10 ways AI can supercharge trading
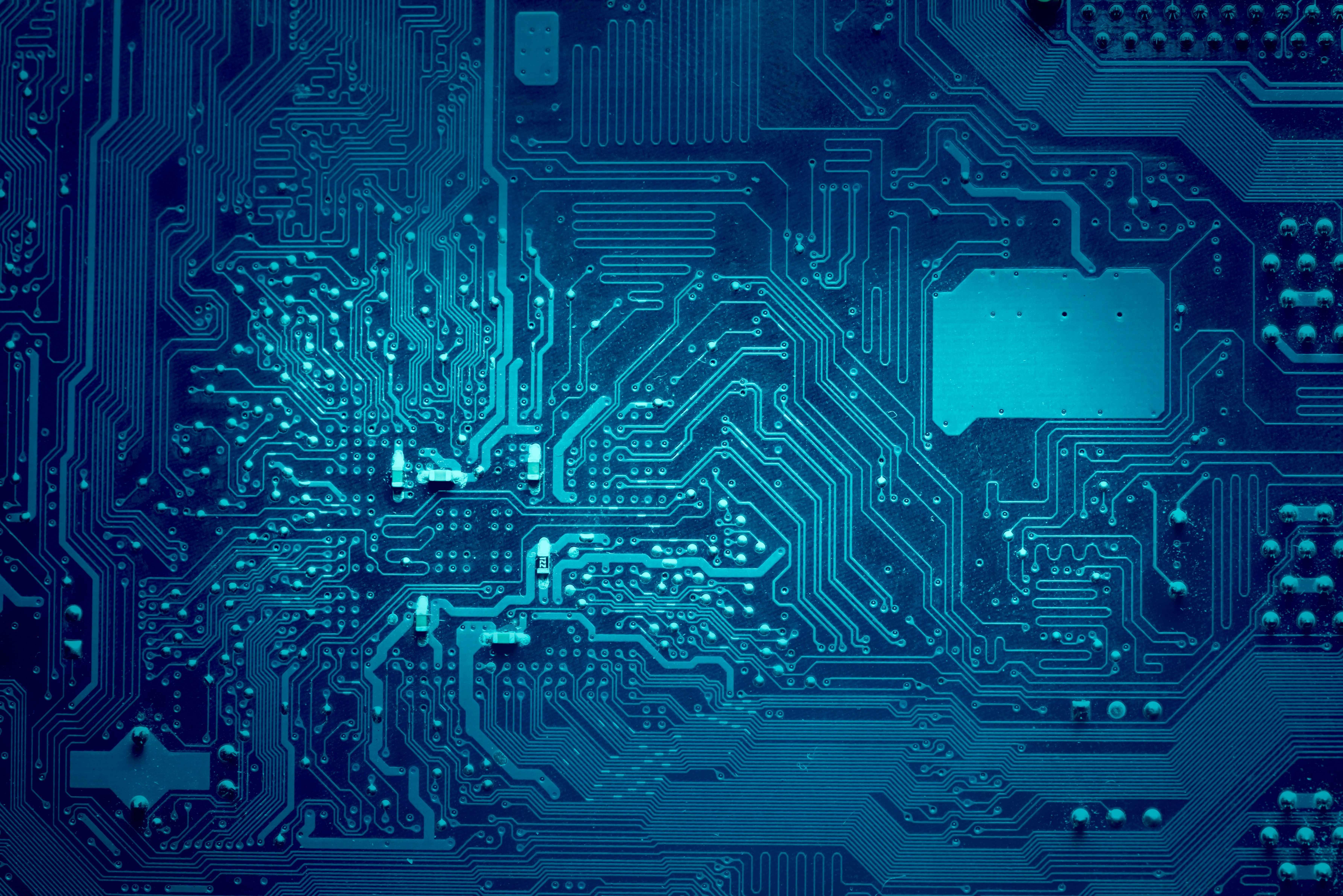
Machine learning is the big buzzword for the quant finance community, and it’s easy to get carried away by its promises. ML is already disrupting quant finance in 10 ways, according to Marcos López de Prado, Professor of Practice at Cornell University's School of Engineering, but it’s important to manage your expectations and understand the limitations of this technology, as Wim Schoutens, Professor of Financial Engineering at University of Leuven, cautioned us to do at QuantMinds International.
To what extent will artificial intelligence and machine learning alter the fundamentals of investing?
The answer involves seeing beyond the hype, according to Marcos López de Prado, Professor of Practice at Cornell University's School of Engineering, who outlined how technology is set to become central to finance at QuantMinds International. Also at the conference, Wim Schoutens, Professor of Financial Engineering at University of Leuven, showed delegates how machine learning can solve quant problems hundreds of times faster than traditional methods.
Computer power has long been touted as a revolutionary force in trading and investment management, promising to make predictions and even decisions without being explicitly programmed to perform a specific task. Even so, López de Prado warned delegates against using such technologies blindly.
Applications include modelling nonlinear relationships, learning and deciphering complex patterns, dealing with unstructured data and controlling for overfitting, he said.
Revolutionary force
“At the same time, you have to be careful not just to feed financial information to these algorithms,” he said. “They are not necessarily designed for financial series, so there are some difficulties.”
