AI in Biotech: Designing the Needle Instead of Searching the Haystack
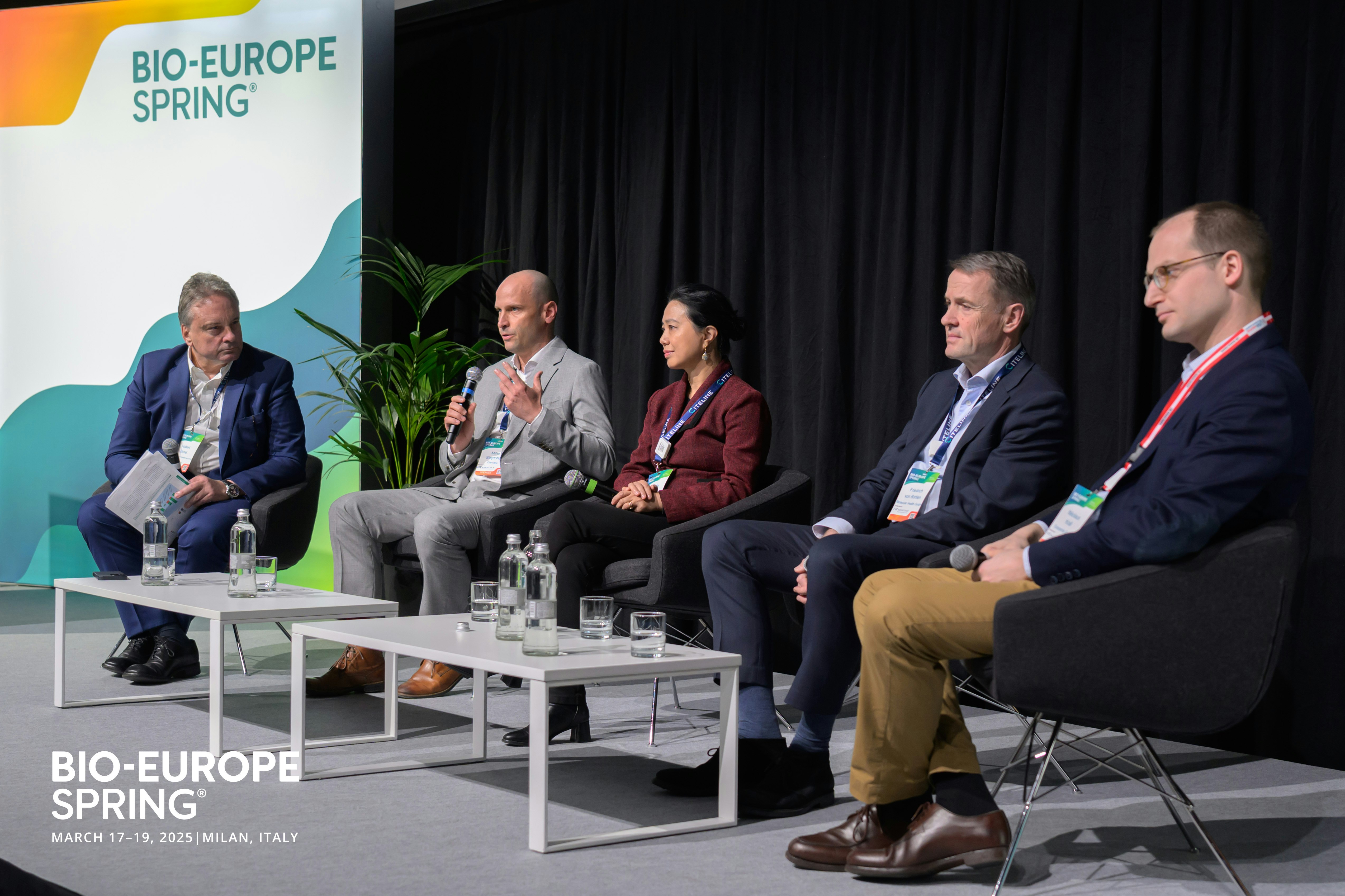
Artificial intelligence (AI) is no longer just a buzzword in the biotech and pharmaceutical industries. With the potential to transform drug discovery and development, AI is driving a revolutionary shift in the field. At BIO-Europe Spring in Milan, a panel of industry experts explored the current and future role of AI in biotech and pharma, discussing how it enhances R&D economics, tackles integration challenges, and reshapes drug development.
