New frontiers in Big Data, Machine Learning and supercomputing
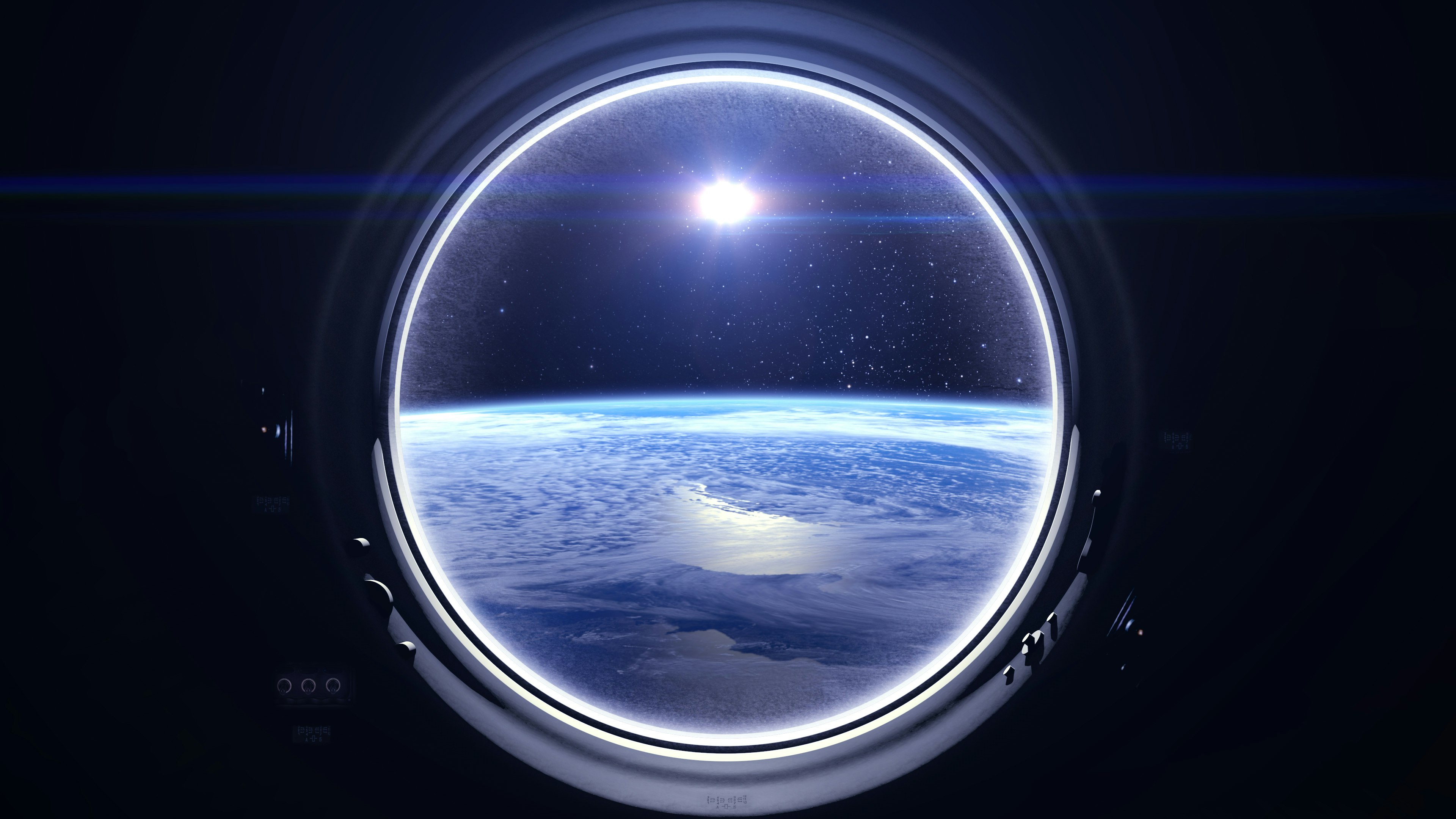
Big Data, Machine Learning and Supercomputing have become an indispensable part of scientific research, and are making waves in quant finance industry. At QuantMinds International this May, a panel of thought leaders in these fields will discuss scientific breakthroughs that would not have been possible without these technologies and how financial institutions could benefit greatly from the adoption of these tools and approaches. Here, QuantMinds asked the panellists to introduce some of the key ideas and highlight some key developments to watch out for.
