What is alternative data and how can quants approach it?
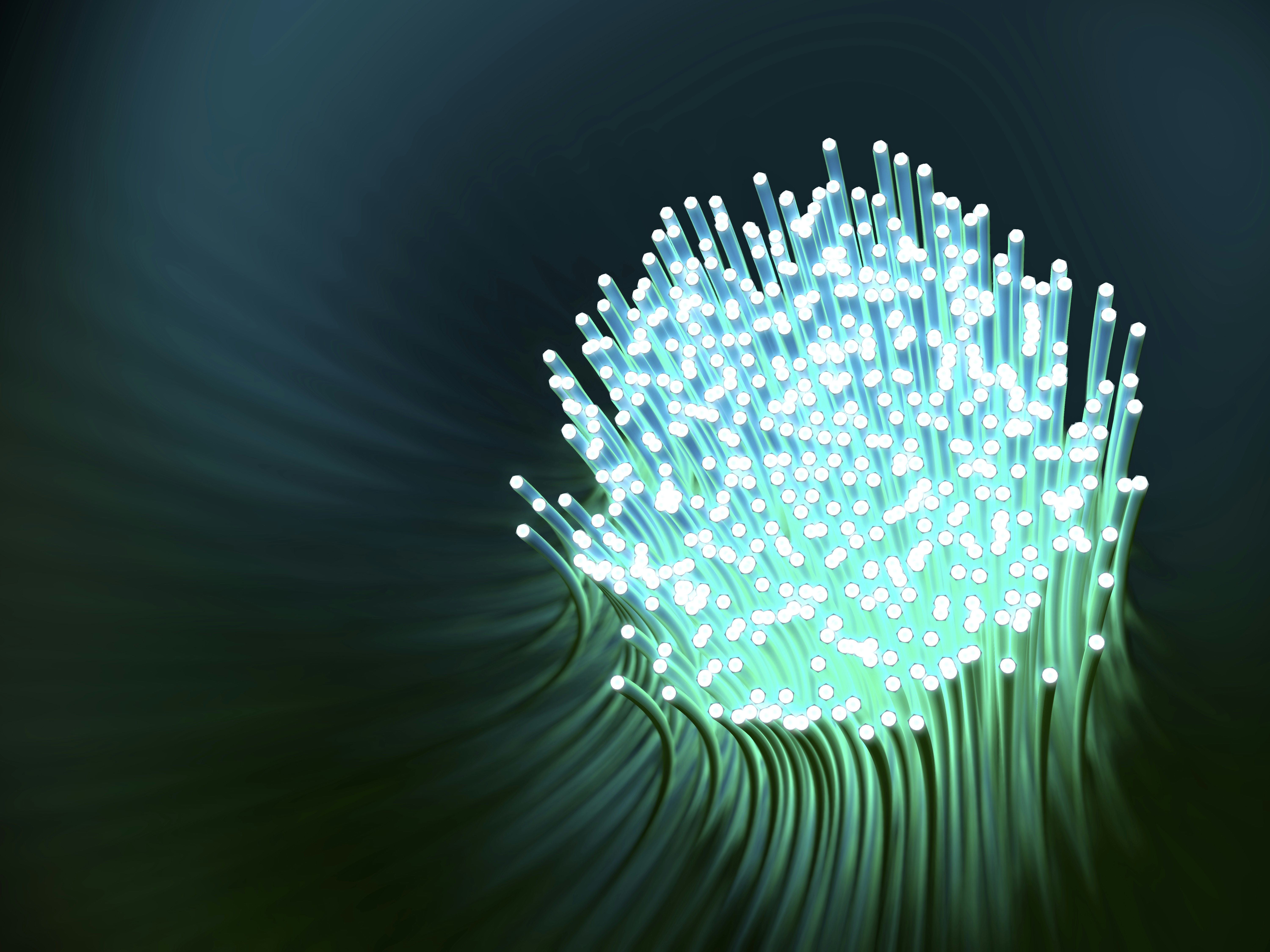
The concept and term of alternative data is no news to the quant finance community, but there's still a lot to learn about how to leverage it. Saeed Amen, Co-Founder of Cuemacro, gets back to the basics to define alternative data, the types of it, and the challenges of working with it.
Buzzwords abound in finance. One of the biggest buzzwords at present is data, and in particular alternative data. It is an area which I’ve been interested in for a number of years, before the phrase of alternative data became more commonplace. At present it is a major focus of mine, as Alexander Denev and I are co-authoring “The Book of Alternative Data” which will be released on Wiley in early 2020.
The key question to first understand is what is alternative data? One of the simplest definitions is simply data that is relatively unusual. What constitutes alternative data can obviously change over time. In the distant past having access to readily available daily price data might have seemed exotic, clearly this is not the case today. As time passes datasets inevitably become more commoditised within the investment community.
In recent years, the amount of data being generated has increased significantly, as has the ability to store it. Often data is generated as a derivative of other processes, and this type of data is referred to as exhaust data. Take for example an airline which records passenger details and numbers as part of everyday business. This data is a derivative of its activity, but conceivably of interest for investors.
For example, if we have an idea of shifts in business class travel, it might be an indicator for the broader economy, the premise being that business class travel tends to be one of the first things cut, when budgets are tightened. At an individual level, we also generate a considerable digital footprint, for example through mobile phone usage, which creates an exhaust of our web usage and also location.
There are many different datasets which can be considered as alternative, some are text based, these include news, social media and web based sources. Other sources, can include images, such as those available from satellite imagery, which can be used for a number of diverse use cases, including estimating crop yields, understanding GDP and also levels of oil storage. Data aggregated from credit card transactions is also a big area in alternative data and is often used to forecast retail sales on aggregate and also at a company level. In many instances, the initial raw data does not exhibit a common format and often needs considerable cleaning, in other words it is unstructured.
A key problem in alternative data is how to convert unstructured datasets into structured datasets, which exhibit a more consistent format, such as a time series. Once we have structured datasets, it is easier for it to be integrated into the investment process. In practice, most of the time and effort of working with alternative data goes into the cleaning and structuring process.
There are of course key legal questions to be asked with the distribution of alternative data, in particular with the advent of GDPR. Whereas an aggregated dataset, which blurs personal details might be usable externally, clearly, a dataset where individuals are clearly identifiable is not. A large number of alternative datasets also use data gathered from publicly accessible websites. Again, there are questions about which web pages can be accessed in this way and which would violate terms of usage. If a website is behind a paywall, such an approach is very likely to violate any sort of subscription agreement.
Ultimately generating returns is a product of several factors, notably capital and data and the time spent on crunching that data to come up with actionable trading ideas. If we can expand our dataset, theoretically we might be able to come up with better insights to provide us with an edge.
That, of course, is the theory, but the key of course is adding relevant and useful data, which improves our signal and this requires considerable research work. Just because a dataset is an unusual doesn’t necessarily mean it will add value to the investment process. In practice, a good approach is to have an economic prior and then to try and find an alternative dataset which can be used to confirm (or disprove) that prior. This can help us reduce the likelihood of finding spurious results.
Of course, the flip side is that maybe it will also eliminate valid results, that we might have found using a more data driven approach, which is more common in the machine learning community.
This article was originally published in our eMagazine Quantitative finance in the digital age.