Fairness in AI and machine learning
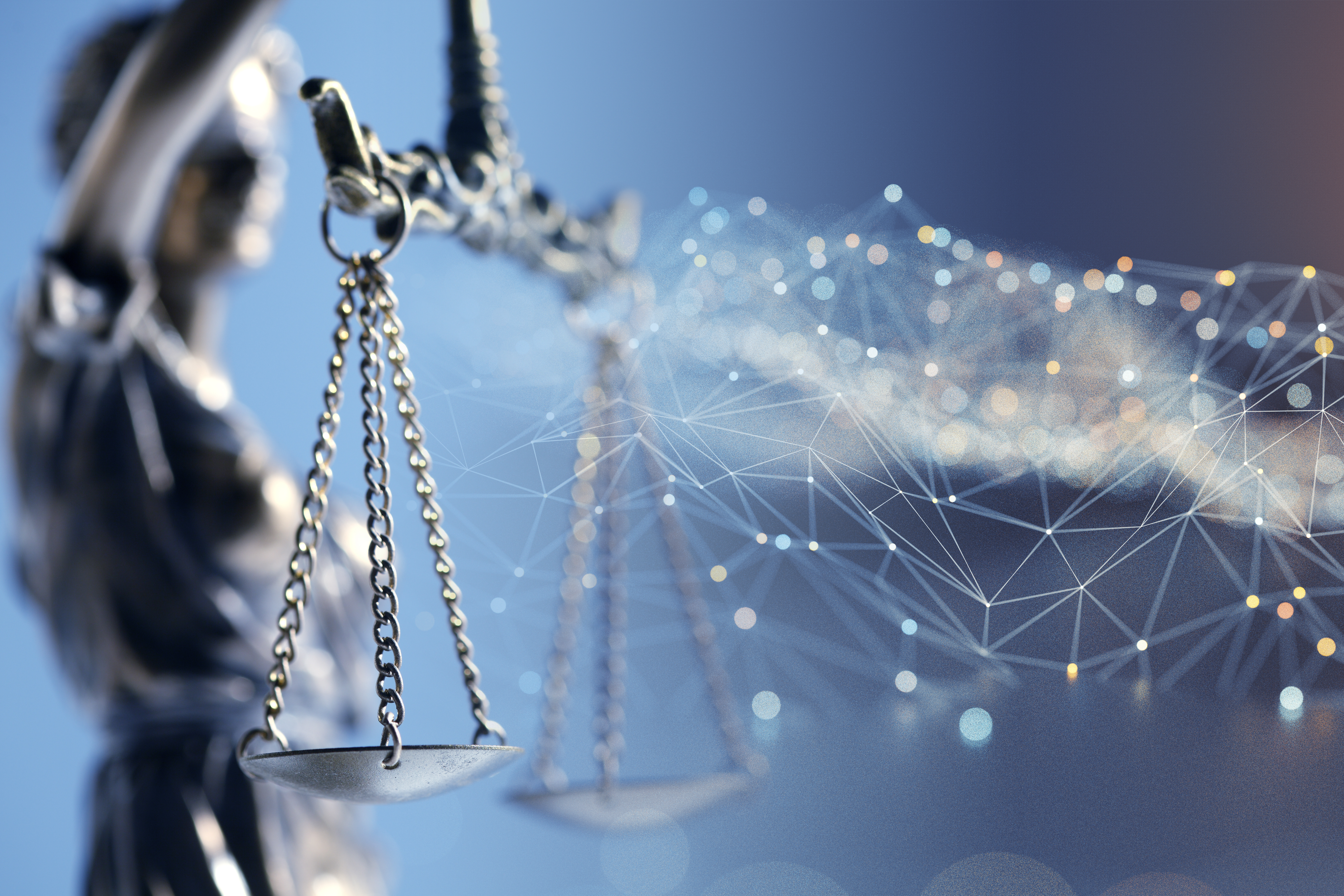
Artificial intelligence (AI), powered by machine learning algorithms, is finding its way into the financial system. AI is currently applied in financial institutions in immaterial areas such as marketing (product recommendation) or customer service (chat bots). However, slowly but surely AI is gaining ground in high-stakes decision making such as credit issuance, insurance underwriting, and identification of fraud and money laundering. These applications are potentially very sensitive to unfair treatment of different groups or individuals. Such an unfair, i.e., biased treatment is particularly damaging for finance sector, as it is held in higher societal standards than other industries, because it is fundamentally based on trust.
