‘Data Matters Most’ in Biotech, and AI is Here To Help
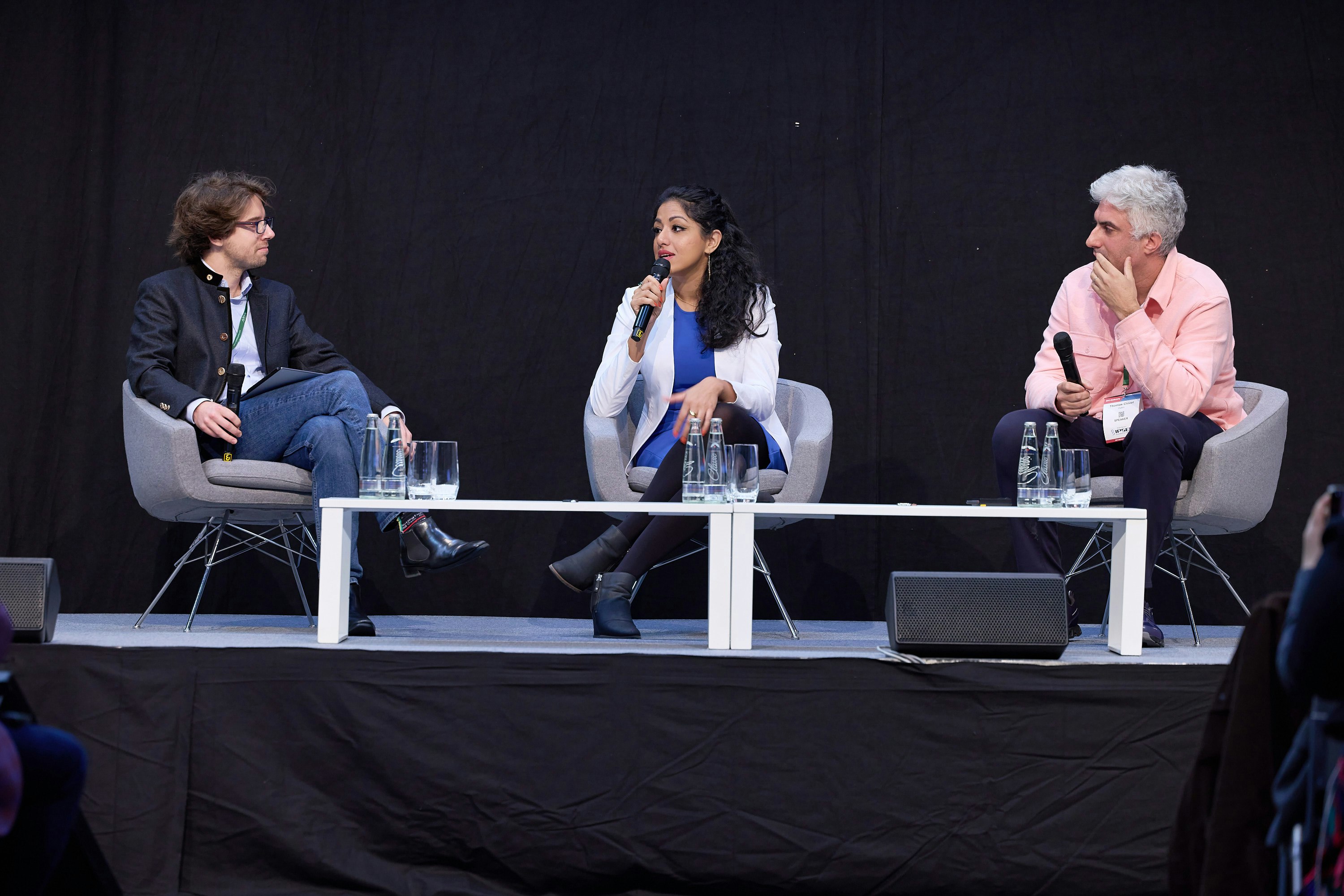
The biotech industry has a problem, navigating through a period of increased risks and challenges in funding, drug discovery and development, and commercialization. Now, more than ever, data matters most.
The lifeblood of biotech, data are the building blocks that drive breakthroughs and fuel advancements. In the labyrinth of biological complexities, data acts as a guiding beacon, enabling scientists to unravel the mysteries of diseases, drug interactions, and molecular structures.
Despite funding reductions in the healthcare space, a surge of digital innovation funding, such as the $200 million Sofinnova Digital Medicine Fund, has ushered in a potential solution to mitigate the challenges and harness our data currency – Artificial Intelligence (AI).
It brings a tantalising promise: Alexandra Kemp, Partner at McKinsey explains “There is a potential to completely reshape how we find and develop drugs”. We are only at the tip of the AI iceberg, and if leveraged effectively, it might be arriving at the right time.
It’s Not a Stupid Question: What is AI?
