What are the latest innovations in quantum machine learning?
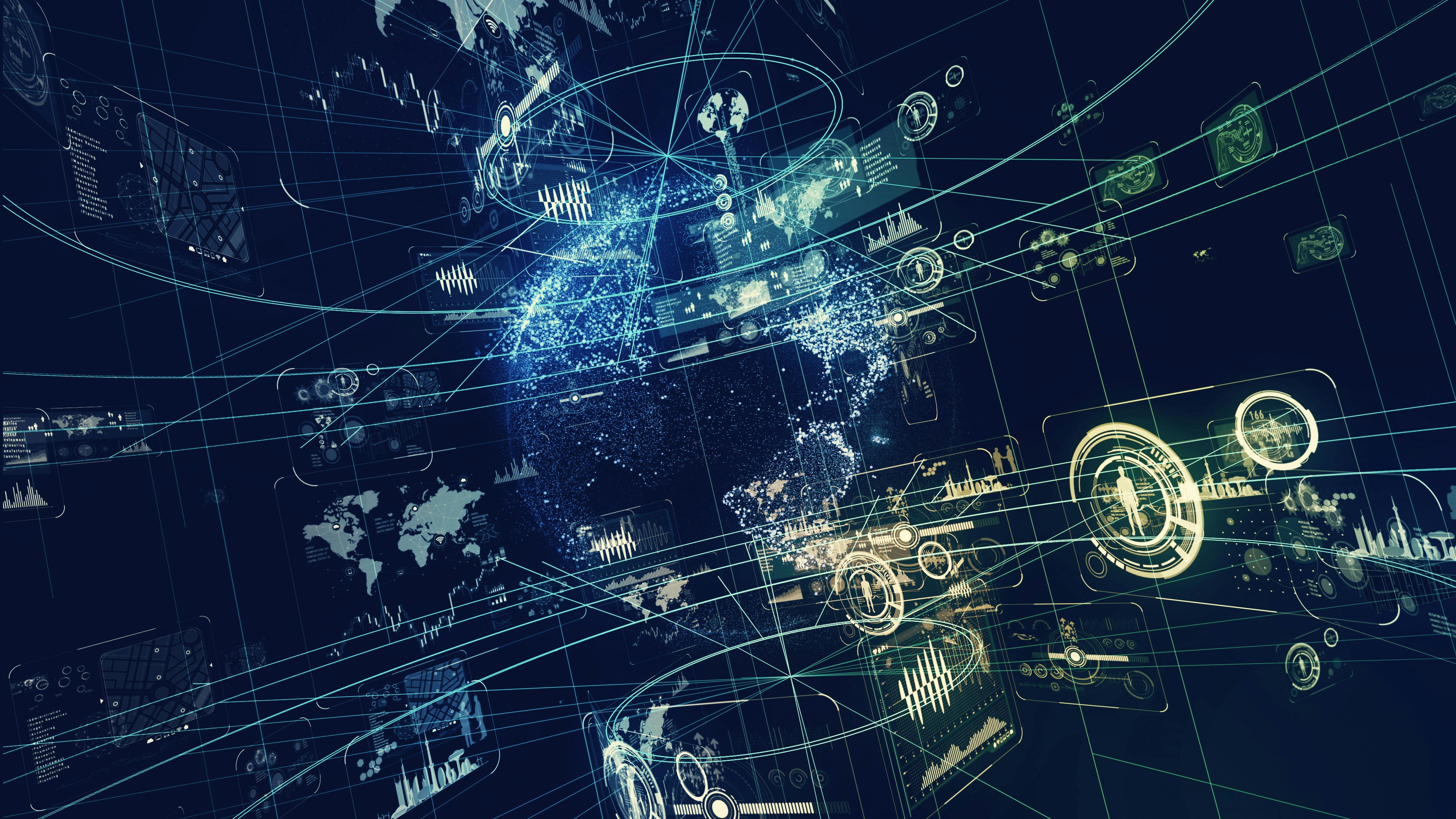
Quantum computing has been on our radar for a while now, but 2019 has brought on some exciting leaps in the technology, including quantum neural networks. In this article, Alexei Kondratyev, Managing Director, Global Head of Data Analytics, CCIB, Standard Chartered Bank, summarises the most promising breakthroughs in quantum computing and applications of quantum information theory, while exploring the opportunities that this presents quant finance.
