Computational intelligence: a principled approach for the era of data exploration
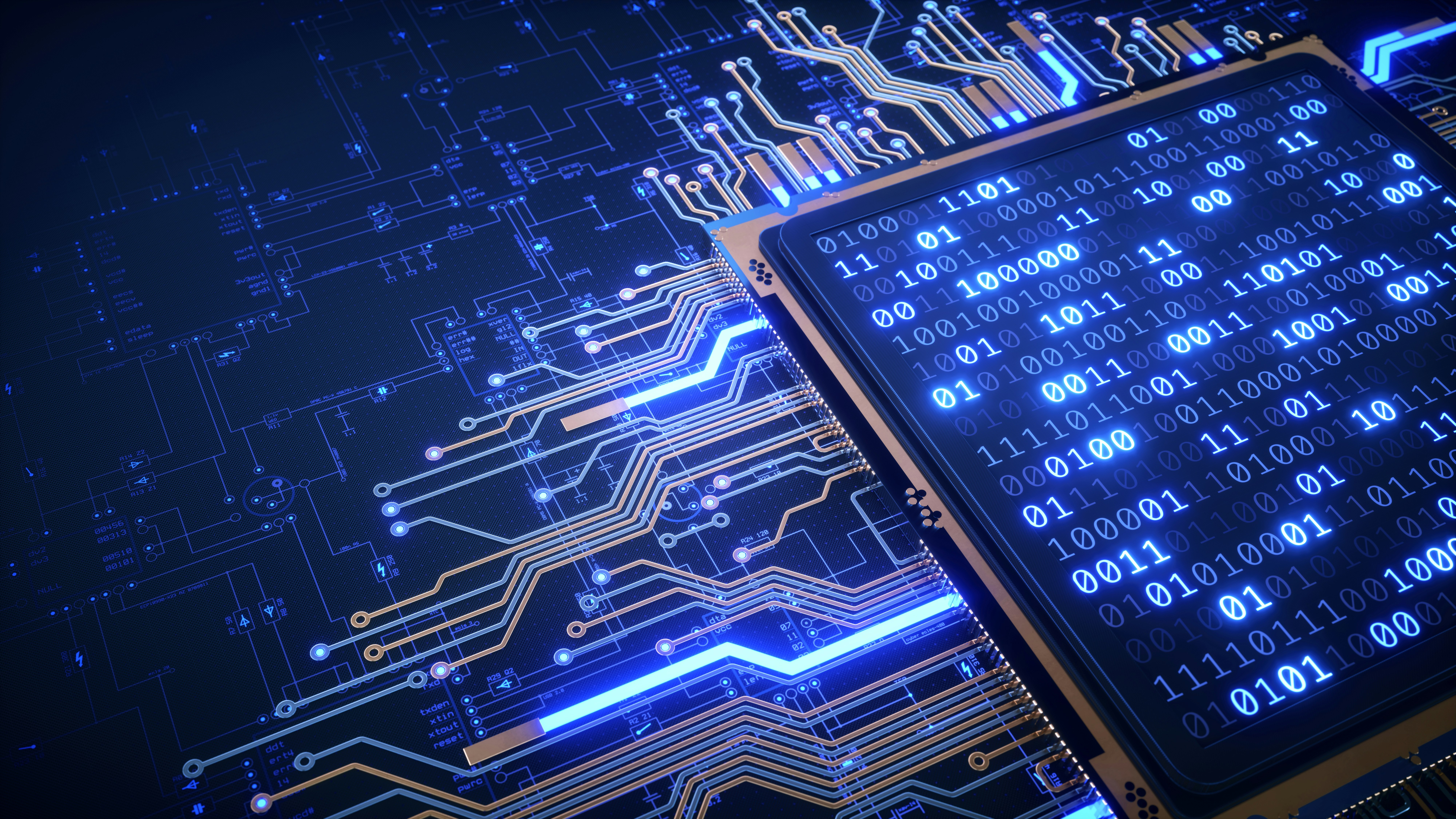
The term “AI” is frequently used as an intellectual wildcard and often without much scientific rigor. This is the manifestation of a systemic failure to understand the technical complexity of intelligence. Computational intelligence represents the currently available toolset to extract actionable intelligence from data, and as such it offers the promise of building the bridge between the awesome mystery of human intelligence and the aspirational world of AI. In this article, Cris Doloc, Founder and Principal of FintelligeX, introduces the concept of computational intelligence and its applicability to quantitative finance.
