Building up accountability in algorithmic credit scoring
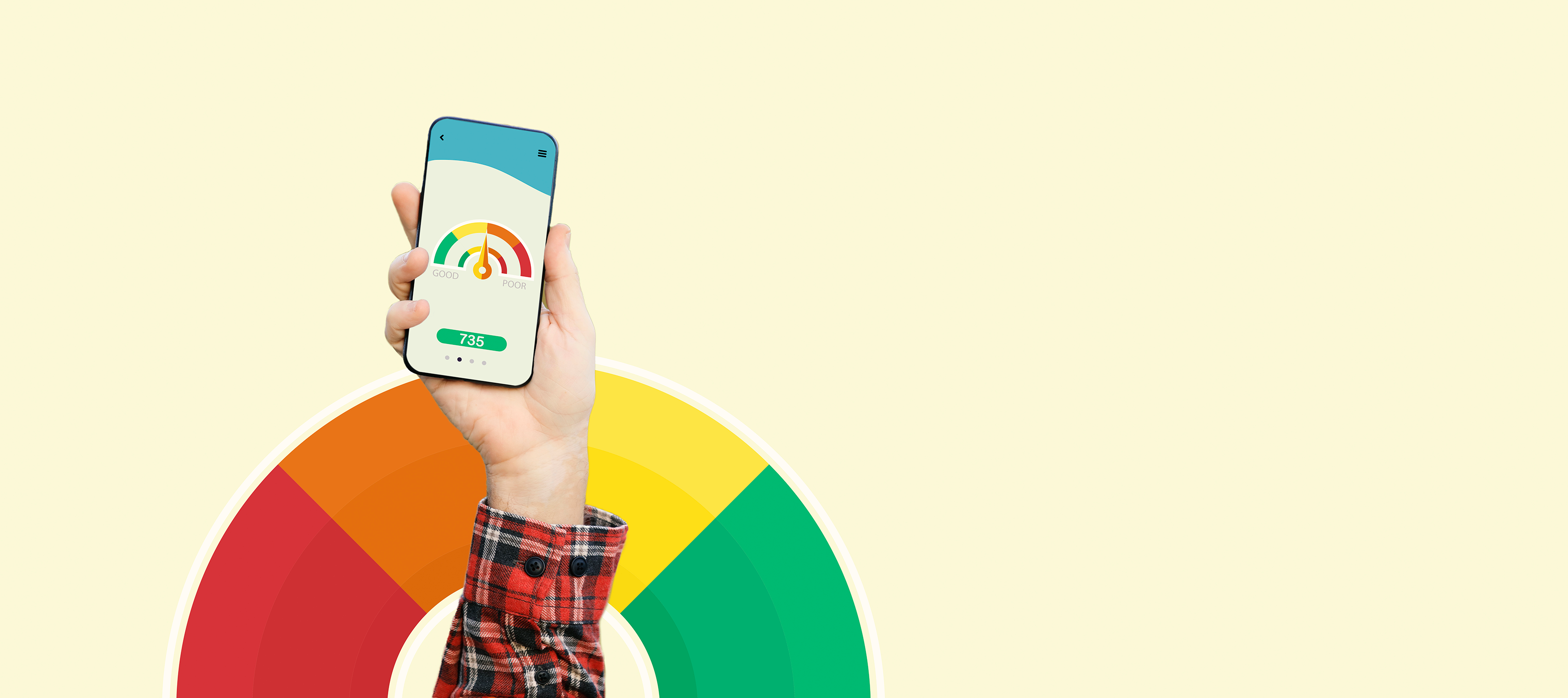
The central argument defended in this article is that the construction of the concept of accountability of the credit rating decision maker assisted by algorithms responds to the morphogenic[1] nature of regulation. We adopt the construction of morphogenesis led by Professor Margaret Archer in the sense of describing change processes conceived as "generative mechanisms"[2] that produce tendencies towards change in the relational organization of the social order. In our context, the social order comprises designers, users, and affected by algorithmic credit rating systems. The necessary interdependence between social normativism, social integration and regulation is at the center of this discussion[3]. We argue that the creation of an effective regulatory concept of accountability for the algorithmic decision maker, is called to respond to the regulatory objectives of distributive justice and reconfiguration of the right to privacy of the financial consumer. Consequently, this concept must find the balance between legal validity and solidarity and social effectiveness.
