Cracking open the black box: New generation machine learning models and regulatory analytics
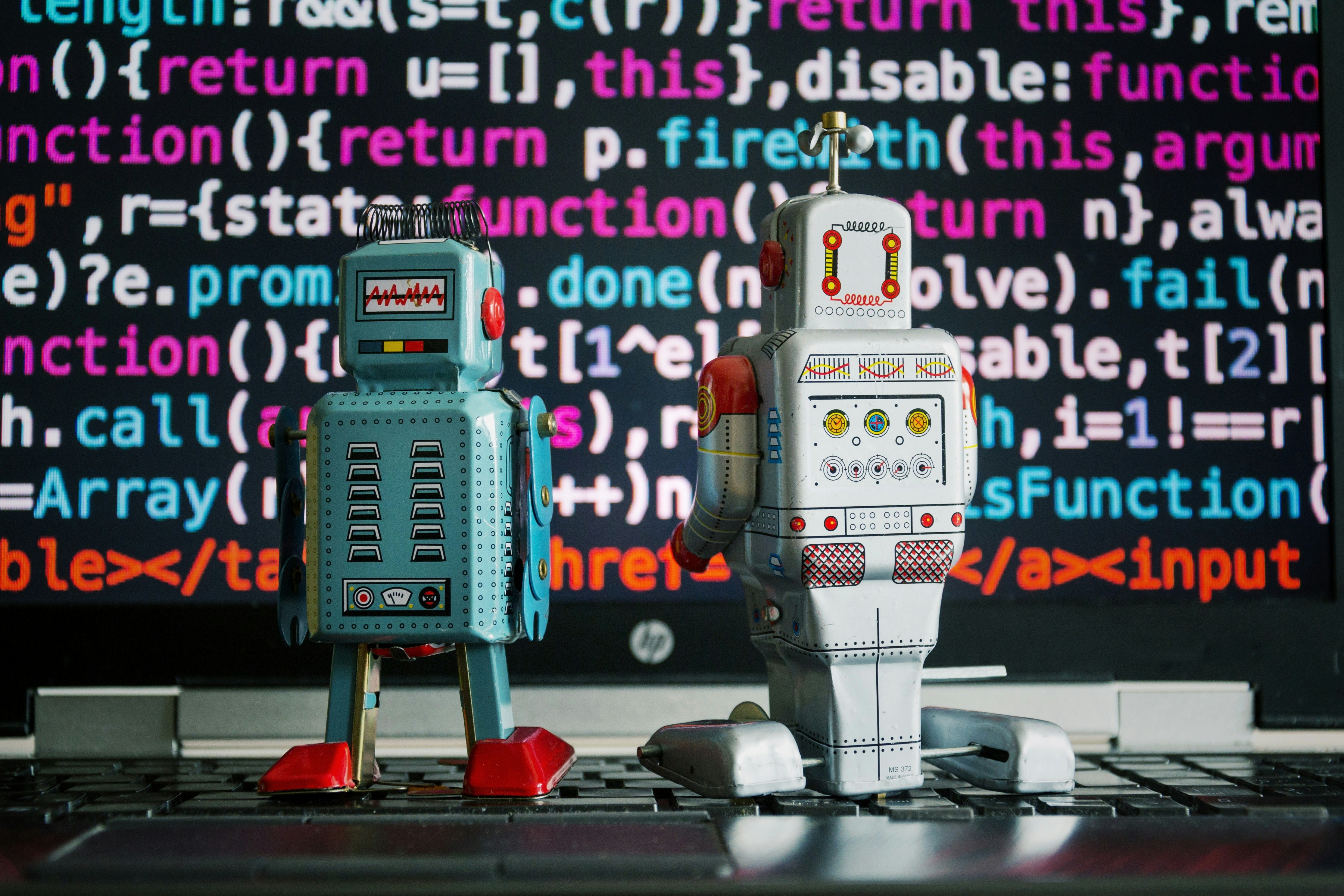
Back in May, RiskMinds International producer Vincent Beard identified machine learning (ML) as one of the top 10 concerns on the minds of the global risk management community in 2019. Machine learning was flagged as a topic “that has been on the rise for the past few years but has more interest than ever in 2019. With new case studies launched each week in a range of different risk taxonomies, where can artificial intelligence and machine learning be of most benefit?”