Ground-breaking technological achievements have created the conditions for a major paradigm shift in our culture. This is the dawn of a new era where business decisions are going to be driven by data and powered by algorithms. The development of a large variety of analytic models and computational tools has made it possible to find solutions to problems that were previously considered difficult or impossible to solve. Many of these computational methods are grouped under the umbrella of “computational intelligence” that is a paradigm addressing the ability of algorithms to learn through “computational means” a specific task from experimental observations by using data.
What is computational intelligence?
The specificity of computational intelligence (CI) lies in the fact that it represents a set of “nature-inspired” computational techniques that are applicable to complex real-world problems for which traditional mathematical modelling does not work, either because they are too complex mathematically, or because they are stochastic in nature. The methods used by CI attempt to mimic the human way of reasoning, i.e. using inexact and incomplete information, with the declared scope of generating controlled and adaptive actions.
This methodology is closely related to the field of “soft” computing which is a computing paradigm that will “exploit the tolerance for imprecision, uncertainty and partial truth to achieve tractability, robustness, low solution cost and better rapport with reality”. CI provides for the ability to learn and deal with new situations in which a system is required to possess reasoning attributes such as generalisation, discovery, association, and abstraction.
CI is closely related to biological concepts such as adaptation and evolution. These characteristics could facilitate the appropriate actions necessary for systems to “survive” in complex and changing environments. Biological organisms deal with such problems every day: extracting meaning from information that was acquired through perception, communicating and understanding via the use of languages, solving all kinds of ill-defined problems.
CI comports a multi-disciplinary framework that includes well established research fields such as artificial neural networks, fuzzy logic, evolutionary computing, or swarm intelligence. CI deals with “low-level cognitive” functions such as perception (i.e. object recognition and signal analysis), pattern discovery, object association and system control. The CI toolset is currently used in augmenting human performance (through automation) and in facilitating the extraction of intelligence from data. These techniques could be successfully applied to the new paradigm of data-driven trading (C. Doloc: “Applications of Computational Intelligence in Data-Driven Trading”, Wiley, 2019).
Computational intelligence in quantitative finance
One of the realities of quantitative finance is that many of its problems do not have exact solutions but require trade-offs and optimisations instead. A typical example is the optimal execution problem. From a high-level decision-making perspective, for every order there is an optimal execution schedule. But reaching the optimal rate of execution depends very much on preferences and tolerances assigned by market participants. These parameters depend on market impact and appetite for risk. During the process of learning and adaptation to the state of the market, a CI agent has to deal with a system of variables of high dimensionality and this will require a low-level decision-making capability. Every observed market state can potentially evolve into an almost infinite number of other market states. In such an environment the set of possible actionable parameters (i.e. order time, price, or size) is very large and dense. Moreover, local optimality does not necessarily translate into a global optimality; what could be considered as a bad decision (trade) now could eventually turn out to be an excellent one later. This is referred to as “non-local optimality”. The usual machine learning techniques are not going to be efficient for this kind of problem, and CI techniques could come to the rescue.
The novelty that CI brings to the field of quantitative finance is the centrality of the learning process. Dealing with the ever-increasing complexity of the financial markets will require a fresh new approach to cope with real world situation for which “hard” computing techniques are not effective any longer. The ability to adapt dynamically to rapidly changing environments, such as the financial markets, require the use of different methodologies, more akin to the principle of “soft” computing. A new breed of algorithms needs to be developed. This new family of algorithms will not run on environments already known to the designer, but instead they will need to learn from the environment they run on and cope with unknown scenarios.
Because of its emphasis on solving problems, CI should become an important tool in the decision-making process, by generating scenarios that are presenting the users with choices and their possible consequences. A long-term goal for CI is to create cognitive systems that could compete with humans in a variety of areas. Nowadays this is possible only in restricted domains, such as pattern recognition, automation, or reasoning in board games.
Despite a great deal of progress achieved by CI research, systems designed to solve lower level cognitive functions are still far behind the natural ones. The situation is even worse when higher-level cognitive functions (AI) like language understanding, reasoning, or problem solving are considered. The crude reality is that AI is a far cry from what the meaning of intelligence will infer.
Moving from the current paradigm of “representation learning” (or learning by “imitation” from data) to a paradigm of “machine consciousness”, where the agent will be able to make decisions based on independent “thinking” by using an approach that is more akin to human behaviour, is still a distant milestone.
Until more progress will be achieved by cognitive sciences in the understanding of how human intelligence works and how it could be optimised, the financial industry will have to focus its efforts on the development of computationally intelligent methods (C. Doloc: “Applications of Computational Intelligence in Data-Driven Trading”, Wiley, 2019) that will improve the automation of actionable intelligence extraction from data.