Morphogenesis processes in the provision of services and the offer of financial products generate the need to identify their regulatory challenges. One of these processes is specified in the evolution of the algorithmic credit rating. This is understood as a mechanism to assist the process of qualifying the payment capacity of consumers-debtors, through the use of machine-learning algorithms. As with other forms of algorithm implementation, algorithmic credit scoring allows you to analyze data, classify people into categories, and serve as a gatekeeper for some human needs.
While the main benefits derived from algorithmic credit scoring are anticipated to focus on increased efficiency and certainty in decision-making associated with granting loans, there are also limitations.
Thus, it is noted that the massive and rapid adoption of algorithmic credit rating systems can increase the probability of the occurrence of problems of inaccuracy, opacity and ways in which discrimination, already existing in traditional credit instruments, in granting is perpetuated. of loans to certain population groups. In addition, the potential high risk of affecting privacy, autonomy and the desired power of the financial consumer.
The occurrence of these and other new risks is closely related to the process of building regulation and the attitude of the recipients of regulation. As Archer studies it in the development of morphogenesis in the face of the crisis of normativity, a situation of social conflict or encounter of multiple diverse interests, is called to be solved based on the binding nature of normativity, which, in all case should avoid becoming a predatory regime[4]. This implies reaching a high level of legitimacy in regulatory decisions. This legitimacy implies involving the interests of all the subjects of the regulation, including the ‘conventional morality’[5] created in specific cultural systems. In this process of seeking agreement between normativity and legal order, we began by considering that there was a unitary and unifying normative source to which the legal validity was subscribed and, therefore, the obligations were binding on society. Then we evolved towards the concept according to which, increasingly, the legal order revolves around a minimum acceptance by regulated subjects, a view supported by Ross[6], Hart, Dworkin and Habermas. Consequently, we accept that in the regulatory decision-making process it is necessary to find foundations of compatible ideals[7], and thus achieve normative validity and social effectiveness.
The concept of accountability[8] refers to the set of burdens and responsibilities that arise in the decision-maker's head to explain to the affected or beneficiary with the decision – that is to the 'subject of the decision' – the reasons behind the design and the way that algorithm operates. The objective is that there exists, in a transversal way for all the scenarios in which algorithm-supported decision-making is being used, the possibility that the receiver or subject of the decision can accept or reject that decision. Naturally, the acceptance or rejection are called to be accompanied by a reasoned decision that is based on the foundations of compatible ideologies. Understanding that both the decision maker and the receiver of the decision are going to present reasoned arguments, there are several sciences that have tried to contribute with an answer. One of them is the political philosophy that formulates that based on the fact that most societies are founded on democratic principles, the argument that can help us find a justification for algorithmic responsibility is that of public reason[9].
This means to accept that institutions, principles, control mechanisms, and the imposition of sanctions, have to be justified in principles that we all agree upon. On the contrary, we would leave aside the individual criteria that have their own reason, but that will hardly find a common point that gives greater tranquility to this process of construction of the parameters to determine the elements of algorithmic responsibility. Our argument focuses on the fact that a way to find the balance between the different reasonings that concur in the establishment of the algorithmic responsibility in the particular case of the credit rating must be guided by the objectives of distributive justice and the reconfiguration of the right to privacy of financial consumers.
The development of technical concepts such as the need to explain the operation of the algorithm or "explanability" are at the center of the discussion. The European Union regulation contained in the GDPR[10] made it mandatory that intelligent autonomous decision-making systems can be audited and verified[11]. This implies understanding that the desired and widely discussed call for total transparency of algorithms is opposed by technical and legal obstacles associated with their protection and secrecy[12]. Both technical and legal discussion stems from the regulatory decision[13] to impose the burden of explaining[14] the logic behind the development of algorithms involved in decision-making[15]. Sensitive questions arise such as what is the type of information that should be revealed to the subject of the decision, or how a decision made with the assistance of algorithms can be explained[16]. Likewise, how to control biases or biases in algorithmic decision-making, especially when ethnic, racial[17], and economic aspects are involved. In establishing transparency as a desirable criterion in the design and use of machine learning algorithms, it is appropriate to recognize the need to define it. That is, to understand that the main consequence of the use of these tools is their decision prediction function, which can technically be divided into segments. That of data collection and aggregation of data sets, data analysis, and actual strategies and practices for the use of predictive models[18]. So then, each of these segments may require a different level of transparency. In the data collection and aggregation segment, transparency refers to providing information about the types and forms of data and databases used in analysis[19]. In the data analysis process, transparency is related to the technology used, this is to make known the names of the software applications when they are for commercial use, or if they are specially designed, the publication of the source code for those programs[20]. Finally, transparency in the segment of use of predictive models would seek to know the predictions made by models formulated through the data mining process[21], which lead to the construction of categories and profiles of the subjects of the decisions.