The Panel:
Marcos Lopez de Prado, CEO, True Positive Technologies and Senior Lecturer, Cornell University (MLP)
Dr. Leo Razoumov, Principal Machine Learning Scientist in Amazon (LR)
David Leinweber, Founder Centre for Innovation Financial Technology at Lawrence Berkeley Laboratory and author (DL)
.
.
Horst Simon, Deputy Lab Director for Research at Lawrence Berkeley National Laboratory (HS)
.
.
Alejandro Perdomo-Ortiz, Senior Research Scientist at Rigetti
.
.
What are you currently working on?
MLP: I manage multi-billion dollar portfolios for institutional investors using machine learning techniques and supercomputing technologies. I have recently published a new book, Advances in Financial Machine Learning (Wiley, 2018), based on the on-boarding manual we give to new recruits, so that they hit the ground running at True Positive Tech.
LR: Currently, I am building Machine Learning (ML) and Deep Learning solutions to forecast demand for pretty much everything that Amazon sells. This is an example of probabilistic multiple time-series forecasting which is very common in the world of Quantitative Finance.
DL: I am now officially a retired FinTech codger. In the 80s, I was a founder of one of the first firms to use AI in a commercial trading system, MarketMind, which used expert system technology to analyze quantitative market data. In 1999, I founded a company called Codexa that used natural language and AI methods to extract investment information from textual web sources. After stints as low forms of faculty life (visitor, adjunct, fellow) at USC, Caltech and Berkeley, I was invited by Horst Simon to found the Center for Innovative Financial Technology at Lawrence Berkeley National Lab. Marcos was an early supporter. Small world.
APO: I am passionate about the computational limits and opportunities of quantum computing for problems in Artificial Intelligence (AI). At NASA’s Quantum AI Laboratory, I lead the Quantum Machine Learning effort, where we have been designing algorithms with the potential for speeding up and enhancing current machine learning pipelines. At Qubitera, LLC, we are excited to be working at the intersection of quantum computation, ML, and finance applications.
Why have big data, machine learning and supercomputing come to the forefront over the last year?
DL: I think this “coming to the forefront” is due to some impressive, easily understandable, and well publicized successes. Few people notice when a computer is used to prove a theorem in topology. Beat the reigning Jeopardy champ, and it makes the news. Throw in widely used speech recognizers (Alexa, Siri and the like) and put them on phones, and everyone notices. AI has been around, and had impressive, but narrowly reported successes, for decades.
While reporters like to focus on the millions of jobs that may be replaced by ML, they seem to ignore that the implementation of these technologies is non-trivial and it requires substantial investments in human capital.
LR: In the last couple of years, the usefulness of ML solutions was conclusively demonstrated in several important and diverse domains ranging from Speech Recognition and Natural Language Processing, to beating humans in board games like Go (Google Alpha Go). Late last year ML took a lead in game of Poker -- a non-deterministic game which is very different from types of Chess and Go. And impressively, training of the models was achieved by so-called "self-play", where the machine plays millions of games against itself. This relies on massive computational power. Most of the fundamental Deep Learning algorithms have been known since 80s and 90s of the last century. It is the massive advances in computational power and storage that made those old algorithms perform so well.
MLP: Recent breakthroughs have been achieved before most people expected them. Today, these technologies can accomplish tasks that until recently only expert humans could perform. High performance computing (HPC), Big Data (BD) and ML are ubiquitous today in our daily lives. Take air travel, where pilots make today a fraction of the decisions they would have made in the past. At airports, screening algorithms conduct background checks, looking for any suspicious pattern. For those who found their luggage, they have an algorithm to thank, and for those who didn’t find it, an algorithm will soon recover the suitcase that some human misplaced.
