Unexpected bug in your machine learning: how can you recover?
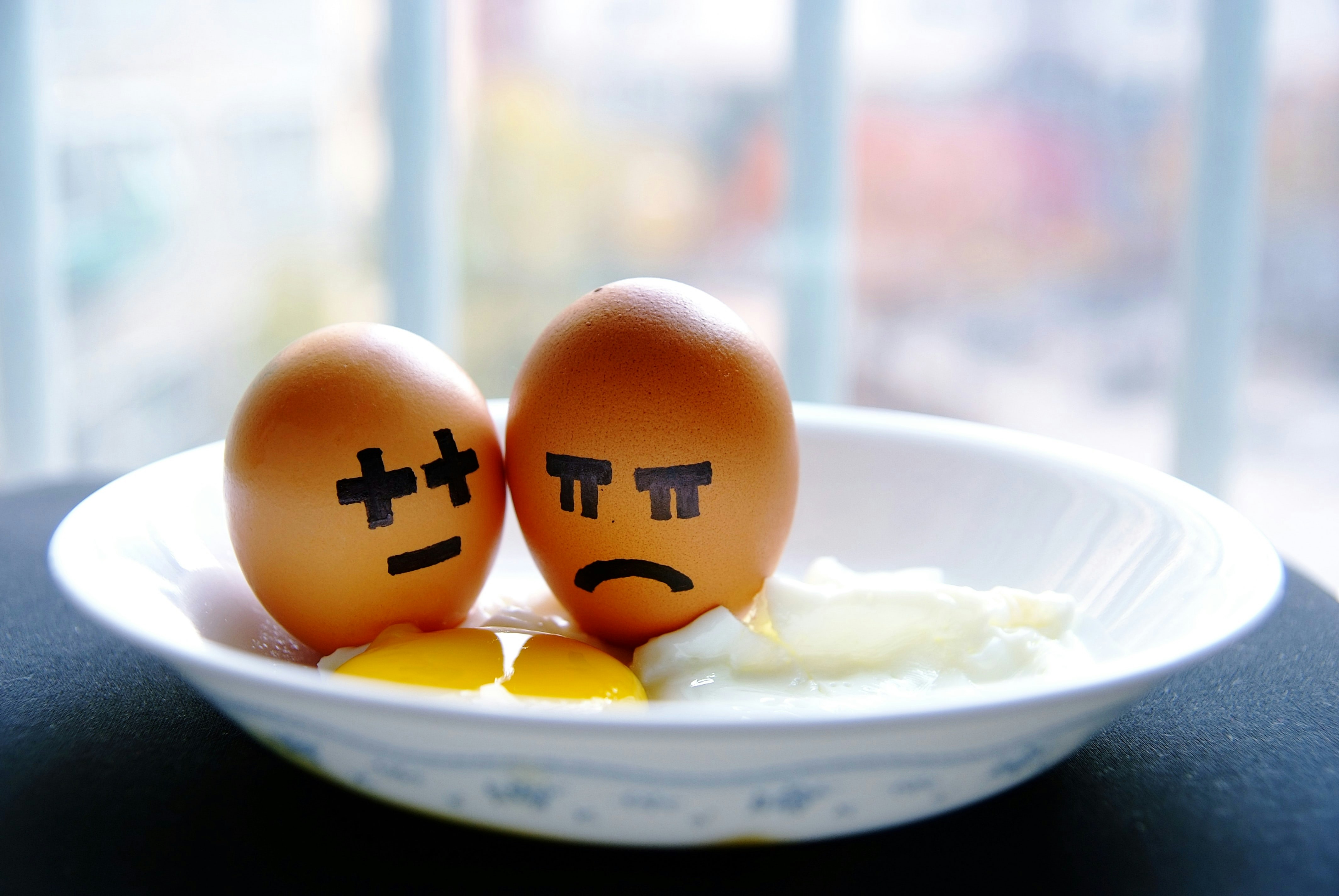
Although machine learning has been a huge part of quant finance for a while now, there is a reason why complex ML models haven’t become mainstream… yet. There are so many obstacles that need to be overcome from computing power to the available man-power that’s needed to even assemble the models. So when you managed to put the model together, how can you improve it and troubleshoot? Maurizio Garro, Senior Lead IBOR Transition Programme, Lloyds Bank, assesses the building blocks of ML models, and explores its implications on the wider business.
In recent years, financial institutions have been investing in the development of machine learning (ML) to deliver additional value to their customers and products. The exponential growth of the development of ML has been possible by the evolution of the technology which has allowed us to use a larger amount of data (including unstructured data) and to increase computing powers. ML can be used to support business decisions in many areas like asset management, customer relationship management (CRM), fraud detection, credit risk management, and regulatory compliance.
Given the intrinsic complex and dynamic nature of ML, the possibility of failure is not a surprise. There are many reasons why this can happen. One of them is bias in the training data and method (e.g. sampling, data preparation) as the scientist can select many options to train a ML with different associated outcomes. Another reason is that the ultimate scope of the ML is not well defined and transparent and does not match any specific business requirements. Further issues are linked to the machine learning techniques which are not able to inform us when the information is not clear, or they cannot effectively learn from the data. Furthermore, ML uses a high number of hyperparameters (e.g. how many trees I consider in a decision process like random forest). These hyperparameters are defined by the developer and they cannot be derived by the data, so they introduce a human bias. Finally, algorithmics cannot understand the context of the language used, so they may learn letters, words, sentences or even the syntax, but where they fall back is the context of the language. The points above can help to understand the “black box” nature of the learning mechanism of the ML which requires extra care when we want to rely on algorithms to make decisions.
Of course, perfection always starts with mistakes. So, how can we make the ML a better place? The starting point is the data. First of all, it is important that data are accurate, complete, and sufficient to extract statistically significant insights. Data inputs must be interpretable, coherent with the internal policy of the firms, and have a business rationale. In addition, we need to have a robust approach to pre-process the data to avoid any corrupted learning process. Another important point is the calibration. As we know, this is a crucial part of the traditional models and it is even more important for ML given the number of parameters, data, and the frequency they are updated. In this case, we can establish specific controls to assess if the calibration is appropriate and develop a monitoring framework including thresholds and triggers to inform if the model is working as expected.
Naturally, the above requires some changes in the way we review model risk for ML. A current challenge for financial institutions is how to review and amend the model governance policy to reflect the features of the ML discussed above. Each firm must define if the change should be at policy, standards, or procedure levels. In addition, the enterprises must decide if they want to track the new developed ML in the existing model inventory or create a new one specific for them. The key driver of the direction is the risk appetite that the firm has for model risk as this will determine the basis of their model risk management framework. This requires the involvement of the senior executives of the firms as they need to receive appropriate information to make an informed decision about the role ML can play in their firms.
Secondly, the validators must be sure they are equipped with the right tools and skills to deal with the big data and computation complexity behind the ML exploit. While the appropriateness of the skills and knowledge of the model validation team is a not a new topic, the exponential growth of ML has highlighted the importance of the tools the model validators need to perform their tasks. In addition, given the higher complexity of the ML from a data and methodology perspective, it is crucial that the model validators define a clear validation plan for each model where they describe the specific tests to perform, the rationale to use those tests and explanation of the possible outcomes with implications for the model under review.
As final remarks, it is important to be aware that ML can provide a lot of benefits in terms of accuracy, predictive power, and actionable insights. However, these come at cost of a higher complexity from a model risk management perspective which require to fully understand the peculiarities and challenges of the ML.