Implement and operationalize AI/ML models to optimize and accelerate credit decisions
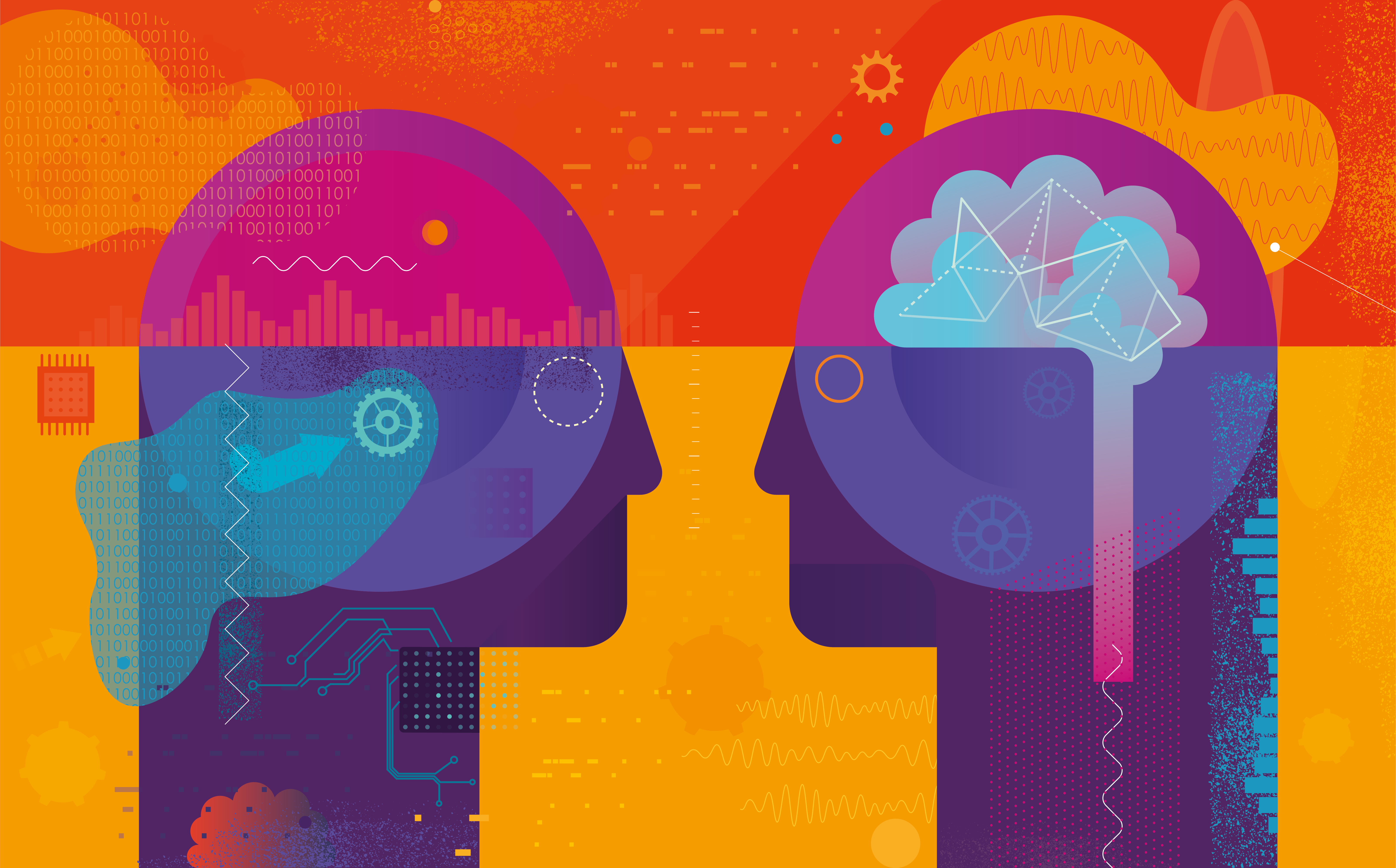
One of the game changers to stay ahead of competitors is believed to be artificial intelligence (AI) and machine learning (ML). Yet, many financial institutions (FIs) are not able to benefit from the full potential. Many institutions are still struggling in their adoption to operationalize AI/ML in automated business processes. This is largely attributed to the fact that FIs are required to close the threshold between model development and integration into operational decision-making processes.
AI-powered decisions across the lending life-cycle
Nearly every aspect of financial services is transformed by emerging technologies, and the pandemic has only further accelerated ambitions relating to the implementation of new technologies, amongst them AI. In its broadest sense, AI is most likely used in some form at the majority of FIs.
There are numerous use cases where machine learning models can be integrated in the lending life-cycle of a customer. However, financial institutions need to prioritize where AI capabilities add the most value and should complement or replace rule-based decisions. Many of the decisions best suited to AI improvement are operational decisions: high volume, transactional decisions.
The use of machine learning within the lending life-cycle has the potential to increase value in various ways:
- Improved customer acquisition: Highly personalized and needs-based customer communication in digital banking in real-time across all channels and faster response rates create superior customer experiences and increase conversion rates.
- Reduced operating costs: Banks can lower their costs by intelligently automating back-office processes and decision making as much as possible, especially when acquiring and servicing customers.
- Improve customer loyalty and retention: Demand-oriented information for customers about service offers and continuous and intelligent engagement with customers strengthens the relationship across multiple products and services.
- Lower credit risk: To reduce credit risk, banks can adopt more sophisticated screening of potential customers and early detection of behaviors (anomaly detection) that signal a higher risk of default or fraud.
What are the most common adoption barriers for AI technologies?
When companies are asked what the biggest challenges are in putting the theoretical groundwork and models they have developed into practice, a study by Gartner* identified a number of different barriers.
Apart from the obligatory security and data protection concerns, it is the complexity of integrating the AI solution into the existing IT infrastructure, as well as the perceived difficult use and deployment of AI technology.
If financial institutions do not manage to close the threshold between model development and integration into operational decision-making processes, AI experiments in many companies will remain just that: an experiment.
Hence, the crucial question is: How can a financial institution integrate and operationalize the developed (AI/ML) decision models – with as little effort as possible and in a short time – without failing due to the barriers described above and thus jeopardizing the investments already made?
Digital Decisioning Platforms: The perfect combination of human and artificial intelligence
One of the most persistently adopted technologies for delivering AI is achieved by introducing a platform that integrates decision logic (aka business rules platform) and AI-based insights, or so called Digital Decisioning Platforms. Thereby institutions can reduce manual efforts and streamline business processes for better decision-making.
Digital Decision Platforms allow to automate high-volume, transactional, and operational business decisions. Consistent and accurate decisions can be made in real-time which allows FIs to quickly adapt to market changes, provide personalized offers and pricing, reduce regulatory compliance costs and help prevent fraud. Rule sets can be adapted and operationalized without programming by specialist departments (e.g. risk methodology teams) providing companies with agility and control.
Further, such platforms support bridging the gap between two sometimes ‘incompatible’ worlds: data scientists having developed (AI/ML) models with tools and languages such as Python, R, etc. on the one hand, whilst having core banking and workflow systems (e.g. in Java) on the other. Modern decisioning platforms enable the integration of AI/ ML and deterministic rule-based models, thereby achieving better and explainable, predictive business decisions. Explanations of ML decisions to humans are also provided in understandable terms.
To sum up, modern Digital Decision Platforms enables credit institutions to exert control over every decision being made, conform with regulations, and integrate AI and ML algorithms.
*Gartner 2019, AI in Organizations CTMKT_1161674