What is idiosyncratic Alpha?
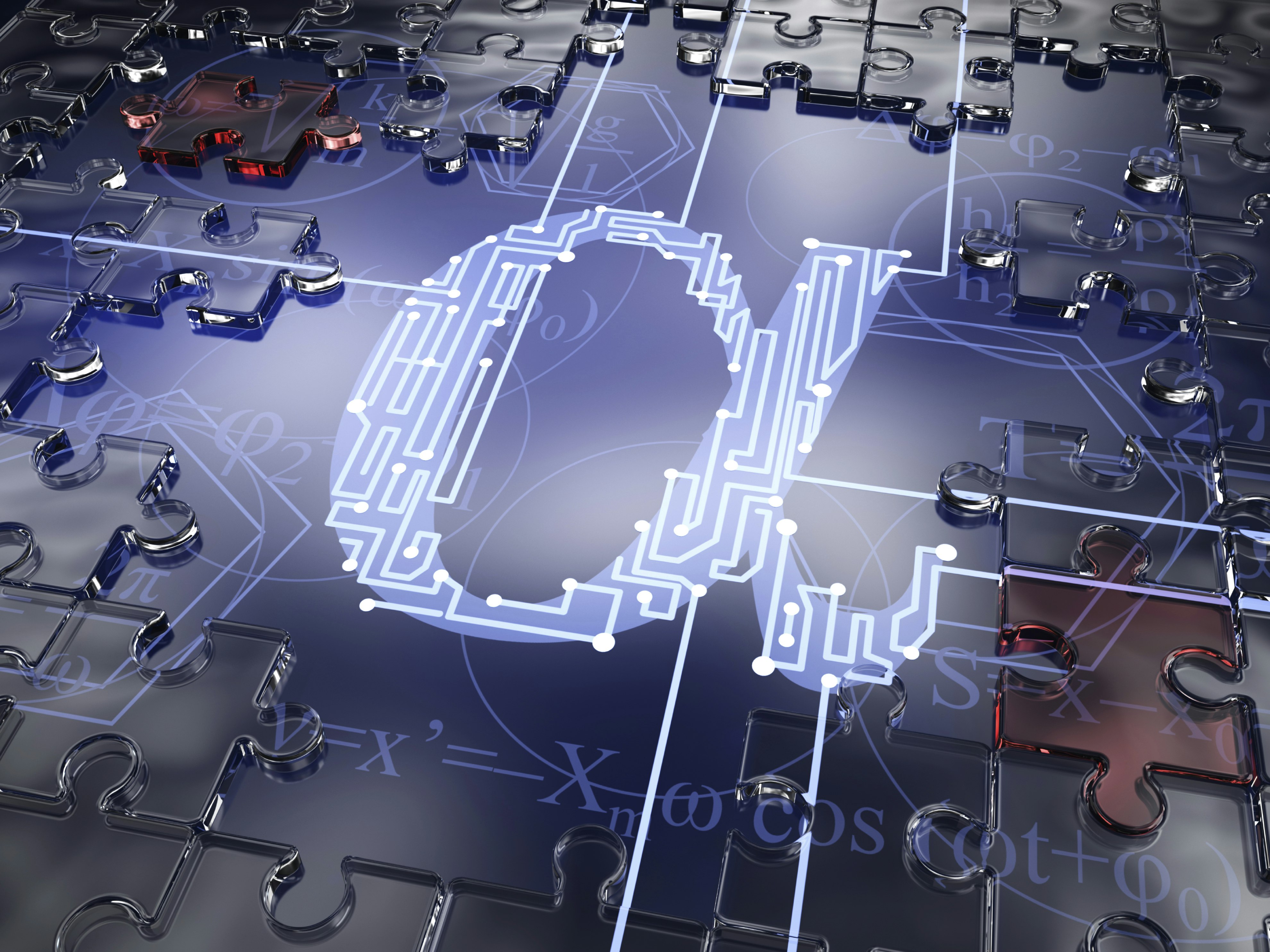
Michael Harris, quant systematic and discretionary trader and best selling author puts idiosyncratic alpha strategies under the spotlight to explore what they actually mean for quants.
A reference to idiosyncratic trading strategies was made in a market commentary by Neal Berger, the President of Eagle’s View Asset Management. In this article we attempt to clarify what these idiosyncratic strategies are.
Below is an excerpt from Neal Berger’s market commentary as reported by Matthias Knab (added emphasis is mine.)
In sum, we believe quantitative strategies still have a place in our portfolio. Traditional and more ‘pedestrian’ quantitative strategies such as fundamental factor, momentum, and mean-reversion based statistical arbitrage do not. We have already, or, are in the process of exiting those strategies and Managers who we believe run more pedestrian quantitative strategies that have not recognized or kept pace with the increased competition in quant and the reduction in available alpha due to the reasons mentioned above. While we are reducing quant broadly, within quant, we are increasing our allocation to strategies and Managers who run idiosyncratic and highly capacity constrained strategies that either require a highly specialized skill-set and knowledge to effectuate, or, are simply too capacity constrained to attract competition from the larger players.
The reasons mentioned for the reduction of alpha are primarily due to crowding effect in “pedestrian strategies” and less availability of dumb money to profit from. It was argued that a way out of this conundrum for some funds at least is through a shift to idiosyncratic alpha.
Idiosyncratic strategies are certainly not the strategies that are fully described in a few lines of code in some popular books.
Let us start with this definition:
idiosyncratic: peculiar or individual.