Machine learning algorithms learn patterns from historical data, which they then carry forward. So any potential bias reflected in that past data will be reflected – and possibly amplified – in the outcomes of a ML algorithm. One famous example is the Apple/Goldman Sachs credit card, which was unveiled to a great fanfare at the end of 2019. Apple proudly declared that the credit limit on their card will be determined solely by an AI algorithm, only to find out, a few days later, that women got on average 10 times less credit than men, even in cases of the same or higher credit score. One can only imagine the reputational damage this has inflicted on Apple and especially on Goldman Sachs.
Avoiding such disparate treatment of certain groups of society – based on gender, race, or age – is at the heart of AI fairness. At the end of 2019, ECB came up with Ethics Guidelines for Trustworthy AI. Two out of six of these guidelines – Fairness and Ethics – are directly related to fair and unbiased application of AI.
Whether an AI or ML-aided algorithm is fair can – and should be – assessed before this algorithm is adopted in practice, and potential bias of an algorithm can be quantitatively measured. But for that, first we have to define the so-called protected attributes, i.e., those features of an individual on basis of which he or she should not be discriminated, e.g., denied credit or an insurance policy. Typical examples of protected attributes are race, gender, age, sexual orientation, or religion. Protected attributes are determined by law, but financial institutions can outline their own ethical standards (alongside those enforced by regulation) and ensure their AI algorithms comply with those standards as well.
The main risk resulting from AI algorithms being unfair is reputational risk, and it is particularly damaging for financial institutions. So ensuring fairness of your AI solutions should be one of the routine tasks of risk managers and all those responsible for implementing AI and ML in your organisation.
Next, I would like to give some practical insights into how the bias in ML algorithms can be measured and where in your modelling process it can be eliminated.
Measurement of the bias is the first important step: an algorithm can be “very” biased (think of the Apple/GS credit card example from my last column) or a “little bit” biased (and a small bias could be something you can live with). There are three formal definitions of the model’s fairness:
- independence,
- separation, and
- sufficiency.
Hence, three ways of measuring bias.
Independence is the crudest fairness definition and it strives for equal outcomes for favourable and less favourable groups. For example, this means that men and women should have the same overall chance of getting a credit. This definition is very simple and easy to understand. However, there could be a lot of heterogeneity between the groups of men and women, and so this definition can be too crude to appropriately address the bias.
A more subtle definition is that of separation. To explain this definition, imagine you have a sample of men and women in your dataset, for whom you know whether they defaulted on their credit in the past. You have built a ML model which predicts whether someone will or will not default (and your credit issuance decision will be based on this prediction), and you apply this model to those individuals. Separation means that, if you consider only those people who defaulted, the chance that your model also predicts them to default should be the same for men and for women. The same, by the way, should hold for those who did not default. In mathematical terms, this means that true and false positive prediction rates should be the same for men and for women.
The last definition is sufficiency. It’s quite similar to separation, only here, we swap what we predict and what really happened: consider only those people who we predicted to default. Among those, the proportion who really defaulted should be again the same for men and for women (and the same should hold for those who we predicted to default but who did not default in reality).
The magnitude of your model’s bias is the discrepancy between those probabilities (in either of the three definitions) that should be equal. Usually we use the so-called four-fifth rule: the difference between these probabilities should be not more than 20%, or we deem the model significantly biased.
Which of the three ways the bias is measured is usually left up to the model builder. Suppose the bias has been measured and it turns out to be too high. What now? Well, there are also three points in your model where it can be improved in that respect. First thing you can do is to modify the data used to train the ML model. This can be done via the so-called “massaging” (swapping some of the outcomes between the advantaged and disadvantaged groups), re-weighting or changing features to increase fairness. We call it bias mitigation in pre-processing stage. Another solution is to modify ML algorithm itself, so that it becomes less biased. This can be difficult and costly, as most model builders rely on ready-made algorithms which are not easy to change. Finally, you can also change the model outcomes to increase fairness – this mitigates bias in the post-processing stage.
Any bias mitigation algorithm would typically decrease the performance of the ML model. So the bias mitigation is a balancing act between improving fairness while not sacrificing too much of the performance. However, this balance is easily achieved: the modern bias mitigation techniques do not require too much of the performance loss, while significantly improving fairness of your ML model. This should be the joint task of quant modellers, model validators, and risk managers in financial institutions in order to prevent major reputational risk in this area.