Truly Explainable AI: Putting the “Cause” in “Because”
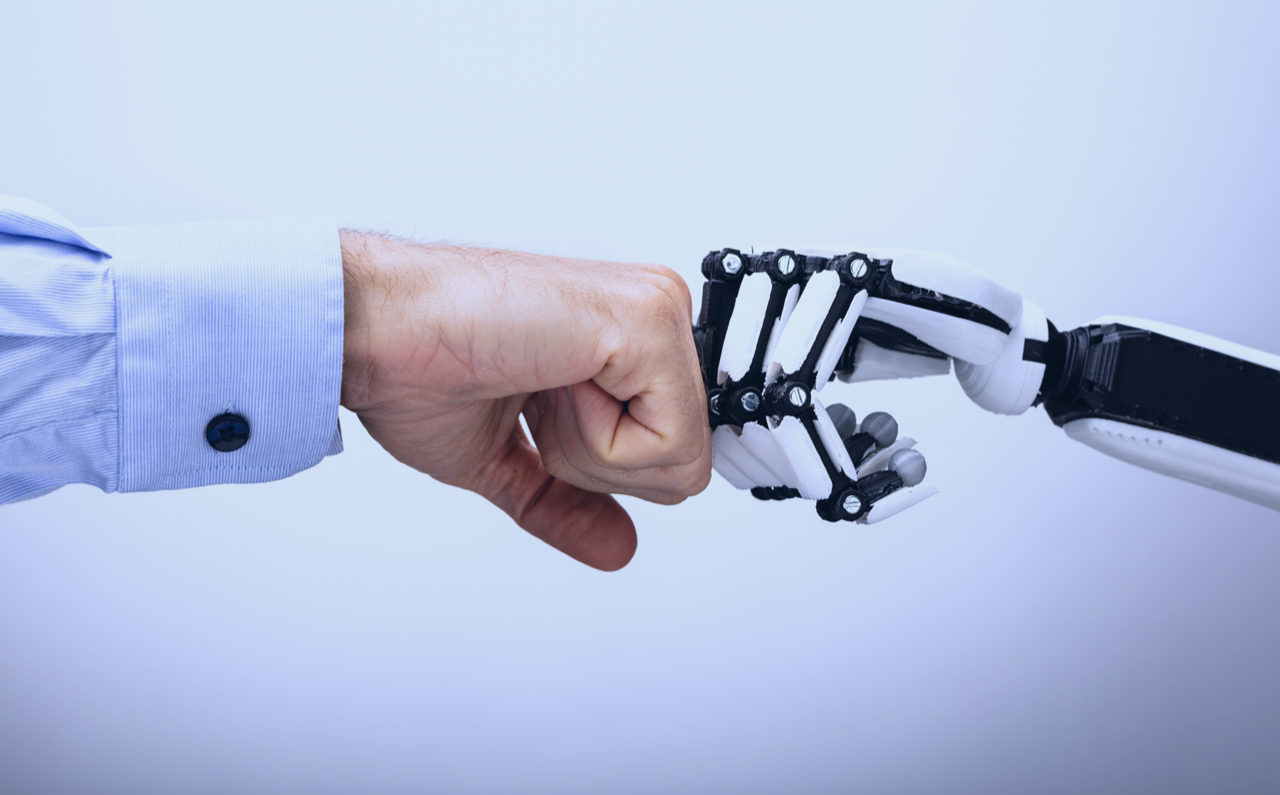
There are two serious problems with state-of-the-art machine learning approaches.
One is that the most powerful models are too complicated for anyone to comprehend or explain. For instance, a deep neural network is highly flexible — it can learn very intricate patterns — but it is essentially a “black box” that no one can look inside of. Conversely, more transparent models, like linear regression, are typically too restrictive to be useful.
---
A second big problem is that the more powerful learning algorithms, while amazingly successful in artificial environments like board games, often fail in real-world, dynamic, low signal-to-noise environments — such as financial markets or commercial sectors. This is because they “overfit” to past correlations, which may break down in the future.
A new generation of Causal AI technology solves both problems, generating highly accurate models which avoid overfitting, and that are also inherently explainable.
Causal AI achieves high predictive accuracy by abstracting away from features that are only spuriously correlated with the target variable, and instead zeroes in on a small number of truly causal drivers.
Crucially, models built with Causal AI are also highly transparent. They are lean, simple and uncluttered by noise. They reveal the systematic, causal relationships between input features and target variables that have been discovered in the data, and, moreover, render these relationships in intuitive visualisations.
While the benefits of increased prediction accuracy are obvious, the advantages of superior explainability are perhaps more subtle, but still hugely significant.
