Climate change related business interruption risk
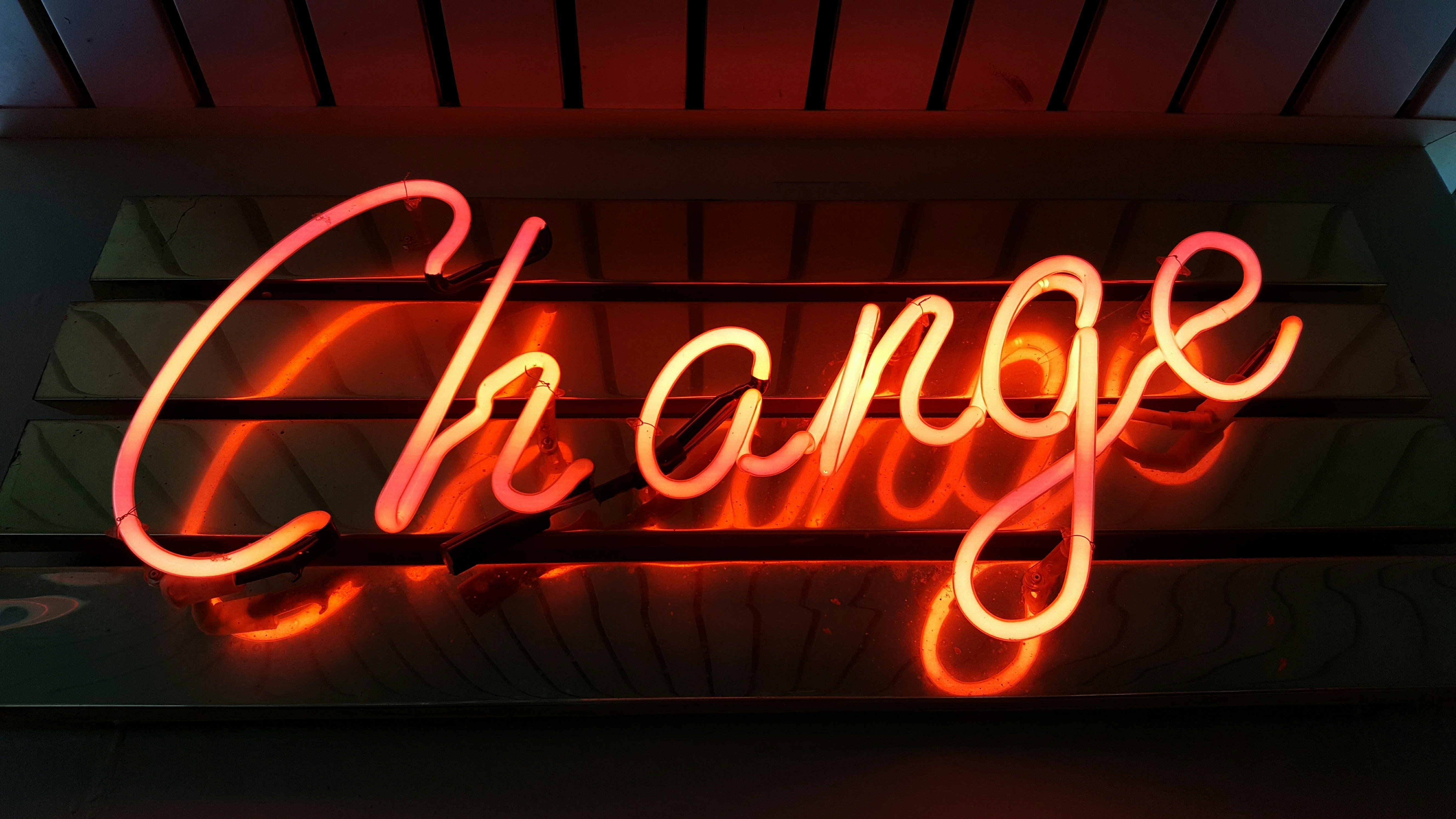
Business interruption from supply chain vulnerability is one of the most complex risks in global business environments. Climate change is a major root cause of supply chain interruption, mostly resulting from acute risks of extreme weather. However, also company-internal processes are subject to business interruption because of climate impacts. In the extended heat period in Central Europe in 2018 that caused high temperatures and low water levels e.g. in the Rhine, the global chemical manufacturer BASF had to adjust production and logistics at its Ludwigshafen headquarters. The quantitative assessment of such risks is crucial to adequately price insurance products, and also to design parametric products around business interruption.
