Model robustification and the future of quant finance: an interview with J.D. Opdyke, Allstate
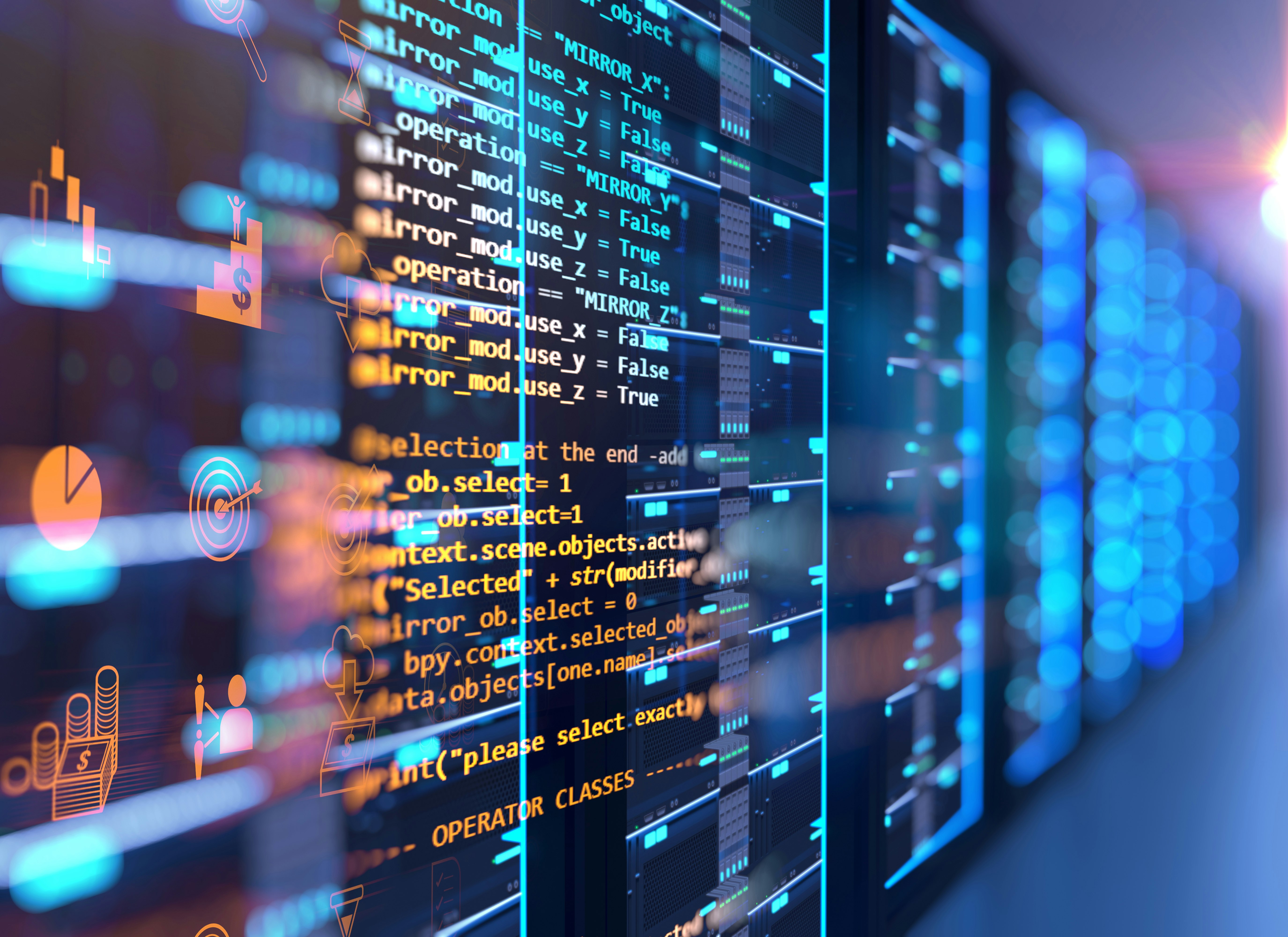
Watch the interview with J.D. Opdyke, Vice President, Enterprise Risk and Return Management, Financial Risk and Measurement, Allstate, or read the written version below.
Thank you for joining for this quick interview. How have you been?
My pleasure! I’ve been great; all has been working out well.
What have you been up to these last couple of months? Did you pick up any new hobbies?
I haven’t picked up anything new. Things have been extremely busy, but like I said, things have worked out well in 2020 with Covid-19 and going remote. We’ve been very fortunate.
I’m the head of enterprise risk analytics at Allstate. For our industry – insurance –, for our firm – Allstate –, and also for my group – the enterprise risk analytics group –, things have gone very smoothly during 2020. We’ve gone fully remote, and much to my firm’s credit, the ease with which we’ve done that has been very impressive to me. Part of that has been due to the nature of our work, essentially as a model development team. The data usually comes to us anyway, so we’re good as long as we have the computational horsepower we need to do our jobs. It’s really been a seamless and easy transition to go fully remote for us.
That’s really good! The exact opposite of some companies. I’m guessing not a lot of things have changed, but was there anything in particular that you feel you’ve learnt from this? Was there anything that was a challenge these past couple of months?
From my perspective, running a fairly large team in model development at a large financial institution, I think the major lesson learnt was what model development should have been doing all along, which is:
Do not wait until you’re in the middle of a pandemic and an economic upheaval to make sure your models are robust.
This is easy to say, but it does speak to the issue of building this robustification process into your model development processes so you’re not caught with your pants down when a pandemic or an economic upheaval happens.