These are the top ten ways machine learning is disrupting the industry and offering potential for better returns:
1. Price prediction
Machine learning methods can help with the most fundamental trading task by enabling the modelling of complex relationships between many variables, and will ultimately lead to more accurate pricing.
2. Portfolio construction and risk analysis
Many investment firms are still allocating trillions of dollars using mean-variance portfolio optimisation, even though this is known to underperform other selection methods, López de Prado said.
“The method is not robust,” he said. “Numerically, the result is very unstable.”
In contrast, machine learning outperforms the current methods used and can give more reliable and robust estimates.
“These methods are able to extract whatever signals there are in the data and cancel them out,” he said. “These machine learning approaches are able to outperform.”
3. Outlier detection
When assessing financial data, even a small percentage of outliers can cause a very large percentage of wrong signals. That manifests itself in the generation of “buy” signals that should be “sells”, or in making assets or securities that are expensive look cheap, or cheap ones look expensive.
“Just 5% of outliers can produce carnage,” López de Prado said. “With a machine learning approach, you can reduce misclassification errors.”
4. Bet sizing
Machine learning can help decide how much money to put behind a trade or a strategy. Often a trader will know they want to buy or sell something, but not how much to put into the transaction. Using machine learning, algorithms can be trained to understand how much confidence an individual has in the recommendations they’re being given, and that will help size the investment.
“Bet sizing is a very unappreciated aspect of our business,” López de Prado said. “Should we go all-in, 50% of our budget, 10%? Based on our confidence in the bet, we can determine the bet size.”
5. Feature importance
Machine learning algorithms identify patterns and allow us to understand what features are more important, without knowing why. In traditional statistical analysis, key features are often missed as a result of the models’ misspecification, López de Prado said. Once we know what the factors at play are, we can develop a theory of how they work.
“This is where machine learning is incredibly useful,” he told the delegates.
6. Controlling for effects and interactions
Computer power and artificial intelligence offer a pathway away from traditional regressional approaches that have long formed the basis of econometrics and can be complementary to them. Machine learning algorithms can model complex interaction effects by allowing for hierarchical, non-linear and non-continuous approaches.
7. Credit ratings and analyst recommendations
Traditionally, stock analysts have used a number of different models and heuristics to produce their recommendations. Machine learning algorithms have been successful at reproducing a large percentage of recommendations produced by bank analysts and credit rating agencies. “The heavy lifting can be done by algorithms,” López de Prado said.
8. Sentiment analysis
Thousands of news articles and online snippets are produced every day containing information on stocks and securities. Harnessed properly these can offer a powerful sentiment indicator. Using machine learning algorithms, the information can be assessed, deciphered and used to indicate aspects like direction and volatility.
“The market is bombarded every day with all these articles,” López de Prado said. “Who is going to read them all? Nobody. We need an algorithm that can compare the evolving narratives.”
9. Execution analysis
This is a very obvious way that machine learning can help quants, exposing core issues in operations and strategy, and supporting decisions, he said.
10. Detection of false investment strategies
Most quant firms invest in false discoveries, said López de Prado. Machine learning can help here by identifying how many are taking place, and once you know how many there are, then you can deflate the sharpe ratio, he said.
Machine limitations
These examples showcase how artificial intelligence can help solve quant problems, making progress in areas like risk management and pricing. Even so, Wim Schoutens, from the University of Leuven, told the audience that it also has limitations.
“I am not a believer in too much artificial intelligence in trading,” he said. “As an investor, I want to understand why a trade is put on and I don’t know if artificial intelligence is something that can bring me that.”
Even so, he said there were many ways in which machine learning could be utilised to help make better decisions, since often, similar processes are repeated many times, for example repricing a derivative.
“With machine learning we can combine everything,” Schoutens said. “There is now a machine that is essentially applying old statistical tools and that can learn for you the things you have been doing over and over in such a way that it can speed it up dramatically.”
Speed vs accuracy
The trade off is that at a much higher speed there is some loss of accuracy, but this is reasonable, given the time gains are so large, he said. The biggest challenge facing the financial community is convincing regulators that what’s being done using these new technologies is fully understood.
Schoutens shared his new machine learning tools with the audience and showed how they can solve traditional quant problems. He explained the Gauss Process Regression (GPR) and compared its speed and accuracy with other methods.
Traditional methods of derivative pricing and risk management are time consuming and often rely on Monte Carlo simulations.
“From the moment the price comes out, it is essentially outdated,” Schoutens said. “So how could it have instant prices? That is where machine learning comes in.”
A model with a limited set of parameters can be trained, for a wide range of market situations, to come up with a price. The training is time consuming, but when it is complete and the computer has learnt the relationships, prediction is extremely fast.
Covariance matrix
GPR is a non-parametic approach. It finds a distribution over the possible functions that are consistent with the observed data, starting with a prior distribution and updating this as data points are observed.
Schoutens uses a covariance matrix to ensure that values that are close together in input space will produce output values that are close together. His method doesn’t model the relationship as one function, but as a distribution.
He showed an example produced using only five dots on a graph, and said the machine learning algorithm was able to come up with a solution with only a few small errors, and that it was about 600 times faster than the classical Monte Carlo pricer.
“I have generated an instant price,” he said. “And that takes me a fraction of a second.”