According to the above definition then, popular strategies, including trend-following, cross-sectional and absolute momentum, statistical arbitrage, including long/short market neutral, do not offer idiosyncratic alpha since they are widely known and are of high capacity. For example, CTAs are being already impacted by relying on high capacity and widely used strategies; alpha has diminished and CTAs are now trying to market these strategies in the context of low correlation with stock market and alternative beta; i.e., the past high absolute return potential is now gone, for good according to Neal Berger.
Therefore, we know what idiosyncratic strategies are not about. They are certainly not the strategies that are fully described in a few lines of code in some popular books. Below is an effort to identify a few of these strategies as the potential domain is large but difficult to fully research.
Idiosyncratic alpha strategies
Event and sentiment driven
Event-driven strategies attempt to generate alpha from corporate events that include mergers, acquisitions, earnings surprises, bankruptcies, CEO replacements, debt restructuring, to name a few.
Sentiment-driven strategies are based on analysis of news and social media feeds for determining sentiment and also trend.
I talk briefly about these strategies and their perils in Chapter 8 of my book Fooled By Technical Analysis. In a nutshell, data-mining bias and generation of spurious correlation are their main problems. These strategies are hard to backtest, but that is not necessarily a major drawback because any strategy that cannot be backtested cannot also be easily replicated. However, there is no conclusive evidence that these strategies can be effective.
It is quite unlikely that any fund can rely solely on the above sources of alpha.
Discretionary technical analysis
Technical analysis based on the use of trendlines, chart patterns and simple indicators of price and volume is fundamentally a random trading method for the majority of those who practice it. It is also acknowledged that a small percentage of technical analysts have managed to profit consistently but the cause of this is not attributed to predictive power of technical analysis but to their understanding of market structure and operation. In effect, technical analysis is used not as a prediction tool but for identifying attractive market entries and exits.
Obviously, such a method cannot be tested by quants. The proof, if any, is in actual performance records. Even in that case analysis must be done carefully to differentiate between skill and luck. This is because given a large number of random traders using technical analysis, there is high probability of some of them generating large returns. Therefore, one must also look at the consistency of those returns in time and how they are affected by any outliers.
Based on my own, but limited experience, with hedge funds, not many managers will allocate to traders using technical analysis because they are concerned about confirmation bias and other cognitive biases that may be present in decisions.
Machine Learning models
The fundamental problem of Machine Learning (ML) is the bias-variance trade-off. Supervised classification requires availability of a set of features, also known as predictors, factors or attributes. Simple models have high bias and low variance and more complex models have low bias and high variance. In a nutshell, as the number of features increases, the bias decreases at the risk of over-fitting to noise. As the number of features decreases, the models tend to under-fit new data. There is no easy way to find the optimum trade-off. More importantly, feature engineering is a key aspect of ML but it is more of an art than a science.
The problem [with ML strategies] lies in the tendency of new quants to think that the solution depends on the sophistication of the ML algorithm rather than on the quality of the features.
There are several quantitative funds that try to generate alpha via ML. Numerai provides encrypted features to data scientists to use in developing models. The data scientists upload their predictions and these are evaluated by the fund operator to determine their compensation. Last time I used the data from Numerai there were 21 features. This rich set of features may result in high variance predictions. The operator hopes to reduce the variance by taking an ensemble of prediction but this may guarantee only low equity variance but not the equity trend, i.e., fund equity can fall regardless. This can happen because most of the data scientists use more or less the same models, which they also discuss in various forums and blogs. In other words, it is questionable that model variance can be minimized by taking the ensemble of low bias/high variance predictions. However, this is a more interesting approach as compared to that of Quantopian, where a large universe of securities (in the order of 1500) is used to develop market neutral long/short equity strategies with ML employing known factors. Risks from inability to short a large number of securities, over-fitting in training set and the high variance in the test set increase risks of large and rapid drawdowns, something that is unlikely to occur in the case of Numerai due to the prediction ensemble approach.
My approach to tackling this problem focuses on feature engineering. DLPAL LS software generates a small set of idiosyncratic features that can be used to develop algorithmic and Machine Learning models. This approach may realize a better bias-variance trade off. An example for the Dow 30 stock universe can be found here.
A number of funds have decided to explore ML but in my view the problem lies in the tendency of new quants to think that the solution depends on the sophistication of the ML algorithm rather than on the quality of the features. As a result, these funds may experience losses.
Discretionary quant methods
These methods essentially take subjective technical analysis to the next level of evidence-based analysis. In this approach all models are required to have a clear logic that can be coded to backtest performance.
It sounds too good to be true and it actually is.
The fundamental problem of discretionary trading models based on some perceived anomalies is that even if they appear to generate alpha the sample size is small. This is evident recently from all the backtested indicators in social media and blogs that try to forecast the next market top: the sample size is much less than 20, in most cases less than 10. The reason for this is that high profitability anomalies are rare. Given this problem quants must find ways of validating the prediction of these models. Most of those who present backtests of this kind in blogs and social media never discuss validation and the possibility of a spurious correlation. The main reason for that is that backtesting for most of these analysts is a means of confirming their bias. As a result, they present backtests that only confirm their views.
There are ways of validating discretionary quant methods but backtests of the method are nearly impossible to generate. As a result, these methods will lack behind in preference of fund managers and long performance records will be required to screen traders.
This article was originally published in Price Action Lab Blog >>
If you have any questions or comments, happy to connect on Twitter:@mikeharrisNY