Guiding AI in plain language summaries for clinical trial results
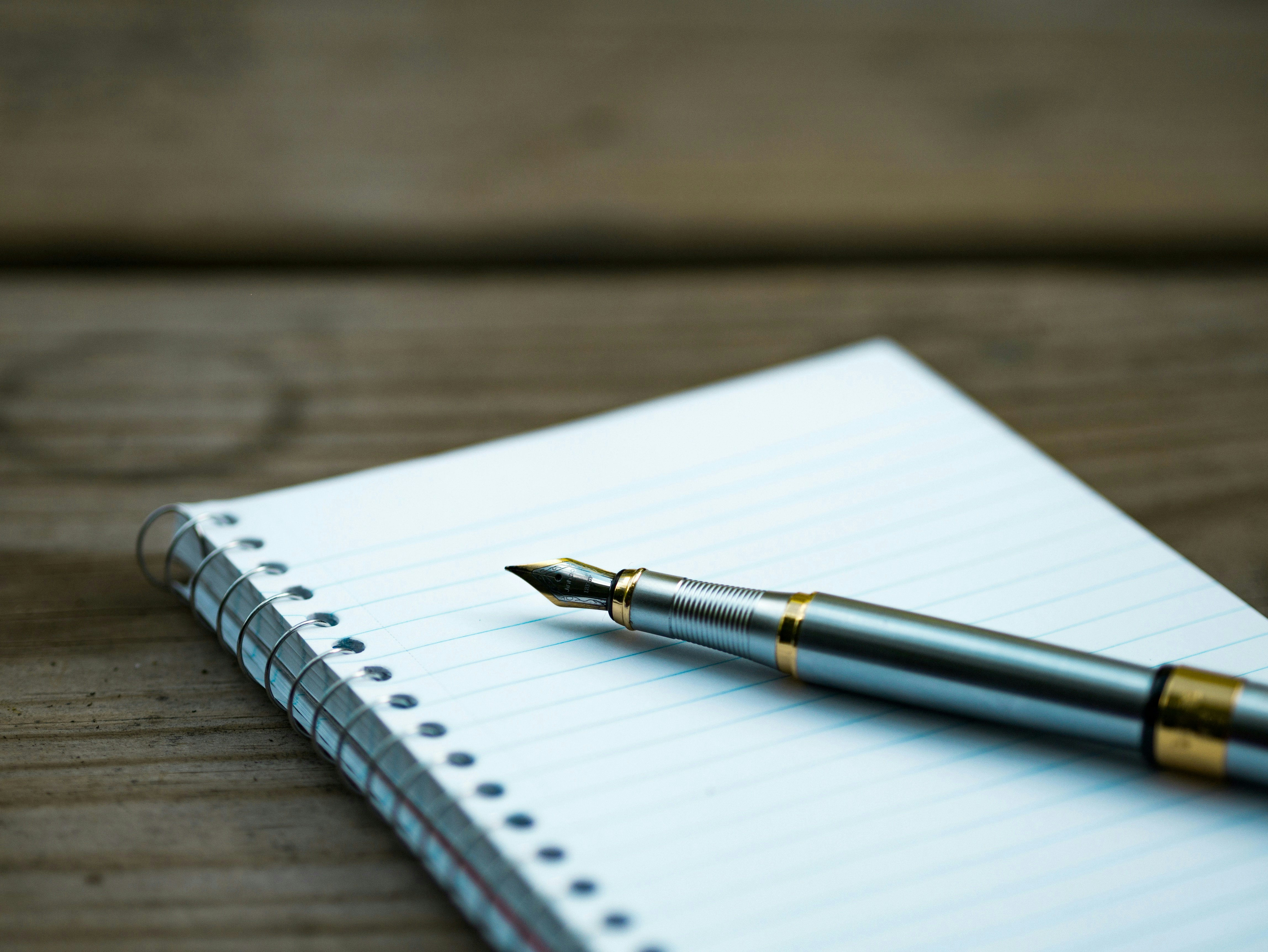
A multistakeholder working group has developed a draft considerations document on the responsible use of artificial intelligence (AI) in creating plain language summaries (PLS) for clinical trial results.
In a recent webinar presented by CISCRP, a nonprofit organization that educates patients, professionals, and the public on clinical research, representatives from the multistakeholder working group shared the goal for the initiative and discussed key considerations from the document.