The complexity associated with the creation and implementation of transparency parameters in those three segments, which are required of designers and users of machine learning algorithms, explains the absence of regulatory intervention. Market participants end up making the data collection, choice, and analysis methodology decisions that best meet their business interests.
Requiring the regulator to establish transparency parameters would impose the duty of constructing sets of data whose use it authorizes[22]. In this hypothetical scenario, the burden of proving the causality between the information collected and the use that should be made of it, would fall on the regulator.
Additionally, understanding that a large part of the responsibility to protect the consumer of credit products corresponds to the financial regulator, the defense of transparency is recognized as a regulatory tool aimed at counteracting information asymmetry as a market failure. To illustrate our argument, we understand that information asymmetry is common to relationships between providers and consumers of goods and services. Market participants are obliged to present the necessary information to ensure that consumers make the right decisions when they are choosing or making use of these goods or services[23]. Financial regulation can be used as a mechanism that seeks to defend the interests of consumers, when it comes to the products offered by the financial sector and that have an impact on the administration of public resources. So then, as we will develop later, the construction of algorithmic responsibility must respond to the regulatory objectives of distributive justice and reconfiguration of the right to privacy of financial consumers. Faced with these two regulatory objectives, the financial regulator is forced to consider an expansion of the consumer-debtor's right-to-information duty, focused on achieving greater efficiency in the algorithmic credit rating.
Against this background, we explore one of the questions that has occupied those who design algorithms, those who are interested in using them and consumers as subjects of the decision made with the assistance of algorithms, that is, is it possible to adopt and develop a notion of 'accountability' or 'algorithmic responsibility' and how to do it. We develop our argument in three parts. The first one refers to foundational aspects of algorithm-based decision making. In the second part, we address the challenges of algorithmic credit rating, to consider both those arising from the current state of technology, and those arising from credit rating itself. Then, we provide the reader with the elements to build the notion of accountability or algorithmic responsibility.
The construction of algorithmic responsibility or accountability in the algorithmic credit rating must be based on the establishment of broad and clear criteria on the way in which the protection of privacy is observed in the terms explained and the search for compatible ideologies that give it content to distributive justice in its two manifestations, both equitable distribution of available resources and increased efficiency in making credit granting decisions. In the process of building these criteria, the financial regulator must attend to the possibilities provided by the algorithm model based on machine learning that is adopted and that guarantees a balance between predictability, stability, and interpretability. The achievement of this balance will be reflected in a decrease in algorithmic opacity and correlative epistemic opacity, in addition to the promotion of higher levels of trust by users and subjects affected by algorithmic-assisted credit rating decisions. Increasing transparency is a changing process that will reflect the expected morphogenic character of regulation. In this sense, the formulation of regulatory policies is called to have instruments of constant cooperation with the regulated, open dialogue that allows the assessment of the regulated interests at stake, and enforcement mechanisms aimed at strengthening voluntary compliance by the regulated.
The formulation of the notion of algorithmic responsibility or accountability should focus on facing both the challenges imposed by the state of the art of technology, as well as those inherited from the credit rating. In both scenarios a fundamental element is that of trust. This trust is based on the transparency with which access to information on the data collection process is guaranteed, the criteria used to determine which data is relevant for the study of consumers' ability to pay, the way in which it is analyzes that data, and the parameters that arise as a consequence of algorithm training, either in its causality or correlation function. It is then about aspects that are called to be recognized as part of the right-duty of information that assists the financial consumer.
Likewise, considering that financial strengthening and development lies in an improvement in social indicators and in the well-being of the population in general, the accountability regime that accompanies the algorithmic credit rating should directly reflect mechanisms of poverty reduction, improvement in income distribution, and guaranteeing that those who have access to resources will not be left in a situation of greater vulnerability than they were before receiving the loans.
