How to reduce a domino effect: modelling interconnections
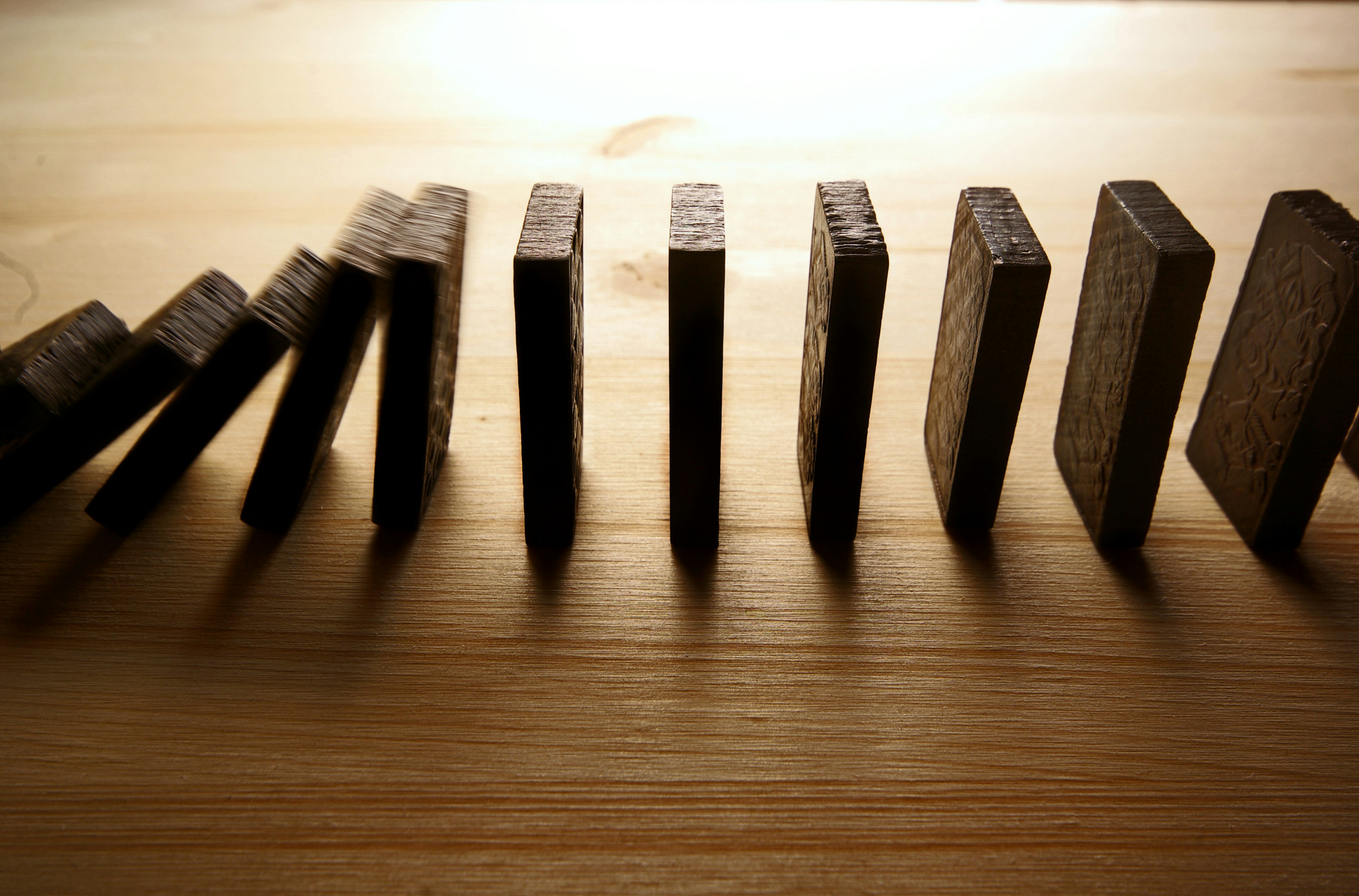
Managing risk requires an understanding of how multiple factors interconnect to influence a larger picture; assessing your models interconnectendness and identifying weakness can be even trickier. Julia Litvinova, Head of Model Validation and Analytics and Nikhil Dighe, Vice President (Quant) at State Street explore the best techniques for this. Julia will be presenting at RiskMinds Americas on analyzing the different relationships between models to eliminate inconsistency and uncertainty.