Quantum computing and AI are going to revolutionise and disrupt our society in the same way as classical digital computing did in the second half of the 20th century and internet did in the first two decades of the 21st century. Quantum computing (and, more generally, quantum information theory) has been the subject of extensive research since the 1960s, but it has only been in the last decade that progress in quantum computing hardware made it possible to actually test the quantum computing algorithms and it was only last year that the quantum computing supremacy was finally established as an experimental fact (Google’s 53-qubit Sycamore quantum chip).
The story of quantum computing is in this respect similar to the story of AI: AI was born in the 1950s but then experienced two “winters” – two periods when the interest in AI and machine learning declined considerably – before becoming widely used and adopted to the point that we can no longer imagine our life without it. Even though we cannot rule out a “quantum computing winter” before quantum computing technology becomes embedded in our everyday life to the same extent as the internet, smart phones and AI, the whole range of quantum computing breakthroughs we’ve witnessed in the last few years makes it somewhat unlikely.
With recent advances in quantum computing technology, we have finally reached the era of noisy intermediate-scale quantum (NISQ) computing. NISQ-era quantum computers are powerful enough to test quantum computing algorithms and solve non-trivial real-world problems – and establish quantum speed up and quantum advantage over comparable classical hardware. However, it is likely that the first real-world production level business applications of quantum computing will be as part of a hybrid quantum-classical computational protocol, where most of the computation and data processing is done classically but the hardest problems are outsourced to the quantum chip (for example, discrete portfolio optimisation problems, which are NP-hard for classical computers).
Also, it is a combination of quantum computing and AI that will likely generate the most exciting opportunities, including a whole range of possible applications in finance, but also in medicine, chemistry, physics, etc. We have already witnessed the first promising results achieved by quantum neural networks (QNN), with many more to come soon.
Similar to their classical counterparts, QNNs can be trained as either discriminative or generative models. The main difference between QNNs and classical neural networks is replacement of the activation functions (which transform the signal going through the network) with quantum logic gates (which transform the state of the quantum mechanical system). A combination of 1-qubit and 2-qubit logic gates can create a complex entangled state which may be seen as an analogue of a non-linear transformation in the classical neural networks.
In discriminative machine learning context, a QNN can be trained as a classifier with the help of a classical backpropagation algorithm that adjusts parameters of the quantum logic gates (in classical case, backpropagation is used to update the weights associated with the network connections).
Generative learning is another promising QNN application area. In deep learning, a well-known approach for training a deep neural network starts with training a generative deep belief network model, typically using contrastive divergence algorithm, then fine-tuning the weights using backpropagation or other discriminative techniques. However, the generative training can be time consuming due to the slow mixing of Gibbs sampling. Also, generation of new independent samples when working with restricted Boltzmann machine (RBM) can sometimes take too much time due to the need to “thermalise” the system. These problems can be efficiently resolved when working with the quantum circuit-born machine (QCBM), a special type of QNN which is a quantum counterpart of classical RBM.
One of the main motivations for working with QNNs is the fact that some types of QNNs have larger expressive power than comparable classical neural networks. This makes experimenting with QNNs a promising avenue for establishing real-world practical quantum advantage.
At the same time, it is important to keep in mind that we still need significant advances in science and in systems engineering to fully realise the potential offered by quantum computers. The challenges ahead of us are enormous but the progress over the last several years was equally spectacular. With so many potential applications of quantum computing in finance (portfolio optimisation, generation of synthetic market data, data anonymisation, and secure communication, just to name a few), we are entering the new exciting period of quantitative finance development.