Thus, in addition to the theoretical constructions on the ideal of justice, the design of the algorithms, the control and access to data can be decisive to ensure the observance of the shared ideology of distributive justice. According to which, it is not only sought to prevent the existing discrimination in access to credit from being perpetuated, but the discrimination factors that are revealed in the data linked to the algorithm are directly administered, to avoid affecting the rights of the consumers. In addition, there is a reevaluation of the position of the subject affected by the algorithmic credit rating decision, so that it is not simply the object that can be ranked, but rather becomes an active subject of the setting of standards of algorithmic responsibility or accountability.
The reconfiguration of the financial consumer's right to privacy is the second regulatory objective that guides the establishment of the notion of algorithmic liability. The realization of the right to privacy as a manifestation of the autonomy and self-determination of the financial consumer can make use of the financial education structure. Our proposal is that based on public policy instruments, such as the Principles for Digital Financial Inclusion, clear concepts are established that are delivered in the financial consumer's own language, allowing them to be aware that all virtual activity remains registered. That in the construction of their digital history it is possible for the consumer to build an identity and, in the exercise of their self-determination, decide what type of data will be available, when and who will have access. In addition, to be able to understand and interpret the way in which your data influences the algorithmic credit rating processes.
References
- See Buckley, Walter. (1967). Sociology and modern systems theory . Englewood Cliffs: Prentice-Hall
- Margaret Archer (ed), Generative Mechanisms Transforming Social Order (Springer International Publishing,2015) P vi.
- Margaret S. Archer (ed), Morphogenesis and the Crisis of Normativity (Springer International Publishing, 2016) P.2.
- Margaret Archer, Morphogenesis and the Crisis of Normativity (Springer International Publishing, 2016) P 6.
- Ibid.
- Ross, A. Directives and norms. London: Routledge and Kegan Paul. (1968).
- Koller, P. ‘On the nature of norms’, Ratio Juris, 27(2), 155–175. (2014).
- Bovens, M., Goodin, R. E., & Schillemans, T. (2014). The Oxford handbook of public accountability. Oxford: OUP Oxford.
- Reuben Binns, ‘Algorithmic Accountability and Public Reason’ Philos. Technol. (2018) 31:543–556.
- Regulation (EU) 2016/679 on the protection of natural persons with regard to the processing of personal data and on the free movement of such data, and repealing Directive 95/46/EC (General Data Protection Regulation) [2016] OJ L119/1.
- Bryce Goodman and Seth Flaxman, European Union regulations on algorithmic decision-making and a “right to explanation” https://arxiv.org/pdf/1606.08813.pdf
- Brkana, Maja and Bonnetaa, Gregory, ‘Legal and Technical Feasibility of the GDPR's Quest for Explanation of Algorithmic Decision: Of Black Boxes, White Boxes and Fata Morganas’, 11 Eur. J. Risk Reg. (March 2020) P1.
- GDPR Articles 13(2)(f) and 14 (2)(g).
- Article 22: Automated individual decision-making, including profiling, paragraph 1 prohibits any “decision based solely on automated processing, including profiling” which “significantly affects” a data subject. Paragraph 2 specifies that exceptions can be made “if necessary for entering into, or performance of, a contract”.
- M Brkan, “Do algorithms rule the world? Algorithmic decision-making and data protection in the framework of the GDPR and beyond” (2019) 27 International Journal of Law and Information Technology 91, 112, 118. Véase S Wachter et al, “Counterfactual explanations without opening the black-box: automated decisions and the GDPR” (2018) 31 Harvard Journal of Law and Technology 841, P 843, 863.
- M Brkan, “Do algorithms rule the world? Algorithmic decision-making and data protection in the framework of the GDPR and beyond” (2019) 27 International Journal of Law and Information Technology 91, 112, 118. Ibid
- Ari Schlesinger, Kenton P. O’Hara, and Alex S. Taylor, ‘Let’s Talk About Race: Identity, Chatbots, and AI’ CHI 2018, April 21–26, 2018, Montréal, QC, Canada.
- Tal Z. Zarsky, Transparent Predictions, University of Illinois Law Review, Vol. 2013, No. 4, 2013 https://www.illinoislawreview.org/wp-content/ilr-content/articles/2013/4/Zarsky.pdf
- Ibid. P.1524.
- Ibid. P 1525.
- Ibid. P 1526.
- Ibid. P 1528.
- Kotza, Mitchell and Balakrishnan, Srinivasan, “Information asymmetry, adverse selection and joint ventures: Theory and evidence”, Journal of Economic Behavior & Organization, 1993, Vol.20, N1, P.99-117.