Less is definitely more: a lesson in simple heuristics
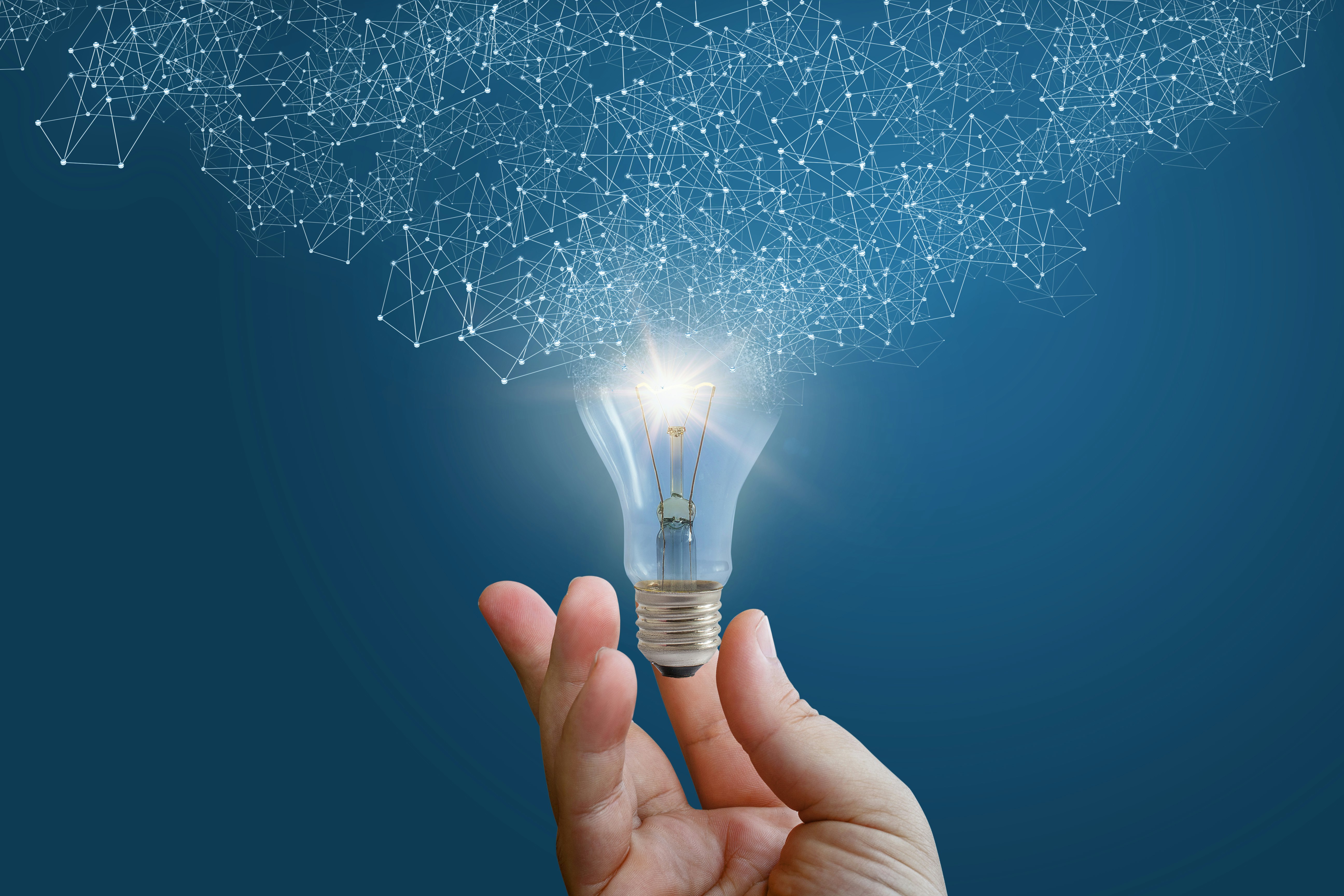
Being a successful quant in the modern age is more than just having a strong understanding of models and computational methods and statistics. Demand for soft skills and out-of-the-box thinking is on the rise, so QuantMinds International invited Gerd Gigerenzer to speak about simple heuristics which shed a new light on complex models.
It’s easy to assume that, now that we have access to masses of data and increasing computational power to analyse that data, we must use as much of it as we can get.
But that isn’t necessarily so, according to Gerd Gigerenzer, Director at the Max Planck Institute for Human Development and Harding Center for Risk Literacy in Berlin.
Gigerenzer made a convincing case at QuantMinds 2018 in Lisbon that there were situations where less is very definitely more.
