Harnessing the power of hybrid intelligence for enhanced investment decisions
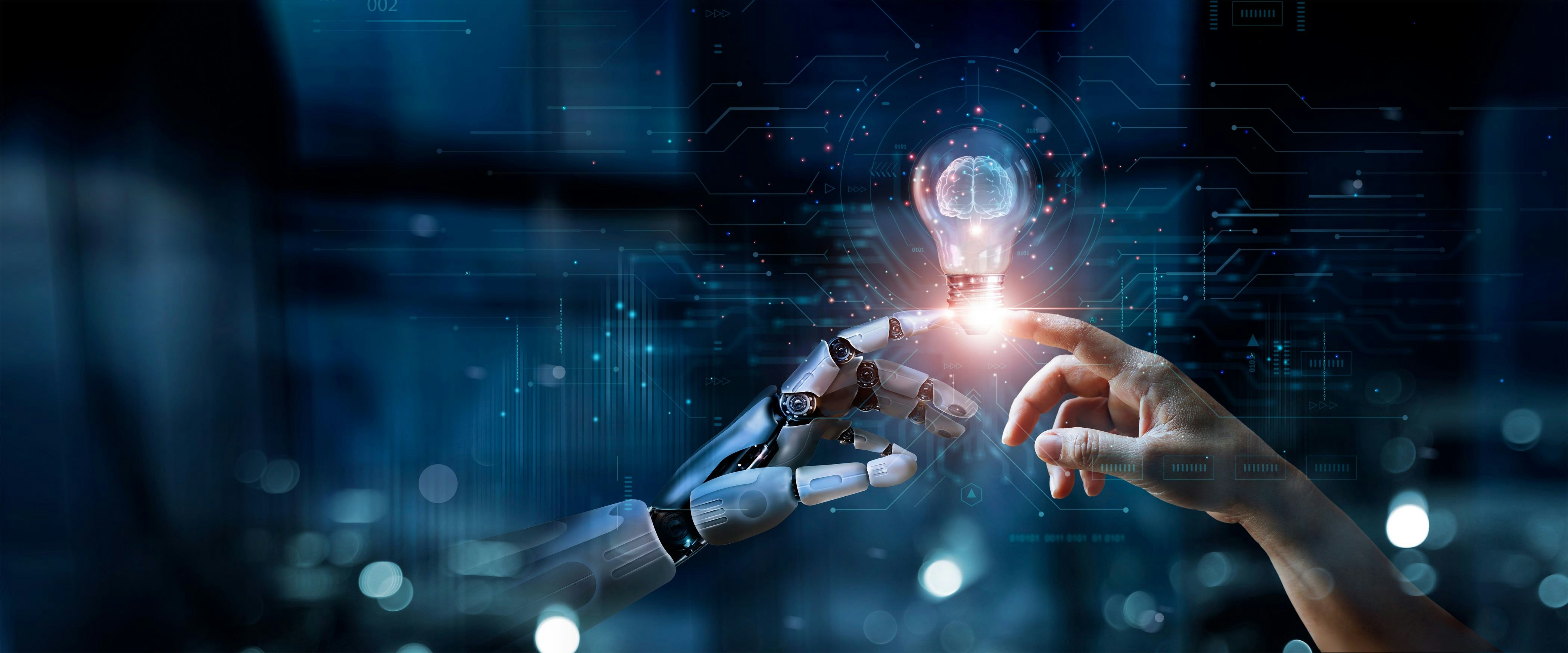
The benefits of hybrid intelligence
Decision-making, especially in uncertain situations, is at the heart of the investment process. But investment managers, like most of the general population, often use mental shortcuts to make many important decisions, and those shortcuts can create what are called cognitive biases.
Many types of cognitive biases can impact decision making by institutional investors, including Recency Bias, Confirmation Bias, Anchoring Bias, Overconfidence Bias, and Incentive Bias. These biases in turn can result in less-than-optimal outcomes. Hence the draw of Artificial Intelligence to support human decision-makers isn’t surprising.