APO: Besides the significant progress in ML and AI, there are still tons of problems, of which even the largest supercomputer centers have a hard time in solving, and some of these will not be solved in the next decades. Still, quantum computing has come to the forefront as well as an alternative with the potential of revolutionizing the computer industry, with FinTech one of the core applications.
Do you think there are persisting misconceptions about these technologies?
MLP: Indeed. One of them is the belief that these technologies are off-the-shelf, or that they run by themselves. While reporters like to focus on the millions of jobs that may be replaced by ML, they seem to ignore that the implementation of these technologies is non-trivial and it requires substantial investments in human capital. Those who resist progress will be replaced, not by machines, but by those who embrace it and adapt. Another misconception is the belief that these technologies are somewhat in an early stage of development. As David said, the truth is, these technologies are mature and have demonstrated their power for many years in large-scale research programs. It is only now that the public has come into contact with them, through consumer products.
Unbeknown to many, today virtually all breakthroughs in experimental sciences involve some or all of these technologies. To cite a few: The discovery of the so-called “God particle”, the hunt for supernovae, the development of affordable genetic diagnostics.
LR: Real successes are accompanied by lots of hype, which I guess is inevitable in such a red-hot field as ML. One persistent misconception which irritates me quite often is the misuse of the word AI. I prefer to deal with concrete problems, algorithms and systems leaving philosophical questions to a later stage.
APO: There is also lot of hype about what quantum computing is capable of doing in the near-term. Although we are in an exciting time in history, I think we need to communicate clearly the challenges we face. Understanding and talking openly about these will guide the major developments in the field and it will help to balance the expectations from investors. I strongly agree with Prof. Wilhelm, from whom I recently heard: “quantum computing is really cool, even when we restrict it to the true facts”. I totally agree with this line, and I see it as an invitation to take with a grain of salt popular media articles and to follow closely what the experts say.
What is one scientific breakthrough that would not have been possible without these technologies that makes you excited?
DL: Jim Gray wrote eloquently about this, and pioneered many of the underlying technologies. The book “The Fourth Paradigm: Data Intensive Scientific Discovery”, was published in Jim’s memory by Microsoft, and is available (for free here). It surveys the advances brought about in many scientific fields – climatology, meteorology, medicine, neuroscience, genetics, astronomy, cosmology, astronomy and basic physics. The most significant breakthrough is that technology has changed the scientific paradigm itself.
LR:There are quite a few of those break throughs that greatly affect our everyday life already. An enormous variety of Amazon product selection, speed of order fulfillment and delivery would not be possible without Data Science and ML. Or imagine your life if suddenly all search engines go away - no Google, no Bing nor Yahoo are available. People are so accustomed to knowledge at their fingertips!
MLP: Unbeknown to many, today virtually all breakthroughs in experimental sciences involve some or all of these technologies. To cite a few: The discovery of the so-called “God particle”, the hunt for supernovae, the development of affordable genetic diagnostics, … and the medicines that saved many of our children’s lives. These technologies have already had a meaningful impact in our society, particularly in telecommunications, health care, consumer products and industrial applications.
APO: In slight contrast to the insights from my peers in the panel, I think we have to admit quantum computation as a potential supercomputing technology is still in its early stages. Therefore, we do not have a “breakthrough” yet demonstrating the real-power of the technology on a real-world application. Despite the huge potential and the rapid progress, the power of today’s quantum computers is comparable to that of early computers in the 50s or 60s.
On the other hand, there have been several developments inspired by research in quantum algorithms. One example, is the development of post-quantum cryptography, which encompasses a series of cryptographic algorithms expected to be robust against attacks from quantum computers. Also, ML is permeating all fields, and quantum computation is no exception here. We can see examples of ML techniques as a valuable tool in the characterization of quantum systems, and I expect to see more of this in the near-future.
Where do you see these technologies moving to over the next 5 years?