Climate change also has material regulatory and liability implications. Since conventional emission trading schemes have been in place for several years, emissions regulation is tightening, and new targets or instruments are shaping. Germany’s recent ruling about emission taxation impacts on business risks of companies and requires strong improvement in both fact-based carbon accounting of business processes and industry-wide climate risk reporting and disclosure standards. The latter will increase exposures for directors and executives who oversee disclosing the risks.
A comprehensive framework for risk disclosure has been developed by the TCFD. For insurers and climate risk analysis experts, who work with these risks on a daily basis, it is clear that the complexity of climate risks basically eliminates the suitability of generic, industry-focused approaches. Processes differ substantially among companies within the same industrial segment. An AI fed by an underlying modern data logistics layer is best suited to account for such evolving risks in a robust and reliable manner. But how to integrate all required data from corporate legacy?
From closed data systems to an interoperable world of APIs and micro-services
In any business segment, IT and data systems are heterogeneous and hardly interoperable. This problem emerges from either a diverse landscape of applications and vendors serving different needs, or from monolithic Enterprise Resource Planning (ERP) systems that are complex in setup, cumbersome in operation and costly in maintenance and expansion. Use cases that employ AI to work with data across this system landscape are hindered by the lacking interoperability of systems, meaning it is almost impossible to consolidate and analyse data. Concretely, companies are blocked in rapid AI adoption, because they need to:
- carefully evaluate which process is suitable for ML and which data is required to deliver a working AI solution.
- be clear on the desired outcome and the best-suited algorithm and ML technique.
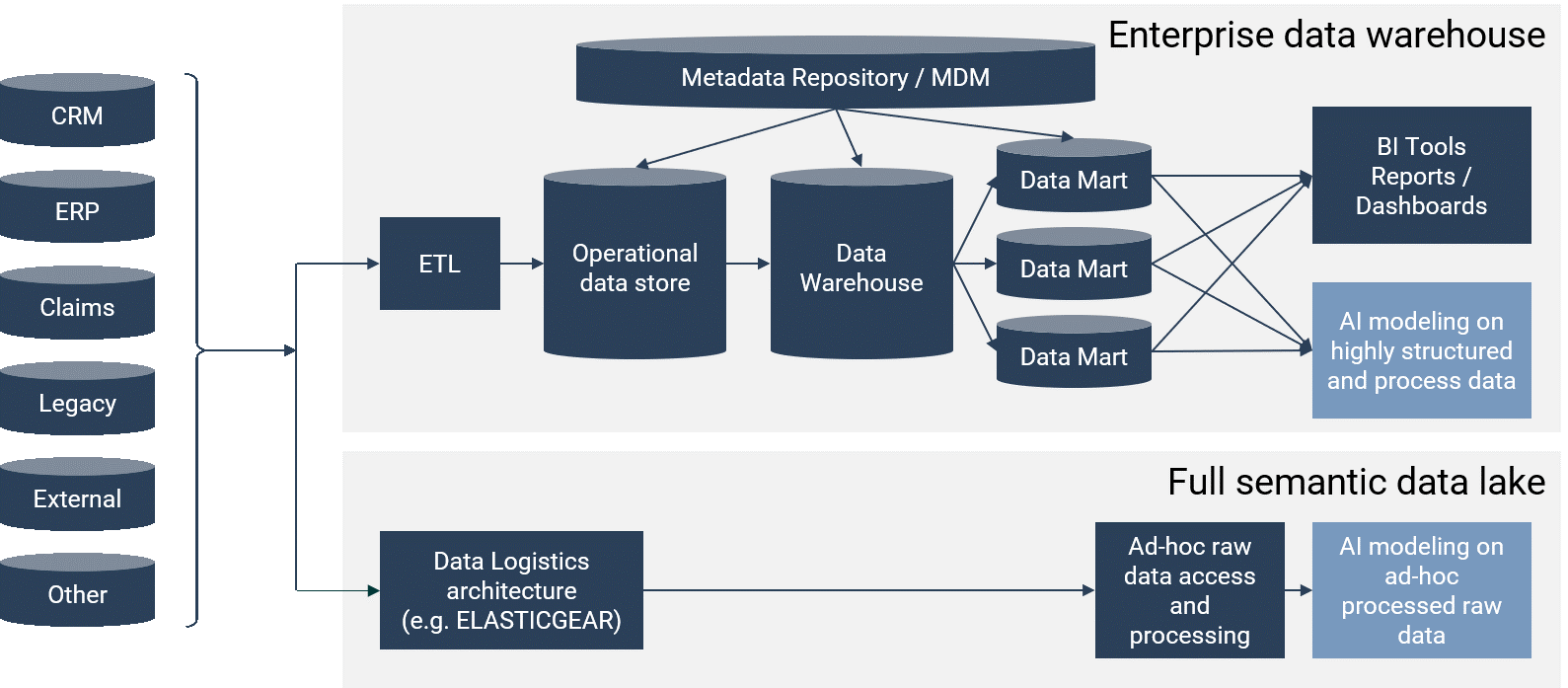
Today, typical IT architectures use data warehouses as the workhorse for data management. Data warehouses are highly organised and structured IT systems for data storage (such as indicated in the upper panel of Figure 1). Data is transformed and loaded in a predefined manner into the warehouse. Changes on the structure of the data warehouse are associated with cost and often hardly possible due to mutual dependencies of the internal sub-systems. Data warehouses are highly tuned to solve a specific set of problems but catering any new use case outside the original scope is typically hard.