When investment firm users embrace the combination of human and machine intelligence, or hybrid intelligence, the business benefits include enhanced risk management and more profitable investment decision-making, among others. Hybrid intelligence allows firms to leverage both human experience and rapid, predictive AI analysis.
Heuristics and how we make decisions
Heuristics are mental shortcuts that allow us to solve problems and make judgments quickly and efficiently. But while they can aid and expedite the decision-making process, they can also have some drawbacks, namely cognitive bias, which can take several forms.
Recency Bias can lead us to place more importance on recent events at the expense of historical events. For instance, in order-driven markets, traders can execute a trade immediately at the best available price by submitting market orders, or they can submit limit orders to execute a trade only at a more favourable price. In this situation, traders are often inclined to make this decision (market order vs. limit order) based on recency bias — recent trade performance, news, or information — as opposed to all the relevant information associated with the trade, including previous performance.
Confirmation Bias is when we look for — and notice — evidence that confirms our existing beliefs. Investors often ignore negative information about buying or selling securities, which if considered, could serve as a warning sign to prevent losses. They can also miss out on otherwise attractive and profitable opportunities by ignoring data that supports a point of view that differs from their own.
Anchoring Bias is the tendency to rely too heavily on the first piece of information we learn. For example, an investor may estimate next year’s earnings for a particular company to be $2 per share. However, when the company experiences difficulties during the year, the investor may not adjust their benchmarks to account for these challenges because they are anchored by the original view.
Overconfidence Bias occurs when we overestimate our own abilities. Investment managers tend to underestimate the risks and overestimate expected returns on investments they feel strongly about. Traders may be quick to sell an asset that has disappointed them, only to buy a new security that they feel overconfident about.
Incentive Bias is the power of incentives (both punishments and rewards) to impact our behavior. We are incentivised to believe the things we want to be true. Take the case of Archegos Capital’s collapse in March 2021, where the focus on generating short-term profits for the firm led to people underestimated the counterparty risks involved in the transactions.
Applying machine learning to decision-making: Building trust
So how can investment firms encourage greater adoption of AI models? Explainability is key. Explainability involves transparently showing how an AI or ML model gets its outputs from specific inputs. When models are explainable, users understand, trust, and incorporate them.
One of the most effective ways to establish user trust in an AI/ML model or data analytics service is to involve users or domain experts in its design. A cross-functional team of domain experts and data scientists must ensure the data used provides a relevant output that is understandable and actionable.
In addition to involving end users in a model’s design, AI and ML teams should constantly be adjusting the model. It’s not a “set-it-and-forget-it” creation. Regular model evaluation via pre-defined metrics is a must to ensure the models stay up to date. Various visual tools are available to do historical assessment along with “what-if” analysis to ensure any drifts are kept in check.
Leveraging hybrid intelligence in investment operations
Getting users to embrace and incorporate AI models into operational decision-making is an important goal for investment firms. But that shouldn’t be misconstrued as “let the algorithm make all the decisions”. AI is at its best when it’s used within the framework of hybrid intelligence —the combination of computer-based, data-backed input and human insights.
At Linedata, we’re building ethical design and sound model governance into our AI and ML solutions, utilising hybrid intelligence to improve operational investment processes. Transparency and explainability are core elements of our predictive analytics framework as we find ways to ease understanding, build in transparency, and remove the “black box effect” around insights and how they can be applied to improve operational outcomes that otherwise might be limited by human bias.
This blogpost is excerpted from the recent white paper “Harnessing Human + Machine Intelligence in Capital Markets: How to Overcome Cognitive Bias to Improve Decision Making” by Ashmita Gupta. Ashmita heads Linedata’s Business Intelligence & Analytics Service offering. She is passionate about helping clients solve operational problems and brings 14+ years of operational experience with hedge funds and investment managers along with her Data Science expertise.
Click here to download the full white paper. To learn more about Linedata Analytics Service, visit their website.
Linedata is a silver sponsor of IMpower 2023. Find out more information about the event here >>