Is Artificial Intelligence The Future of Finance?
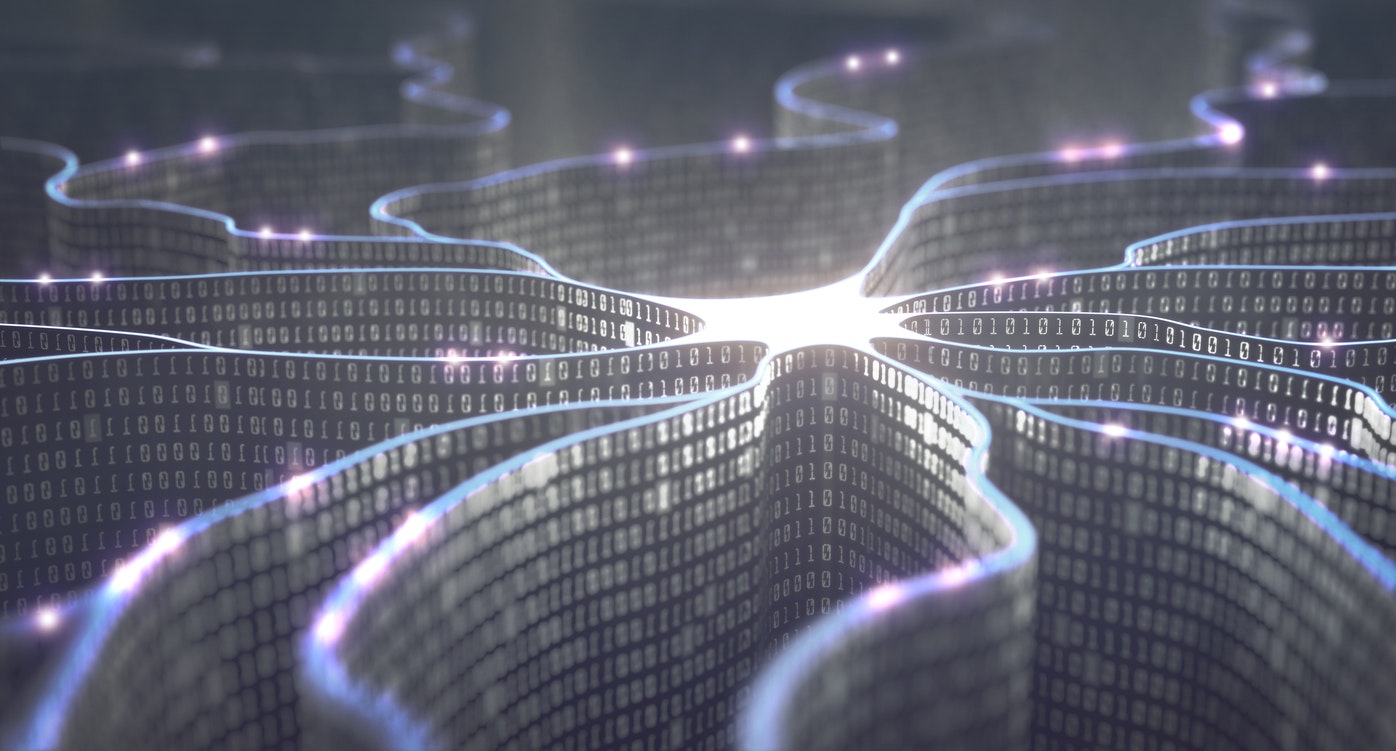
Sviatoslav Rosov, PhD, CFA, is an analyst in the capital markets policy group at CFA Institute. He is responsible for developing research projects, policy papers, articles, and regulatory consultations that advance CFA Institute policy positions, focusing on market structure and wider financial market integrity issues.
The topic of machine learning-enabled artificial intelligence (AI) is gaining visibility in the world of investment management. Of particular interest is the application of AI to development of smarter robo-advisors that some hope, while others fear, will yield ‘intelligent’ and cost-effective investment management advice. This topic was raised by investment professionals during a recent CFA Institute traveling conference in Central and Eastern Europe and the Middle East. AI has also been the subject of an European Commission (EC) consultation document, to which CFA Institute submitted a response.
What Is Machine Learning?
Machine learning is a technique that researchers, and now firms, have begun using to design more intelligent computer systems. Instead of attempting to hard-code a computer with all possible scenarios and actions a machine may encounter when trying to perform a certain task, machine learning allows the computer to ‘learn’ the necessary relationships and actions involved in completing a task intelligently. This ‘training’ involves using a large data set that the computer algorithm can repeat (typically with guidance and supervision) to learn through trial and error how to connect input data (e.g., credit history, employment history, assets, purchasing history) with the desired output (e.g., the correct identification of a suitable risky portfolio). Once this is learned, the algorithm can be used on real-world data with surprisingly good (in some cases) results.
Neural Networks
The most popular approach to machine learning is using neural networks, computer algorithms designed to mimic the way we believe the brain processes information. The networks are created by connecting millions, if not billions, of artificial neurons (essentially input/output switches) in all possible combinations. Through the process of supervised trial and error, certain connections are strengthened, others weakened, and some removed until the correct network of switches and connections is left that can accept an input and identify the correct output.
In the world of fintech, common use cases for machine-learning algorithms are credit-scoring models for fintech credit companies (e.g., marketplace lending or peer-to-peer lenders) and robo-advisors. In the former, hundreds of input parameters about the individual (including, in some cases, Facebook and Twitter usage) are fed into a neural network that attempts to find patterns unobservable to humans but that are correlated with credit-worthiness. Whether these types of neural networks really work has yet to be tested by a full credit cycle. In the case of robo-advisors, similar input parameters about the individual investor are considered by a neural network designed to assess suitability, risk tolerance, and appetite. Alpha-generating algorithms are a separate angle in which machine learning is being developed in the field of investment management.
We’ve Seen These Issues Before
One issue relating to neural network-based AI applications in investment management is the black box, in which the workings of an algorithm are not understood by its user and lead to potentially unintended actions or consequences. It’s a well-known headache for regulators trying to ensure market stability. Although some attempts have been made to check the source code of algorithmic traders, the most effective protection against algorithmic errors are circuit breakers on markets that limit the damage a failing algorithm can cause.
We highlighted in our response to the EC’s consultation document on fintech an example from the world of algorithmic trading on circuit breakers as a de facto solution to the black-box challenge. For neural networks, the issue is even more complex. Although an algorithmic trader is coded by a human, a neural network evolves by itself in response to its training regime.
Regulatory Implications
Even if a regulator would have enough expertise and resources to evaluate, monitor, and police AI algorithms market-wide, it is likely to be fundamentally impossible. The EC consultation asked for opinions on whether a technologically agnostic approach to financial services regulation is desirable. This approach is something CFA Institute has previously advocated for. The development and adoption of new technology in financial services appears to be accelerating, and there’s little chance regulators will be able to ‘pick winners’ or craft tech-specific regulations in a timely manner. We think the only effective solution is regulations that apply regardless of the medium of the service delivery and, like circuit breakers on equity markets, allow some degree of failure without contagion.
This article first appeared on Market Integrity Insights, a CFA Institute blog about capital markets.