The predefined structure and aggregation level of data, the complex system architecture of many interrelated databases, batch-oriented processes, and the missing interoperability to external data sources poses obstacles to the fast development of AI-supported applications. Interoperable data access would allow an AI to learn from a much richer set of data, while direct access to raw data sources without intermittent ETL processes would enable the AI to identify more fine-grained patterns in data if they exist, and to learn aggregating data by itself.
Our recommendation to resolve this dilemma is to modularise data systems. Instead of designing complex processes and systems to integrate data, companies should focus on making disparate data sources accessible, making any data point searchable and instantly retrievable, and using micro-services for dedicated applications on the data. This approach is visualised in the lower panel of Figure 1. One technology that allows for full interoperability of corporate data builds upon an orchestration and industrialisation of open software. The transition to micro-services can be performed step by step: Processes that ought to be transformed with priority are those that are of high value (either internally or towards customers or partners) or create major waste. Based on the applications used in the processes and the way to use them, targeted micro-services can be designed with APIs (interfaces) as publicly accessible as possible. Financial service companies in Switzerland started to follow these recommendations and to make databases of legacy systems API-ready.
The Internet-of-Things: ready-to-use points-of-truth for sustainability management
Modularisation of data sources also implies that valuable information from outside an organization can be integrated seamlessly via APIs. One such data source is the Internet-of-Things (IoT), i.e. objects which collect data and communicate this information over the internet. A first collection of IoT driven use cases in the financial services industry is given by Finextra. IISD describes how digital technologies come together for data acquisition, storage and curation, trust and accountability, and insight and analysis in sustainable finance. And the Sustainable Digital Finance Alliance introduces into a range of applications for IoT technology, for instance to lower the cost of validating green investments by asset monitoring.
The impact of IoT is so significant because it reaches people, objects and processes that other technologies could not or not in a similar quality. Sensors and cloud-based analytics therefor enables better informed capital planning for sustainable investments.
If you like to hear more about Data Logistics and IoT integration for climate related impact and risk analysis, listen to our recent video interview with the Swiss Re Global Institute.