AI: The next great augmentation tool
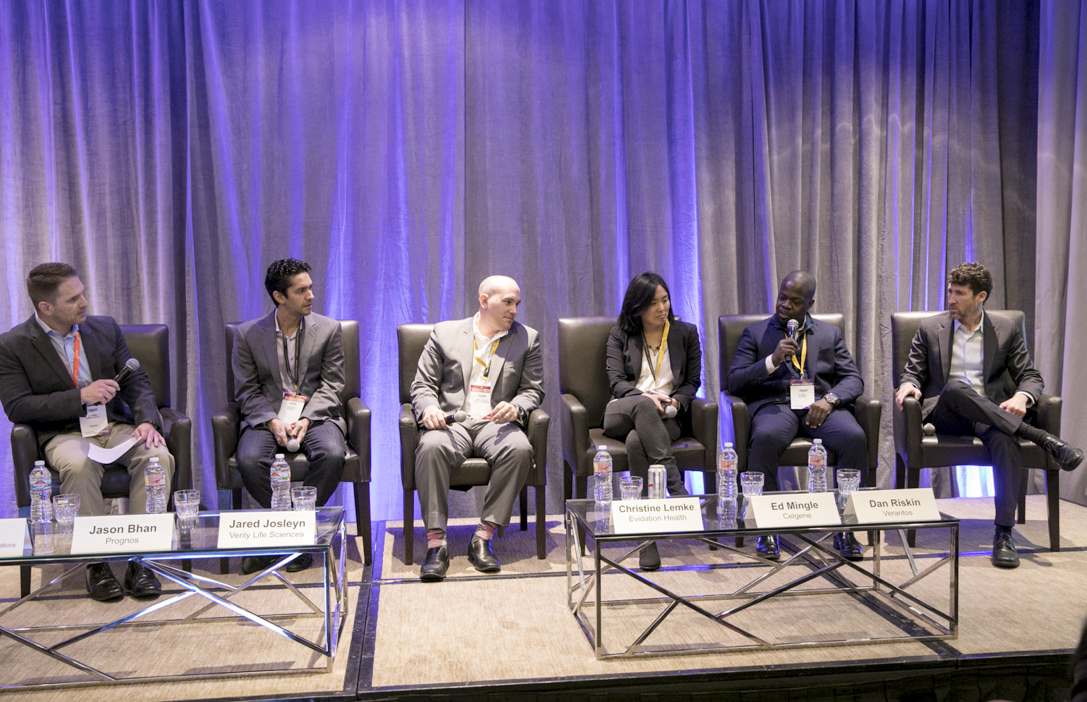
AI isn’t a destination. People don’t entirely trust it, but it is here and it can deliver access to data points that previously were inaccessible, catalyzing new knowledge and potentially better health outcomes, according to panelists at the Digital Medicine & Medtech Showcase luncheon panel moderated by AJ Triano, senior VP at Syneos Health, January 9 in San Francisco.
They’re not the only ones who think this. IT analyst Gartner predicts AI will reach mainstream use within two to five years. Frost predicts it will be a USD 6.6 billion market by 2021, and healthcare is AI’s hottest market. The reason is twofold: Big data analytics are common, and the sprawling healthcare ecosystem is plagued by rising costs, a deluge of data, and little means to makes sense of it.
AI—artificial intelligence—is more and less than its name implies. It is a collection of technologies that includes machine learning, natural language analysis and generation, and pattern recognition among a growing list of related technologies and capabilities. It even includes new programming languages that introduce probability into the outcomes.
The core issue isn’t about amassing huge quantities of data, but about accessing data that was almost unusable before. “AI was developed to find meaning within big data,” emphasizes Dan Riskin, CEO, Verantos.
AI engines are nuanced
For biotech companies, therefore, incorporating AI into analyses is a nuanced exercise based on deploying the right AI engine for a particular job and finding people who understand its capabilities and limitations.
“We may go with TensorFlow (an AI accelerator) for classification of diabetic retinopathy, but use causal inference when influencing care decision,” elaborates Jared Josleyn, head of corporate development, Verily Life Sciences (the science arm of Alphabet and a sister company to Google). “Understanding the nuance of the engines is important. Otherwise you may partner in the wrong way and think AI doesn’t work.”
Are AI engines trustworthy?
Before that can happen routinely, users must be able to trust AI. One of the big questions is, “How did it arrive at that conclusion?” The answer may be that it used machine learning and 50 layers of complexity to reach conclusions from a retinal scan to discern information far beyond a patient’s retinal health. The problem, from a provider’s perspective, is that they can’t always ask that question and get an clear answer.
Error rates exceeding 50% in electronic medical records (EHRs) and claims data pose another challenge. Before these data sets can be used for reimbursements or clinical decision-making, for instance, the industry needs ways to validate the data. Verantos does that by looking for additional telltales. “In Parkinson’s disease, for example, we use AI to connect the concepts and look for tremors,” and other symptoms, Riskin says.
At Celgene, “we are focused on pharmacovigilance and safety, so we look for imbalances in the reporting (from local data sets as well as clinical trials and regulatory bodies),” Ed Mingle, executive director, global safety operations, says. “We incorporate safety and pharmacovigilance in operations as well as medical and scientific concerns to make decisions quicker. Manual ways are basically broken.”
At Prognos, “we’re trying to use AI to find treatment failures and new starts and switches,” to help predict those events in the future,” Jason Bhan, co-founder and chief medical officer, Prognos, says. Presumably, payers and providers will use that information to change patient behaviors.
Real-world evidence proliferates
Trillions of data points are collected about people each year by fitness trackers, which could be harnessed to provide a vivid snapshot of everyday life that can be correlated to reactions to specific therapies. “There are such dense quantities of data per person that physicians can conduct ‘N of 1’ trials,” says Christine Lemke, president and co-founder of Evidation Health. The challenge is to remove the noise from the data.
“We’d like to see real world evidence augment clinical trial data (and inform clinical trial design),” Josleyn says. “Today, one patient produces 12 Tb of data per year, but we collect only 5 Gb of data, 4.5 Gb of which are medical images. That’s the equivalent of 7 CDs of information. In the future, data for one patient, gathered from multiple sources, will be the equivalent of 36,000 CDs. This is a massive challenge—there’s not enough space to store it—but also a massive opportunity.”
Combining molecular and physiological data, eventually, will allow physicians to objectively quantify pain based on movement, electronic signals, tests, and other data sources, panelists suggest. The resulting therapeutics—not just drugs, but all types of beneficial interventions—could augment the value of a particular drug. At its core, “AI is an augmentation tool.”
Want to read more highlights? Click here to read more all of our Biotech Showcase coverage!