And that’s a lot easier said than done, because under normal times, people compete for resources; there are a lot of demands. No matter what your capacity, people are always going to fill that with demands on the group. You have to have the processes in place to make sure that as you’re developing your models, they’ll be robust. So when the pandemic comes, you’re not starting from scratch, you’re not panicking, and you’re not developing new models when you don’t have time to develop new models.
Obviously, no matter how much robustification you do, you’re going to be running new scenarios. There are going to be fire drills. But if you’ve done model development right, you’re going to have robust processes in place and robust models in place when those things hit, so you can weather the storm. Even if you’re not doing scenario analysis as part of the deliverable for which you’re developing a range of models, do scenario analysis. Kick the tires on your models. Whatever the transparently defined range of parameter values and inputs for the project or model are, make them wider. Do the scenario analysis because you’ll be ahead of the game, even if you don’t end up in a pandemic, you’ll end up with models that are more robust. That’s never a bad thing.
So I have to say, if there’s one lesson from the past year, it’s really what we already knew and what you have to do to stay ahead of the game in model development in quant finance:
Build the processes in place so that while you’re developing your models, you’re sure they’re robust to a wide range of scenarios.
What sort of things are you looking out for when you’re building a robust model?
Whether you’re doing enterprise risk or putting together and estimating risk for investment portfolios, what I’m describing is required for just about any estimation of portfolio risk regardless of the level of aggregation or the industry.
Obviously, robustification is a very broad topic. My specific deck (at QuantMinds Americas) addresses doing this with correlation matrices and estimating correlation matrices. Any model development doesn’t only rely on kicking the tires on the inputs. Also kick the tires on the ranges or parameter estimates that you’ve generated from your inputs. Historical data hasn’t existed long enough to represent all the scenarios that are going to occur in the (near) future that we want to test for and that we want to make inferences about.
That’s why I emphasise, in addition to robust methods and statistics, robustify your inputs. Robustify the ranges of parameter values that you think are relevant to your exercise. Make them wider and broader, if you want your inferences to be good for a longer period than two month into the future (this gets to the issue of multiplicity and overfitting your data). If you want the core of your models to be useful and usable for longer than just a minute, then you need to use robust analytical methods, robustify the inputs, and you need to kick the tires on the parameter ranges that you’re estimating in your model.
What’s the future of quant finance?
Looking into the future of quant finance, the way that I’d phrase that question to be most helpful to me (and to us) is to think of the things that will differentiate successful people, methods, funds, and firms. Identifying these things in my mind crystallises what I see in the future of quant finance because these are the things that are going to last. These are the things that are going to move our industry forward. For me, it’s three core things that are going to characterise any successful methods and approaches or people, funds, or firms.
My three points here link together in important and useful ways. I think if you’re checking these three boxes, it will be very hard for the person, the fund, or the methods that you’re using not to be successful going forward in quant finance.
However, these depend on this preface: data and computing power. No one will dispute that our data today is richer, more complex, and more plentiful than it ever has been; and our computational power is as well. Whether it has kept pace or exceeded is moot. They both increased orders of magnitude recently. These feed into the three characteristics I see as being key to successful quantitative finance endeavours in the future.
First is the flexibility and the ability to do cross-disciplinary research. In my view, the labels don’t matter, whether you’re doing multivariate statistical models, econometrics, machine learning, data science… Whatever you call them, the analytical rigour is what matters. Where you draw those lines really does not matter because in the end, if you are putting them in boxes, you’re going to miss things. Because data is so complex, rich, and plentiful, you have to take a flexible and cross-disciplinary approach to solving real-world problems. In a textbook, not so much. Textbooks are used to illustrate and make specific points (so it’s a little bit unfair to take shots at textbooks in that way), but for real world problems, you have to go cross-disciplinary. You have to have the flexibility and ability to do that. That means a lot of depth and breadth on any model development team.
The second, which I think is even more important and fundamental, is that we have to allow our theories to be informed by the data (and there are very good reasons for this). Historically, in academia, there was a linear progression from theory development to testing those theories using the data, but it wasn’t a two-way street by any interpretation. I think that paradigm is overly rigid and constraining, and we will learn so much from our data if we lengthen the leash on it.
That said, which leads me to my third point, we have to be hyper vigilant and very careful that we are not committing all sorts of cardinal sins when it comes to modelling. We cannot overfit our data; we cannot model to our samples, rather that the populations about which we want to make inferences; and we cannot forget about entire areas of statistics that have existed for decades. Many of my brethren in quant finance do sometimes tend to forget about multiple comparison issues – multiplicity –, and controlling family-wise error rates, false discovery rates, and false discovery on proportion rates. These are foundational, even if they have their origins in clinical trials and other industries. If you are developing factor models in quant finance, you are testing functional forms and assumptions thousands of times. You have to account for that when you’re assessing, not only the statistical significance, but also the materiality of your results. Just by chance, you’re going to find lots of results that appear to be statistically significant and meaningful, but are not.
I published a paper on multiple comparisons in 2003 but it’s just as relevant today as it was (and it wasn’t even new then). But it’s very essential to quant finance going forward, especially because we are loosening the paradigm between theoretical research and empirical research – as we should! So what I’m suggesting is that we do this very responsibly and we make sure that we’re not overfitting, and we’re not fitting to the sample. These are all complimentary things to robustness as we were discussing earlier.
In conclusion: cross disciplinary flexibility and ability, allowing our data to inform our theories – if not sometimes drive our theories –, and being hyper vigilant about overfitting issues and multiplicity. Again, this is an area of statistics that people in other analytics silos underappreciate. My three points here link together in important and useful ways. I think if you’re checking these three boxes, it will be very hard for the person, the fund, or the methods that you’re using not to be successful going forward in quant finance.