How complex and how simple should models be? Is more data always better? Or can simple rules be not only more transparent and understandable, but more accurate?
These were some of the key questions posed - and answered - in the session.
When complex modelling doesn’t work
Machine Learning has been capable of extraordinary feats – take Google’s automatic facial recognition technology or the success of AlphaGo – but it has also experienced catastrophic failures.
Google’s Flu Trends was a dismal failure at predicting the spread of flu. Similarly, risk assessment algorithms, currently used in the US to predict the likely recidivism of a convict, were found to be no more accurate than an untrained human.
In both these cases, a simpler approach had superior results.
We live in a world with a certain degree of uncertainty, where we don’t know all the options, consequences of variables, and where the future is not like the past. In this world, trying to optimise and fine tune can lead to more error.
“In the case of flu, a very simple heuristic model that only looked at three variables worked much better,” explained Gigerenzer.
“It looked at the number of flu-related doctor visits two weeks previously, three weeks previously, and the number of doctor visits one week prior to the flu diagnosis one year before. This was more successful than all the complicated big data analysis.”
Similarly, a very simple rule could do better than the recidivism algorithm. “Just considering the age of the defendant and the number of previous convictions did much better than using 137 variables,” he said.
Ultimately, the heuristic model is based on what a human would do, as Gigerenzer explained:
“What we do is we look at experienced and successful people and try to work out how they solve problems. We then model and test this to design and model heuristics that are transparent for the user, and which often do better than highly complex models.”
Risk versus uncertainty
So the question is, how can we tell when simple is not just an easy approximation, but actually outperforms a complex algorithm?
To answer, Gigerenzer referenced the distinction made by Frank Knight between situations of risk and uncertainty.
“A situation of risk is one where we know the entire state space, we know all alternatives, all consequences and if there are probabilities, we know them for sure.
“In this world, fine-tuned optimisation works.
“But of course, this is not the world that we live in. We live in a world with a certain degree of uncertainty, where we don’t know all the options, consequences of variables, and where the future is not like the past. In this world, trying to optimise and fine tune can lead to more error.”
How should we make decisions in this world? The answer is simple heuristics, said Gigerenzer.
Not dropping the ball
“The successes of machine learning are typical in situations of games - like chess - where the future is always like the past.
“The failures are mostly in situations of uncertainty. Flu is not something you can really predict. In that case fine-tune methods will overfit and will incur more error.”
The gaze heuristic is an example of how simpler can be better.
Take the case of a baseball player catching a ball. An experienced player knows where to run to catch the ball. But how does he do it?
“There are two visions,” explained Gigerenzer.
“One is that it’s a complex problem that requires a complex algorithm to solve. The other is that it’s a problem of high uncertainty (variable wind and speed) - not risk - and in this situation you need to simplify.”
Studies show that players rely on a set of simple heuristics - the gaze heuristic. They simply fix their gaze on the ball, and maintain the angle of this gaze while running towards the ball.
“The point is the player can ignore all the variables in the equation in order to calculate the trajectory, and rely only on the single one - the angle of gaze.”
“By doing that, the player avoids not only the computational problem, but the estimation one, and therefore incurs less error than if you would do all these calculations.”
The bias–variance tradeoff
Gigerenzer gave another example of how heuristics had beaten more complex models in finance.
“The commonly-understood solution if you want to invest money in a diverse way is to build a portfolio using Markowitz mean-variance.
“But research has shown that simple heuristics could also be used, by allocating money equally to the number of options available – 1/N. For instance, if N = two you invest 50% in each.
If you have high uncertainty, many alternatives, and a small amount of data then you can expect to do better with simple solutions.
In Gigerenzer’s research, 1/N made more money in 6 out 7 tests than the Markowitz optimisation method, and he explained why:
“The Markowitz model would be optimal if all estimates are precise and the model right.
“But that’s not the case in the real world of finance.”
“In a situation where you have low uncertainty, few alternatives, and large amount of data - in that world you are likely to win if you make it complex.
“On the other side, if you have high uncertainty, many alternatives, and a small amount of data then you can expect to do better with simple solutions.
“1/N has only bias, no variance, and that’s the secret why it does better.”
The triumph of a subset of data
“By definition heuristic simplifies, it doesn’t estimate any parameters,” concluded Gigerenzer.
“In situations where we have degrees of uncertainty, to scale down is to minimise prediction error to avoid overfitting.
“There is a misnomer that simple heuristics are only good because they allow you to make decisions with less effort, but the argument is much stronger than that.
“Less is more - you can do better with a subset of that information.”