A point of inflection for Model Risk Management
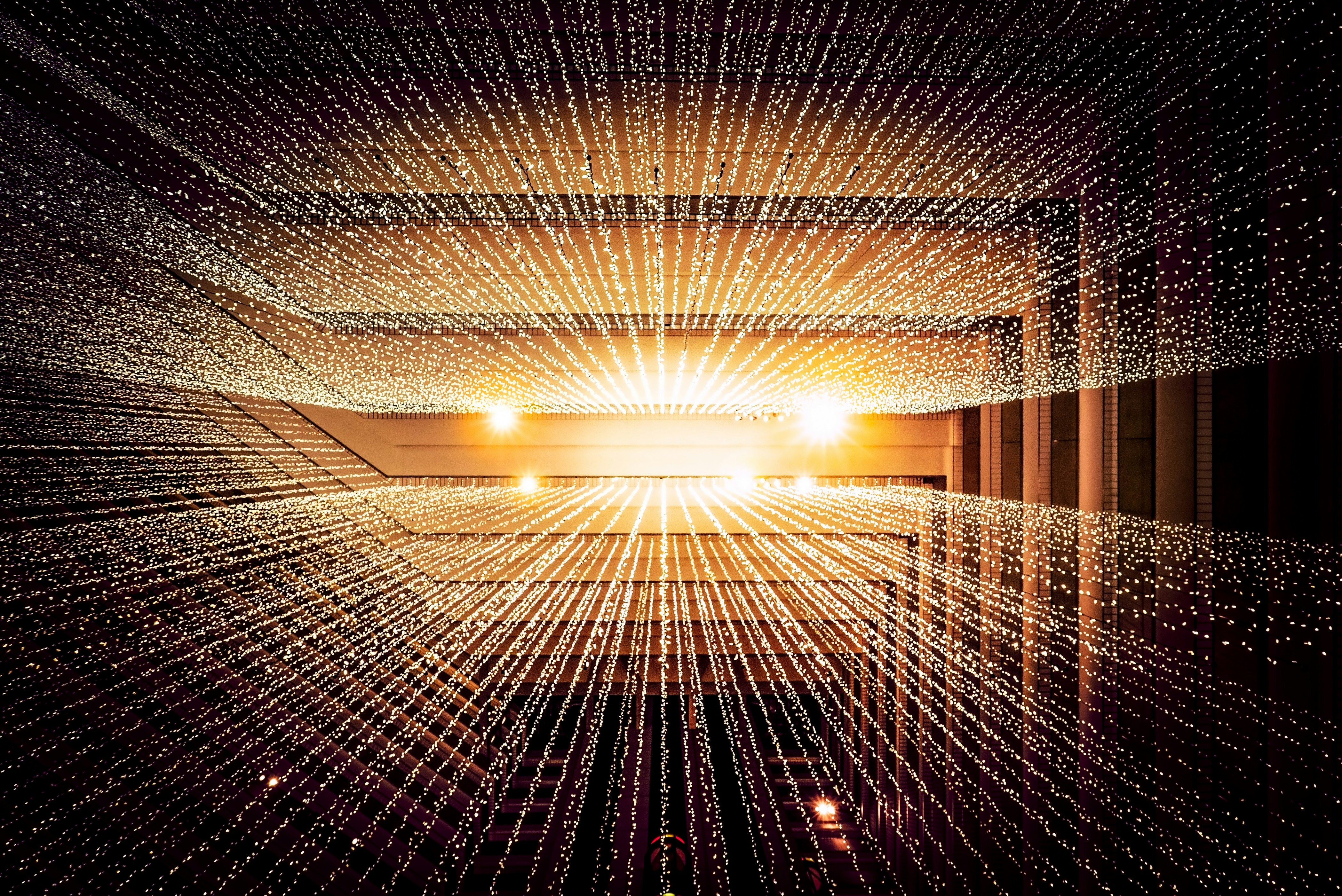
“If there's one thing that's certain in business, it's uncertainty”; what is required is a mindset change from running ‘certain’ mathematical tests to actively managing return on model uncertainty.
The cold war years
Many people outside the quant risk space, associate the start of model risk management with the publishing of the FED’s seminal paper on the topic (SR11-07), but model risk managers ‘in disguise’, aka model validators, were employed by banks with the mandate to test the models much earlier. An interesting breed of mathematicians and programmers had been brought in from academia to form those teams in most of the developed houses.
In the era of financial engineering frenzy, there was an undeclared cold war between the banking ‘academics’, the ones that occupied themselves with coming up with the new ground breaking stochastic differential equations (and hopefully solving them to win a Nobel prize) and those on the control side that had to prove these models were not perfect. Structurers had a new complex product idea, model developers would find an equation to price it and model validators would identify hot spots where the model would fail. However, as a beloved colleague of mine would say…’so what?’ The outcome of the tests, which many times were admittedly based on whatever the validation academics were interested in testing rather than what was necessarily impactful, were not really triggering any actions…until of course a new, better, model came out from a researcher.
At that time, model validation was seen as a control that ensured that the model was implemented correctly and operational risk was mitigated. Little concern was given to the actual number the model was spitting, simply because there was no single ‘right’ number.
Managing return on uncertainty
People are still debating what the future of model risk management is, but for me it all comes down to the realization that a bank needs to take model risk to achieve a return and this is what I call return on model uncertainty. Think of a bank that decides to be a market maker in a new product/market, and develops a ground breaking new model to price the transaction; the trader should require that the spread they are earning, on top of the traditional market risk, counterparty risk etc., reflects that the base price, is actually pretty uncertain due to the model. The objective truth is that there uncertain pricing and the potential cost requires a return.
So the million dollar question (or whatever the right number is?) for a senior manager on the verge to decide the firm’s fate is: ‘What do I need to know on top of the model’s output in order to make a decision?”, and although I love detailed answers, it cannot be that they need to understand the model deficiencies, the numerical uncertainties etc…or for that matter have a coffee with the professor that came up with the equation of the model one rainy afternoon in Cambridge.
Banks need to be able to identify, measure and manage model uncertainty for the model they use in each use case. The answer lies with a new species of risk manager (not in disguise anymore), i.e. model risk managers, who proactively seek to identify the adjustments required to the model output to facilitate appropriate financial decision making.
Coming up with a ‘correct’ model is an illusion, so let’s rechannel the cold war brainpower to try and quantify what is wrong with them!