AI’s Role in Drug Discovery and Target Validation
Moderator Hubert Birner, Managing Partner at TVM Capital Life Sciences, set the stage by emphasizing AI’s growing prominence in drug discovery. He pointed out that AI is a vital tool, and that biotech and pharma companies must take a practical approach to its adoption. “AI is not a magic bullet; rather, it must be integrated thoughtfully into existing drug discovery processes to yield meaningful results,” Birner said. He also stressed that while AI can improve output of stage early-stage research and development, its true test will be whether it improves success rates in later clinical development.
Nikolaus Krall, Managing Director at Exscientia, highlighted that AI, although in relatively early development, is already making significant strides in target discovery and validation. According to Krall, “AI models can now analyze vast datasets, including single-cell sequencing, to pinpoint promising drug targets.”
Michelle Chen, CBO of Insilico Medicine, expanded on AI-driven small molecule discovery. She explained that Insilico’s generative AI models create novel molecular structures with desired properties, significantly reducing the need for traditional high-throughput screening. She compared the approach to “designing needles instead of searching for them in a haystack.” The result? Faster drug development with a higher probability of success.
From Drug Design to Clinical Trials
Already, AI has demonstrated the ability to identify potential drug candidates. However, there is even more potential to unlock. Friedrich von Bohlen, CEO of Molecular Health, emphasized the importance of understanding molecular causality in diseases. Molecular Health has built a comprehensive data system that integrates information on drugs, diseases, and molecular interactions to understand and improve drug safety and efficacy predictions. Notably, the company’s AI-driven analytics are used by the FDA to assess drug side effects, demonstrating AI’s critical role in regulatory decision-making.
Beyond safety assessments, von Bohlen highlighted how AI is being used to plan and predict clinical trial success. “By analyzing historical trial data and molecular interactions, AI models can provide early indicators of which drug candidates are most likely to succeed in later-stage trials,” von Bohlen said. This predictive capability could help biotech and pharma companies allocate resources more effectively and avoid costly late-stage failures.
AI’s influence extends beyond drug discovery to clinical trials. Miha Stajdohar, CTO and Co-Founder of Genialis, discussed how AI-powered biomarker discovery can help identify the right patient populations for clinical trials. Stajdohar explained, “This targeted approach enhances the likelihood of trial success while reducing costs.” He envisions a future where AI-generated “digital patients” simulate different treatment outcomes, potentially reducing the size and duration of clinical trials.
Overcoming Challenges: Data, Talent, and Adoption
Alongside this promise, AI adoption in biotech and pharma is not without challenges. Data quality remains a key hurdle. Chen stressed that AI models require both positive and negative data to effectively refine predictions. “Access to high-quality patient data, particularly from electronic health records, is critical but often restricted due to regulatory and privacy concerns.”
Another challenge is the need for cross-trained talent. Many AI-driven biotech firms struggle to find professionals who possess expertise in both machine learning and drug development. Chen emphasized the importance of fostering interdisciplinary training to bridge this gap.
The Future of AI in Biotech
As AI continues to mature, experts believe its impact will grow exponentially. Krall predicts that AI will eventually bridge the gap between preclinical and clinical research, “significantly improving drug success rates.” Stajdohar envisions a future where AI-powered multimodal models integrate diverse datasets—genomics, transcriptomics, and patient phenotypes — to create a comprehensive understanding of disease mechanisms.
For biotech entrepreneurs looking to leverage AI, the panelists offered valuable advice. The key takeaway, start integrating AI early! Whether through AI-driven drug discovery platforms or clinical trial optimization tools, companies that harness AI strategically can reduce costs, accelerate development, and improve success rates.
AI in biotech and pharma is no longer just a futuristic vision—it’s happening now. While challenges remain, the panelists agreed that AI’s ability to streamline drug development, personalize medicine, and enhance clinical trial efficiency makes it one of the most valuable assets for the future of life sciences.