Correlations in modelling energy derivatives
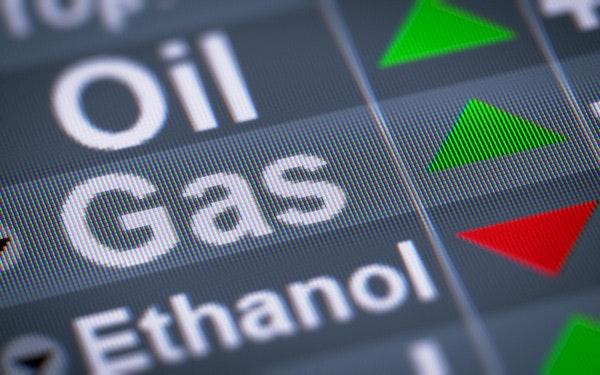
Because of illiquidity, in many cases, correlation inputs are based on historical observations. Even though their estimates might be unstable, realised correlations between two commodity futures follow two main trends of growth and decay. NYU Professor Roza Galeeva proposes a simple model, reflecting empirical properties of commodity correlations and their observed dynamics.
The modelling of the joint behavior of various prices is crucial for the proper evaluation and hedging of energy derivatives. One measure of dependence between prices, among others, is linear correlation. Despite its known limitations and drawbacks, linear correlation remains the most popular tool in financial markets, including energy markets.
Correlation is a key input into the pricing of spread option, which are options on a spread. Among all commodity derivatives, it is the most important option. Everything in energy markets is about spread options. Examples include evaluation of power plants, involving spread between power and fuels (spark spread); storage modelling, which use calendar spreads; pipelines, transmission and many other. While correlation is an important input to a spread option, it is a non-transparent input, as usually there are no liquid markets in correlation. This presents a challenge in their modelling. Another difficulty is a known phenomenon of instability of correlations in financial markets, which could manifest itself even in a more extreme way in energy markets.
For example, correlations between spot power prices and the corresponding spot fuel prices exhibit strong instabilities. While analysing correlations, one should bear in mind that it cannot be done separately from analysing volatility.
Another examples of energy derivatives, which depend on correlations, include baskets and swaptions, which are options on a swap. A typical commodity swap contains several futures, normally over one or several calendar years.
The evaluation of a swaption requires knowledge of a whole calendar correlation matrix between different futures in the swap.
There are many types of correlations in energy: correlations between different assets, (power and gas), correlations between different futures on the same asset (calendar correlations); correlations between different trading blocks, as peak off peak for power, daily correlations and many other. Evaluation of a typical power plant with life span of five years, can easily involve correlation matrices of size 360 and more.
Because of illiquidity, in many cases, correlation inputs are based on historical observations, using calculated realised correlations. Even though their estimates might be unstable, realised correlations between two commodity futures follow two main trends: on the one hand, longer maturity contracts tend to move in more accordance, so they exhibit stronger correlations, and on the other hand, correlations decay with distance between contracts.
To be more specific with respect to the first property, we consider historical correlations between two commodity futures with expirations, Ti < Tj calculated on an observation period [t1, t2]. Then correlations increase with time to the first contract Ti-t1, as well as with the length of the observation period t2-t1. This resembles the well-known Samuelson effect of increase of volatility of any future commodity contract Ti as time t gets closer to the future expiration. The direction of the growth is inverse to correlations, so as a contract, whose expiry is far away, is less volatile. A rough explanation of this fact is that bigger swings in futures prices closer to their expirations cause disconnect in their joint movements, therefore, weaker correlations. The way to achieve this volatility behavior is done by modelling the instantaneous volatility σ(t, Ti) of a future contract, typically, as a function of time to the expiration, Ti-t.
In our research, we start with the case of calendar correlations for the same commodity, as oil, natural gas and Eurodollar.
To accomplish the above property of realised commodity correlations, we propose a dynamics of instantaneous correlations ρ(t, Ti, Tj), together with a simple choice of Samuelson parameterisation for volatility. This allows us to effectively map realised correlations, similarly to mapping market implied volatilities of commodities futures to earlier expiration (as in the case of a swaption).
We will give further details of our research in a forthcoming story, and of course at QuantMinds International.