Explain to discover
Uniquely, Causal AI goes beyond existing approaches to explainability by providing the kind of explanations that we value in real life — from the moment we start asking “why?” as children. When we ask why something happened, we want an account of what caused it. Previous solutions in the field of explainable AI do not even attempt to give insight into causality; they merely highlight correlations.
Unlike conventional machine learning, Causal AI generates models that are both accurate & inherently explainable
However, demands for causal explanations animate scientific discovery and commercial research-and-development. Take a pharmaceutical company using machine learning in drug discovery. There are phases of the drug discovery pipeline that require insight and understanding: for instance, understanding disease mechanisms, or building evidence of target-disease associations. Clearly, accurate predictions alone are not good enough here.
In fact, the term “explainability” is widely misused in AI circles: it is typically used to refer to transparency into the logic of an algorithm; not an account of what conditions in the world caused the algorithm to make its decision. Causal AI goes beyond transparency in this narrow sense, generating the kind of real insight into the underlying data generating process that is invaluable in everyday life and scientific discovery.
Explain to interact
Consider an algorithm that’s deployed to balance an electricity grid by forecasting demand. Incorrect forecasting leads to suboptimality in the grid, with huge economic, environmental and human health costs. Should operators feel comfortable using an algorithm for this job that no one can comprehend? If no one understands what the algorithm is doing, then operators can’t evaluate its basic assumptions or refine them with expert judgment.
Causal AI facilitates more fluid interaction with humans. It makes its “thought process” transparent and it presents plausible hypotheses about potential causal relationships that domain experts can then narrow down and sharpen.
An anecdote goes that in the 1960s an undergraduate at MIT was tasked with solving the problem of computer vision as a summer project. All they established was that the problem was harder than suspected. Researchers have since recognised that computers are bad at tasks that humans are good at, and vice versa.
Causal AI combines the complementary strengths of both humans and machines: our rich world knowledge and intuitive grasp of causality can join hands with machine-enabled causal inference and simulation. This partnership allows us to begin to harness the full potential of AI.
Explain to justify
There is a basic expectation that we should be able to understand and contest decisions that impact us. What’s more, these expectations are enshrined in law. For example, the EU’s General Data Protection Regulation contains a “right to explanation”.
This is especially pressing in the context of the growing problem of algorithmic bias — a trend that may be entrenching existing disadvantages. Suppose, for example, that a minority person is assigned a low credit score by a biased algorithm. They are likely to want, and deserve, an explanation as to why their loan application has been rejected, and they are unlikely to be satisfied with being told “because the computer said so”. In the United States under the Equal Credit Opportunity Act, lenders are legally compelled to justify their credit decisions. Causal AI delivers on these basic social and legal expectations.
Further, Causal AI has the potential to prevent algorithmic discrimination in the first place. An obvious solution to algorithmic bias does not work: when protected characteristics, such as age or race, are withheld from datasets, bias persists or, surprisingly, gets worse. This is because the algorithm can often recover the information that has been held back. Causal AI can help towards a solution by highlighting features in the dataset that are causally related to withheld information, helping tech makers to rule out covert discrimination.
Explain to trust
Explainability is also needed for us to have confidence in our algorithms. If we cannot understand what they are doing, then we cannot trust that they will continue to perform well in production. And, absent explainability, if predictions go wildly wrong then no one can find out what happened, debug the algorithm and upgrade the system to prevent the problem from recurring. It is little surprise then that, of the seven key requirements for trustworthy AI set out by the European Commission, three pertain to explainability.
Causal AI meets our fundamental social & legal expectations for justification & fairness
If AI is to meet our basic social, ethical, legal and human use requirements, explainability is not just an optional bonus feature — it is indispensable. It allows us to audit, trust, improve, gain insight from, scrutinise and partner with AI systems. These are all attributes that we expect of important decision makers in society. We should expect the same of AI systems, as they progressively replace and augment human judgment. Unlike conventional machine learning technology, Causal AI promises to meet these expectations without compromising on performance.
About us
causaLens is pioneering a completely new approach to time-series prediction. Its Enterprise Platform is used to transform and optimise businesses that need accurate and robust predictions – including significant businesses in Finance, IoT, Energy and Telecoms.
Almost all current machine learning approaches, including AutoML solutions, severely overfit on time-series problems and therefore fail to unlock the true potential of AI for the enterprise. causaLens was founded with the mission to devise Causal AI, which does not overfit, and so provides far more reliable and accurate predictions. The platform also includes capabilities such as autonomous data cleaning and searching, autonomous model discovery and end-to-end streaming productisation.
causaLens is on a mission to build truly intelligent machines that go beyond current machine learning approaches - a curve-fitting exercise. Devising Causal AI has allowed us to teach machines cause and effect for the first time - a major step towards true AI.
causaLens is run by scientists and engineers, the majority holding a PhD in a quantitative field. Contact us on info@causaLens.com or follow us on LinkedIn and Twitter.