AI refers to the simulation of human intelligence in machines programmed to think, learn, and solve problems independently. AI learns and operates based on the nuances of human language, AI models for biotech are trained on ‘in-silico’ data, bridging the gap between the language of chemistry and biology, encompassing molecular structures, compound interactions, and biochemical mechanisms. Such models analyse molecular data, understand chemical properties, and predict how compounds might behave within biological systems.
The Urgency For AI In Biotech
With increasing risks in the industry, such as investors becoming more conservative, there’s a need to reduce the cost of R&D while simultaneously enhancing productivity. Compressing the life cycle of drug development is vital and our industry demands innovative solutions.
The sheer volume and complexity of biological data today transcend human capacity, making it imperative to harness the power of AI-enabled data analytics.
AI Use Cases in Biotech and Beyond
- Driving Drug Discovery
AI's prowess lies in handling complex data sets that surpass human comprehension, tackling intricate design problems. AI can assess what molecule could bind to a specific protein and stop it misbehaving by connect the dots in scientific literature and huge data sets. This gives it the potential to identify more targets and candidates quicker – accelerating timelines and reducing cost. Generative AI can unravel complex biology to prompt entirely new ideas for drug targets based on a better understanding of disease causality.
AI-driven R&D efforts from discovery to preclinical could deliver time and cost savings of at least 25-50%
Rather than a linear progression from lead optimisation to ADME evaluation, predictive models also enable optimisation for multiple properties in parallel.
Lastly, the novelty of both discovered therapeutics, and the targets scientists select for, could also be aided by AI. By exploring a wider chemical/biological space than humans can, AI models might create innovative structures like bi or tri-specific antibodies.
- Digital Medicine (early diagnosis, precision medicine and patient stratification)
AI paves the way for digital health – early diagnosis and precision medicine; including stratifying patients and defining appropriate endpoints for personalised treatments. This can augment a physician’s insights into an individual’s health, potentially identifying early-on diseases that they may be susceptible to.
One compelling instance is in Alzheimer's disease assessment, a realm traditionally clouded by subjectivity. J&J's groundbreaking 10-minute AI-enabled assessment offers a revolutionary shift towards objectivity, enabling the identification of early Alzheimer's stages with unparalleled precision. Furthermore, AI enabled ECGs show potential to diagnose patients faster than traditional methods, which have in some instances led to misdiagnosis periods of up to 4 years.
Beyond diagnostics, AI excels in diversifying clinical trials, efficiently recruiting and enrolling patients that meet the selection criteria.
It can also aid in aligning biological intricacies with diverse patient populations, determining the specific cohorts that would benefit from a drug with the capability to define multimodal biomarkers. This could reshape the landscape of tailored and effective treatments.
Adoption Of AI In The industry
Some pharmaceutical companies have embraced AI, leveraging it to revolutionize drug discovery, and others have adopted an 'AI-first' approach.
Najat Khan, Chief Data Science Officer and Global Head, Strategy, Portfolio & Operations, R&D at J&J explained researchers are applying AI and Machine Learning (ML) across the company’s pipeline.
A collaboration with the UK BioBank uses a multi-modality approach, with AI connecting causality with data and in turn using big data to build a multi scale foundational model which can show novel correlations between genomics (input) and proteomics (output).
With this ‘ML-enabled’ inter-connectedness the company has identified 6-7 targets that were previously overlooked, observing evolution from initial disease hypothesis to target identification and phase 1 development occurring within a mere 13 months (traditionally this can take up to 5 years) – signifying the rapid advancements in AI akin to the nascent stages of the internet.
The company has also applied AI algorithms to large, anonymized, datasets to identify and locate clinical research sites with patients who could potentially benefit from medicines being studied.
On the smaller biotech scale, Exscientia is a world leader of a new discipline; pharmatech, the interface of advanced AI application and complex drug discovery. It was the first company to automate drug design and the first to have an AI-Designed molecule enter clinical trials, with a lead programme for adult patients with advanced solid tumours. The company has also entered into strategic collaborations with Merck and Sanofi.
Insilico Medicine, a clinical stage AI drug discovery have developed a fully-integrated drug discovery software suite ‘Pharma.AI’, which has contributed to 31 AI-designed drugs in their pipeline.
The Future
Barriers will need to be addressed to unlock the full potential of AI, such as a perceived lack of value proofs in drug discovery and prevailing uncertainty around AI in general (such as ethical dilemmas and regulatory challenges). Applying AI to commercially less attractive therapeutic areas is a challenge that requires strategic consideration.
Despite this, there is broad consensus on the future potential of this technology encapsulated by the words of Richard Law, CBO at Exscientia: “In the future, all drugs will be designed using AI”. The rise of in-silico data is bridging the language of biology and chemistry, uncovering new truths about the fundamentals of disease biology which can shape drug discovery.