DL: The most dramatic recent advances have been enabled by Moore’s Law allowing us to build and run deeper neural networks. Despite rumours of its demise, the growth predicted so accurately by Moore continues. Coupled with advances in effectors, the technologies to interact with the physical world (robots, large and nano-scale, computer manufacturing, autonomous vehicles) there is likely to be a major shift in the economic landscape. I don’t think this will be another case of horseshoe makers becoming auto mechanics.
APO: I hope to see more and more quantum-assisted solutions at the frontier of ML and finance; this is certainly one of the foci within our company. These technologies represent a radically different computational approach, and we are experiencing a powerful momentum from investors propelling this academia-to-industry transition for quantum computing technologies. I am optimistic that we, as a community, will be driving algorithmic and hardware breakthroughs capable of providing unique solutions to real-world problems in the near-term (5-10 year span).
LR: Well, predicting the immediate future pays my salary at Amazon. But, caveat emptor, predicting technological advances for a 5 year horizon is probably impossible. Few speculations in no particular order: Digital assistants and self-driving cars are nearing practical usefulness. Dialog systems will be common place. Wide spread of ML in Healthcare, specifically in diagnosis and robotically assisted surgeries.
In an ideal world machines will comply with the law, always, when programmed to do so. The risk is that machines will be able to navigate the multi-dimensional maze of international financial regulations and to devise complex “heads I win, tails you lose” strategies that exploit conflicts of interest.
MLP: Finance is perhaps the last remaining sector of the economy that is still virtually unaffected by these technologies. Here we are, in the 21st century, when cult-like activities such as technical analysis still have greater following or assets under management than ML-based funds. Part of the problem is that finance is a particularly difficult field for ML. Standard ML techniques tend to fail when applied to investments problem for the reasons explained in the article here. Finance accounts for about 10%-20% of the GDP in the United States, depending on various definitions. That gives you an idea of the magnitude of the disruption that we are about to experience.
Finally, how can financial institutions benefit from adopting these tools?
LR: I think that financial institutions have always been on the cutting edge of technological progress and are actively adopting ML in general and Deep Learning. Specific to the financial field, quantifying risks and uncertainties are the areas of great importance which warrants further R&D efforts in ML.
MLP: Like other industries, financial institutions can benefit through gains in productivity, competitiveness, quality gains and margins improvement. In addition, there is one major benefit quite specific to finance: resolution of conflicts of interest. As emotional beings, subject to fears, hopes and agendas, humans are not particularly good at making fact-based decisions, particularly when those decisions involve conflicts of interest. In those situations, investors are better served when a machine makes the calls, based on facts learned from hard data. This not only applies to investment strategy development, but to virtually every area of financial advice: granting a loan, rating a bond, classifying a company, recruiting talent, predicting earnings, forecasting inflation, etc. Furthermore, machines will comply with the law, always, when programmed to do so. If a dubious decision is made, investors can go back to the logs and understand exactly what happened. It is much easier to improve an algorithmic investment process than one relying entirely on humans.
DL: Many routine financial analysis and administrative functions will be done by machines – white-collar robots. This is a good thing for the people looking to reduce head count, and not a good thing for the people whose heads are removed from the count. In a broader sense, I concur with Marcos’ comments, with a caveat. As he pointed out, there is one major potential benefit specific to finance: resolution of conflicts of interest. In an ideal world machines will comply with the law, always, when programmed to do so. The risk is that machines will be able to navigate the multi-dimensional maze of international financial regulations and to devise complex “heads I win, tails you lose” strategies that exploit conflicts of interest and loopholes reminiscent of the complex highly leveraged structures that gave rise to the financial crisis of 2008.
APO: My peers already mentioned several of the crucial advantages that machine learning and supercomputers can bring to the finance sector. Just imagine what you could do if you could solve these problems, not only faster, but to tap into problems we cannot even dream of solving today, even if we join the power of all the largest supercomputing centres in the world. This is the real potential power of quantum computing. Not as a minor improvement beyond the limitations of Moore’s law, but as a radically different approach with the potential of tapping into computational resources not available in the conventional classical computing models.