Moderating the panel discussion was Ken Getz, founder of CISCRP, and professor at the Tufts University School of Medicine. Panelists included Sudipta Chakraborty, Clinical Trial Transparency Lead, Biogen; Julie Holtzople, Independent Consultant, Clinical Trial Transparency Expert; and Malgorzata Sztolsztener, Director, Clinical Trial Transparency Patient Engagement, AstraZeneca.
According to Getz, the introduction of AI and clinical research has been both “rapid and dramatic.”
“We are seeing a large number of AI applications, most notably in routine labor and time intensive activities, being deployed and used within clinical research,” he said.
Just recently, the Trump administration announced a $500 billion investment in AI infrastructure in the US. “All of these kinds of investments no doubt accelerate AI and generative AI use and applications,” he said.
Sztolsztener said when the industry began witnessing the widespread use of AI to create PLS without human oversight, it raised valid concerns over data integrity, errors in overall writing style, and the usability for the intended audience.
“This group was established as a response to the challenges faced by the industry in late 2023, beginning of 2024,” she said.
“The main focus of this group was to build a document that would provide guidelines and elements for considerations on how to use AI in a responsible way, how to integrate it in the framework in a responsible manner and making it a useful technology that would assess human and not substitute them,” Sztolsztener continued.
The multistakeholder working group spent the past year defining its scope, establishing a clear charter, and researching the landscape of the PLS, Holtzople said. They also ensured the group included experts with experience in AI-driven PLS development.
The document, “Considerations for the use of Artificial Intelligence in the Creation of Lay Summaries of Clinical Trial Results,” is open for public comment until February 18.
Considerations
The panelists shared core considerations that are included in the document for comment.
One of the key elements is the essential need for researcher involvement in the process of creating PLS results, according to Sztolsztener.
And that’s because “sponsor subject matter experts not only own the data, but also have a deep understanding of objectives, design, endpoints, and all of the statistical analysis and scientific nuances that come into play,” she said.
Experts’ insights into the complexity of the trial enable them to align PLS with scientific nuances of the trial and address the needs of patients, she said.
Sztolsztener emphasized that sponsor engagement ensures “accurate interpretation of complex technical details and data and facilitates the creation of summaries in an understandable manner, while at the same time - which is also of huge importance - maintaining scientific accuracy.”
On the other hand, Chakraborty stressed the importance of human involvement while noting the concerns about AI potentially replacing jobs, but not in the case for PLS. As an example, she suggested that AI could be valuable in generating an initial draft of a PLS document. However, the process gets more complex when stakeholders begin reviewing, commenting, and incorporating revisions.
“That’s really where humans have to step back into play in the process,” Chakraborty said. “AI has to complement your current PLS process and not necessarily take over current steps.”
From a patient-centricity perspective, Sztolsztener also suggests preserving existing best practices that involve patient input in the PLS creation process.
“That feedback is so critical because AI alone can’t give those perspectives to us,” she said.
Another consideration that Holtzople touched on is data privacy.
“We have to make sure that during this process it’s designed to respect data privacy and that includes both the outputs as well as the inputs,” she said.
Furthermore, Holtzople added that it’s crucial that the development of the AI tool and its underlying models prioritizes data privacy and doesn’t store any individual patient-level data.
AI disclosure
Regarding transparency, it’s also important to disclose how AI is used, according to Chakraborty.
“I think a lot of the mistrust or the skepticism that is out there right now comes from the fact that people don’t really understand AI yet, but they also are worried that it’s being used in ways that they don’t really know in their day-to-day lives,” she said.
She noted that the considerations document includes disclosure statement examples of best practices for when, where, and how AI should be disclosed.
Sztolsztener, who agreed with Chakraborty, said transparency is needed when it comes to technology being used as well as building trust, noting that these summaries are legally required in the EU. She added that PLS are consistently listed in patient surveys as key information patients would like to receive or are interested in.
“These are the key elements that will help us build trust and confidence in the pharmaceutical industry and show that we are not choosing the easy way, but we are really committed to providing patients with accurate information. It’s our aim to keep them informed and engaged,” she said.
Hear more from Julie Holtzople and Sudipta Chakraborty at our upcoming conference.
Quotes have been lightly edited for clarity.
Unsplash/Aaron Burden