One of the questions occupying minds of model risk managers nowadays is how to assess model risk arising from known interconnectedness among models. Part of the heightened interest in model interconnectedness is that regulators would like banks to understand better how model risk is affected by interaction and dependencies among models. But setting the regulatory feedback aside, model risk managers also would like to know whether reliance on common assumptions, data, methodologies or other common factors could adversely affect several models and their output at the same time. For example, if several models rely on the data stored in a shared database, which turned out to have a data reliability and accuracy issue, model risk managers would like to be able to quickly evaluate the downstream models affected by the database issue.
Following initial supervisory guidelines (e.g., SR 11-7 and subsequently others) from regulators, firms in general have done a good job assessing and quantifying risk of individual models. However, when one considers an ecosystem of models as is typically the case, understanding the systemic risk presented by these models becomes more challenging. Sequence of models have knock-on effects on downstream models. To assess the impact of any given model on this model ecosystem requires analysis of large group of models together. We chose to approach this problem using a network-wide modeling approach. Similar network-wide modeling approaches have been long used in variety of disciplines like Operations Research and more recently in analysis of social networks. Google’s earliest algorithm to assess the relative importance of a webpage based on other webpages referencing it, is based on such network modeling approaches.
Model risk managers would like to know whether reliance on common assumptions, data and methodologies could adversely affect several models and their output at the same time.
Conceptually, one would like to somehow assess the risk imposed by every model on the entire network. Once we can quantify this systemic risk, we can identify models posing the highest systemic risk. Such an insight is invaluable as these models can be focus of additional review given their importance. It can be the case that in isolation these models themselves are deemed to be low risk. However, they might pose high systemic risk to the model ecosystem. Any approach to assess systemic risk of interconnected models should be able to capture this. Such a consideration was critical in our approach. Model owner might not be aware of the high systemic risk imposed by his or her model or the extent of its impact downstream. Performing a network-wide analysis allows proper identification and appropriate risk mitigation.
In our network topology, models are represented as nodes and directed links represent the interconnections of models. In our terminology, for a model X, upstream models are models feeding data into model X, and downstream models are models which receive outputs from model X. Directed links capture the direction of flow of information. Some models exhibit inherently higher model risk than others. We incorporated this information via individual model risk scores resulting from model validations. An easy first step would be to assess the number of direct connections (downstream, upstream or both) to assess the relative importance of a model. However, this accounts only for the direct impact and not the indirect impact. Our approach accounts for all possible paths in which any given model can impact other models. This has the added benefit of identifying hard to detect sources of systemic model risk.
We assessed our network of models in two ways identifying important upstream and downstream models in our model ecosystem. The foremost criterion is naturally the impact of a model on downstream models, which allows the capture of domino effect. Since model output from upstream models get sequentially used and transformed in downstream model, this important criterion identifies key systemically important upstream models with high downstream impact. Another informative criterion can be key downstream models where either information aggregation from upstream models happens, or information from important upstream models is processed. Even though such models may not impose high risk to other models downstream, they can play an important part since they are closer to ultimate model outputs from our model ecosystem.
Assessing the risk posed by interconnected models is challenging and is getting more attention.
We have applied our framework of assessing systemic model risk to the network of models used for Federal Reserve Board's Comprehensive Capital Analysis and Review (CCAR). This network of models is a good training set for our approach given its limited linkages to models used outside of CCAR. In other words, CCAR models form a closed ecosystem allowing us to apply our approach in a clean and unbiased manner. After an important step of collecting the linkages between all CCAR models, we performed network-wide analysis of all CCAR models and the outcome was in line with our intuition. The most systemically important feeder model was a scenario-generating model, which provides inputs into majority of CCAR models. An important downstream model was the aggregating utility which processes outputs from other upstream models.
Our approach and similar network approaches can be used to identify all downstream models affected by model issues in any model or sensitivities to certain factors. This approach can be further extended by quantifying the impact provided data on sensitivity of model inputs on model output is available. Assessing the risk posed by interconnected models is challenging and is getting more attention nowadays.