Federated learning can aid clinical research says Rhino Health
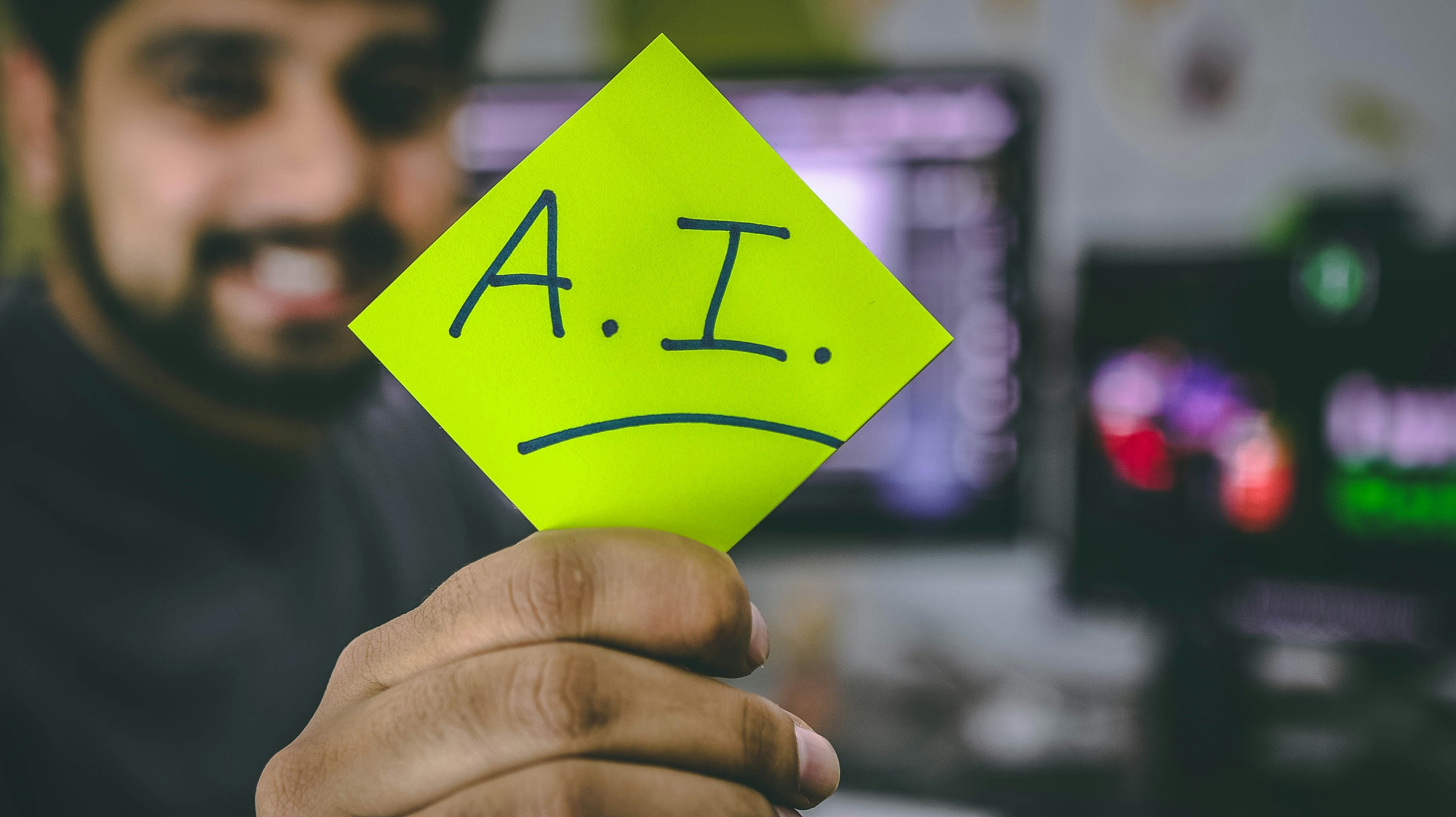
The AI technique federated learning can give trial sponsors deeper data insights, speed recruitment and improve remote monitoring according to Rhino Health CEO, Ittai Dayan.
In federated learning (FL) – like machine learning (ML) – predictive computer models are trained using data. The key difference is that learning data is sourced from multiple servers rather than from a centralized resource.
Use of distributed data has numerous potential benefits according to Dayan, who told us “The most obvious advantage of federated learning over traditional ML methods is that FL allows you to use a large data set without centralizing those data. This can help protect patient privacy and reduce the risk of data breaches.
“Additionally, because the data are processed where they live, there is less need for powerful centralized servers, which can reduce the cost of computing.”
Also, because the approach leaves data with source owners that are often more comfortable providing access, which can broaden the population available this “can be especially useful in a clinical trial setting, where data is often collected from multiple hospitals and clinics” Dayan said.
Frameworks
Industry use of federated learning to-date has been limited. Although various real world evidence platforms that can access and model data from multiple sources are available industry lacks the analytical infrastructure to fully utilize the information according to Dayan.
“These data or insights can be used in trial site selection, but don’t achieve the same value that deploying models in real time through FL would.
“These federated data sets provide a strong foundation for true federated learning, but CROs, sponsors, or the RWE companies still need further processing via a federated learning framework such as Nvidia’s NVFLARE.”
He added that “Rhino Health is actually working with some of these companies to prove out how they can add more value to their biopharma clients.”
Unsplash/hiteshchoudhary