Enter Davide Boselli, Senior Regulatory Analytics Consultant EMEA for Experian. As a thought leader for Experian, Davide has been at the forefront of ML models and their application to regulatory analytics – one of the areas in which Experian has recently evolved platform-based solutions. His keynote for the RiskMinds International forum in Amsterdam on 3 December 2019, entitled ‘New generation machine learning models for regulatory analytics’ offers event participants an intriguing – and practical – insight into the strength (and increasing transparency) of ML for creating models that empower financial institutions.
We caught up with Davide to ask about the new generation of models in machine learning, and why ML is important for regulatory analytics.
Why do we need machine learning to effectively approach regulatory requirements?
We need machine learning because nowadays there are some very good frameworks that can help banks speed up the implementation of modelling for regulatory purposes. Also, we are able to get a lot of information from the web or from external sources today – and ML is a very useful tool to manage this better. Through this kind of information, banks can improve modelling performance and get timely answers from the modelling. We also have the capabilities to implement the model in IT systems easily nowadays.
What are the main challenges?
The main challenge is to be able to track the process that we follow using ML. This is because ML can sometimes be perceived as a ‘black box’ – impossible to look into – and from the regulatory point of view, it’s very important to be able to track the process that we follow in ML. It is important that the auditor can look into the process of the model development, to be sure it meets the appropriate requirements. The challenge is to improve this capability using ML; because ML is impenetrable and works in real time, it’s very difficult to try to recreate the process we follow in ML.
Which regulatory requirements will be addressed most directly through ML?
For financial institutions, machine learning is very useful to monitor risk – but not to develop the model to identify capital requirements, because regulators are not pleased when banks use this ‘opaque’ and untraceable ML.
However, for early warning systems, to prevent the flow of NPL, and to better manage portfolios, ML is a very suitable tool. This is especially true because today we can collect data from the web, and embed that into early warning systems using ML.
In the past, we looked at historical information in order to forecast the future. Now we want historical inference. Yes, we want to learn from the past to learn about the future – but with ML we also want to use the up-to-date information that arises every day, in order to expand upon the historical information we’ve been using, while improving the old framework.
This is in real time – it’s a framework for algorithms – and because of that, we can recognise in a very timely fashion who will not be a good client in the future.
What is Experian doing to make this approach more suitable for regulatory purposes?
At Experian, we are working to crack open the black box, particularly to break down certain aspects within ML. One is to try to make the process leading to the development more explainable. We want to track each step of developing the model. Second, we want to improve and build a new monitoring framework using ML to monitor the model. And last but not least, we are developing a report that will allow the supervisor/auditor to see the process that we are following for developing the ML model while we are figuring out how to embed ML in the old process. We can apply ML to only one variable in the development process; or we can use ML end-to-end, where we start from data manipulation to implementation, and go through the indicators, the statistical framework and so on, ending up with a proper model.
And the last thing that is very useful today is to challenge the internal model with the model developed with ML. That way we can compare the internal model and old framework using ML and identify areas of improvement. This is one of the best things we can do with ML currently. The benchmark is the requirement that every supervisor and regulatory body wants the banks to have in place – and ML could be the champion model with which to compare internal models.
What should financial institutions be thinking about with regard to integrating ML in their processes?
Risk management is not just regulation, but it also the means to better manage the bank’s risk profile by reducing loss and increasing profitability. Everyone wants to be able to handle ML because it’s the latest, hottest thing – this has happened with other technical innovations in the past. Now they want to crack open the black box and know what’s inside, and be able to pick out the process that is being followed in order to satisfy regulators.
It’s very important that financial institutions start to think about on which kind of platform they want to implement next generation ML models. This is a very important step forward to try to standardise the platform for risk and regulatory models.
Meet Davide Boselli at RiskMinds International.
About Davide Boselli:
Davide Boselli is Senior Regulatory Analytics Consultant EMEA for Experian. He has more than 15 years of experience in risk management (with such organisations as Banca Intesa, UniCredit, Prometeia and CRIF) in the areas of credit risk modelling, rating system validation, regulatory compliance documentation, IT architecture to support internal rating systems and regulatory advisory and credit processes.