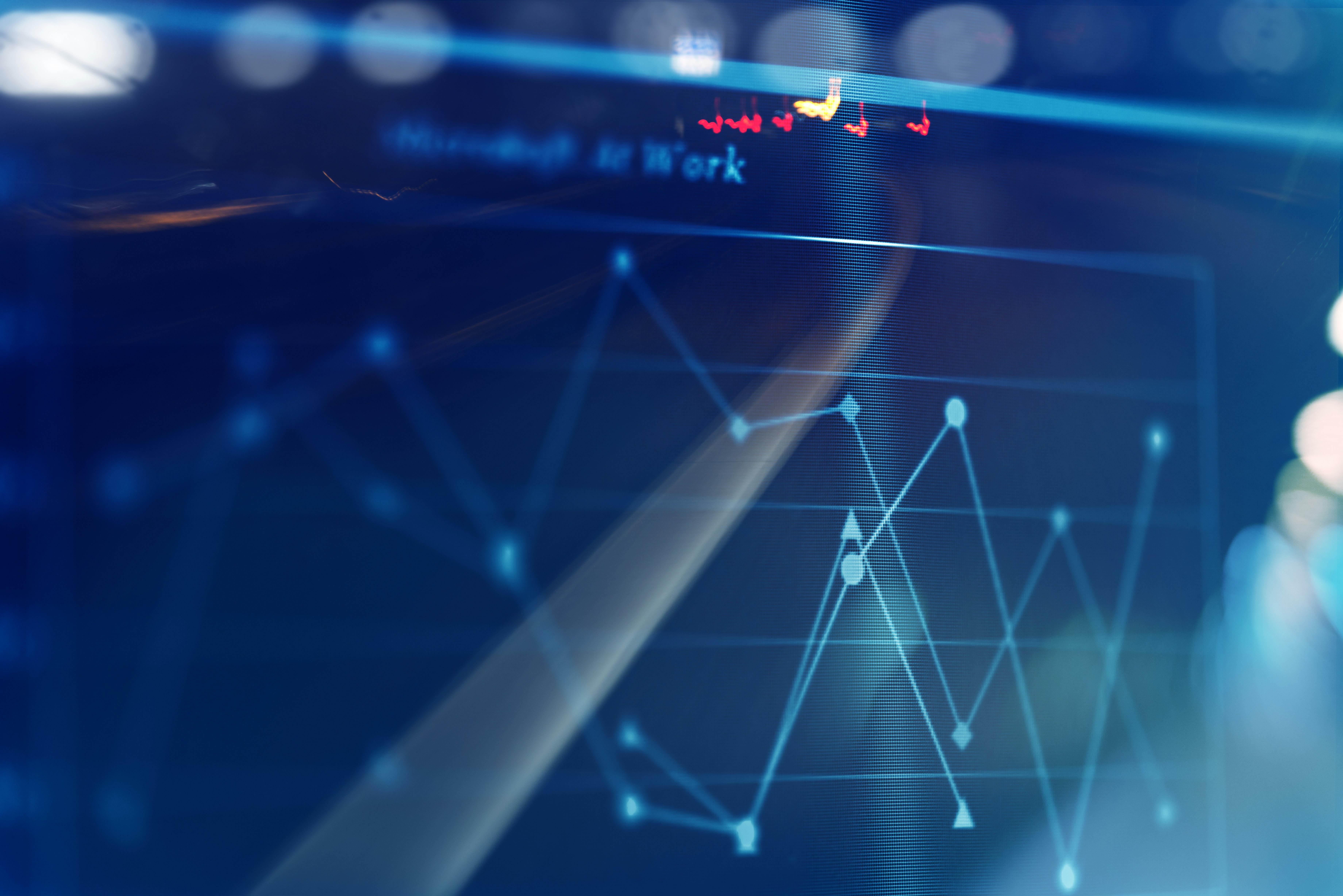
Marcos Carreira is the co-author of Brazilian Derivatives and Securities and a speaker at QuantMinds International 2016 and 2017 conferences. Here, he gives an overview on rough volatility.
It is not uncommon for a theory to influence the behavior of the exact thing that the theory is supposed to model; finance (and options in particular) are a good example (as shown in the excellent “An Engine, Not a Camera”, by Donald MacKenzie).
The advent of electronic markets brought not only a new structure (now it was really possible for a Central Limit Order Book to be enforced), but also more precise intraday data: how prices and spreads behave at each instant; increasing the amount of knowledge available for both market participants and those who study it.
When Mandelbrot studied cotton prices (“The Variation of Certain Speculative Prices”, 1963), he compared daily variations with monthly variations. That was already enough to show that returns on financial assets do not follow a normal distribution; but the Black & Scholes model and the subsequent local and stochastic volatility models used the equivalent of a Brownian motion even though, in Riccardo Rebonato’s words, the implied volatility in the Black & Scholes formula is “the wrong number to put in the wrong formula to get the right price”.
But in this decade a new area of research has emerged, starting with the 2014 paper “Volatility is Rough”, by Jim Gatheral, Thibault Jaisson and Mathieu Rosenbaum. Thanks to the availability of high-frequency data, estimates of the integrated variance over various short-term periods for the whole day can be used as proxies for the (unobservable) spot volatility process; the smoothness of this process can then be estimated.
It is an interesting question whether rough volatility is an inevitable consequence of electronic trading.
The reader can (and should) understand these results, as the methods and data for these results are available at Rough volatility with Python (a Python notebook by Jim Gatheral); in there, one can observe that m(q,Δ), the qth sample moments of the differences in log-volatility at a given lag Δ, are proportional to Δ raised to an exponent ξ(q); this exponent ξ(q) is proportional to q, such that ξ(q)=q*H; this H is close to 0.10 for a variety of financial instruments.
A general formula for fractional Brownian motions (also known as fBm) uses the Hurst index H to determine the nature of the autocovariance of the increments of the process; with H=1/2, we would have the Brownian motion (no autocorrelation between increments); previous econometric analysis of volatility focused on models with H>1/2 and the concept of long memory; but simulating processes with H<1/2 (e.g. H=0.10) gives us realizations much closer to what real high-frequency data looks like, such as fractal-like behavior, volatility clustering and a realistic decay of skew.
The term rough refers to how the paths of these processes with H<1/2 look compared with the Brownian motion paths, hence these models are known as RFSV models, (with RFSV standing for Rough Fractional Stochastic Volatility), with the previous models with H>1/2 being referred to as FSV models.
Further research has applied these results to the pricing of options, by adapting the Heston framework to a rough fractional process (rough Heston) and also by linking it to the market microstructure features associated with high-frequency trading (high degree of endogeneity of market, no-arbitrage property, buying/selling asymmetry and presence of metaorders). It is then an interesting question whether rough volatility is an inevitable consequence of electronic trading.
More resources can be found at the Rough Volatility Network, and QuantMinds International featured several talks on this subject, from Jesper Andreasen‘s “Tough vol” to Mikko Pakkanen‘s “Rough volatility and its applications in FX markets”, closing with Aitor Gonzalez’s “Learning Rough Volatility”. Jim Gatheral showed how to calibrate rough volatility models in his presentation, and Mikko Pakkanen’s work includes a fast Monte Carlo Simulator for rough volatility.
So if you are interested into the latest and most impactful research in volatility, and could not go to Lisbon for the 2018 QuantMinds International, don’t forget to book your place at conference next year to meet the researchers and practitioners shaping the next developments in the field.