Building a deep learning neural network
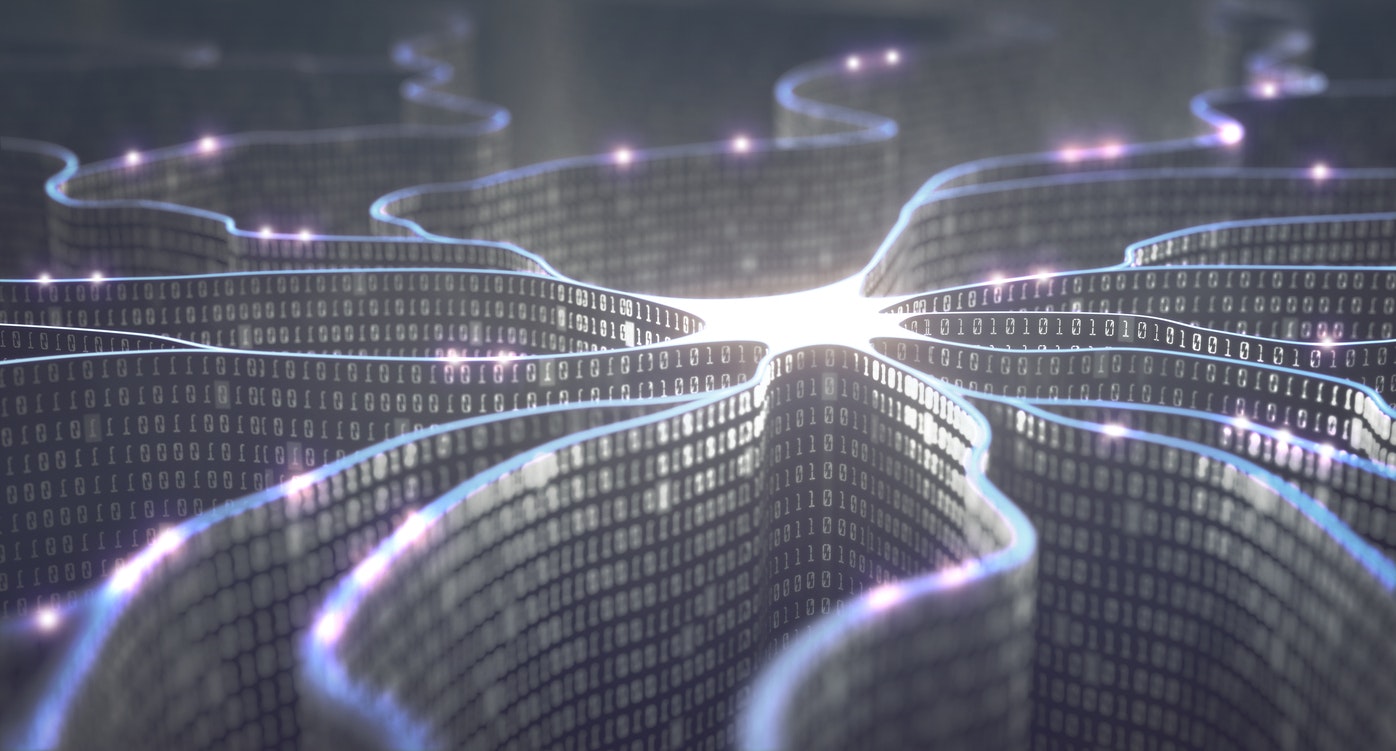
As quantitative finance relies more on machines, how can you ensure that the machine won’t fail you? AI, machine learning, and neural networks still need to be perfected, and B. Horvath, King's College London, A. Muguruza, Natixis, and M. Tomas, École Polytechnique share how this will better performance and efficiency.
Artificial intelligence and machine learning have been growing in the past decades and they have brought about technological advances in several disciplines. These advances coupled with our ability to store ever-growing amounts of data and the increases in computing power has led us to the brink of transformation in our society. A transformation whose scale and impact on our lives resembles the cusp of the industrial revolution. The aggregate digital data produced on a daily basis together with the computing powers that are available today make it possible to gain insights and draw conclusions for future decisions that were unthinkable to preceding generations.
These possibilities have already induced transformations in several areas and are now starting to transform our financial system. But hand in hand with the far-reaching possibilities comes an array of challenges of how to smoothly incorporate these transformative technologies into a running machinery of financial transactions and routines connected to it.
The challenges here are not fundamentally different from the ones that other areas of AI applications are facing. Yet they have their unique features which require bespoke solutions and careful fine-tuning to guard the stability of the financial system as a whole and the ethical but efficient use of data within this system.
The magnitude of data that needs to be processed, the speed at which decisions need to be made, but also the degree of possible ramifications that errors can cause bring about a whole array of complex responsibilities, for example in the case of malfunctions there’s a need for reliable prevention mechanisms.
In a presentation Deep Learning Volatility and a masterclass on Deep Learning Rough Volatility at QuantMinds International, we share our insights and experiences in the challenge of calibration with machine learning inspired by the corresponding article.
The real challenge here is not only to build some neural network that does the calibration job for us, but to design a powerful network architecture that handles all possible issues that might arise. We discuss in this presentation 10 challenges that we faced in this context and propose solutions to them that make the network work better. In the meantime, we continue to search for further challenges.
In particular, we present and discuss a powerful neural network-based calibration method for a number of volatility models, including the rough volatility family, that perform the calibration task within a few milliseconds for the full implied volatility surface. The conservative aim of neural networks in this work is an off-line approximation of complex pricing functions, which are too difficult to represent or too time-consuming to evaluate by other means.
Nevertheless, this perspective opens new horizons for quantitative modelling. The calibration bottleneck posed by a slow pricing of derivative contracts is lifted. This brings several model families (such as rough volatility models) within the scope of applicability in industry practice. We highlight several challenges in this context, in particular that the form, in which information from available data is extracted and stored, is crucial for network performance. With this in mind we discuss how our approach addresses the usual challenges of machine learning solutions in a financial context (availability of training data, interpretability of results for regulators, control over generalisation errors). We present specific architectures for price approximation and calibration and optimise these with respect to different objectives regarding accuracy, speed and robustness.