Moving from Eminence-Based to Evidence-Based Healthcare
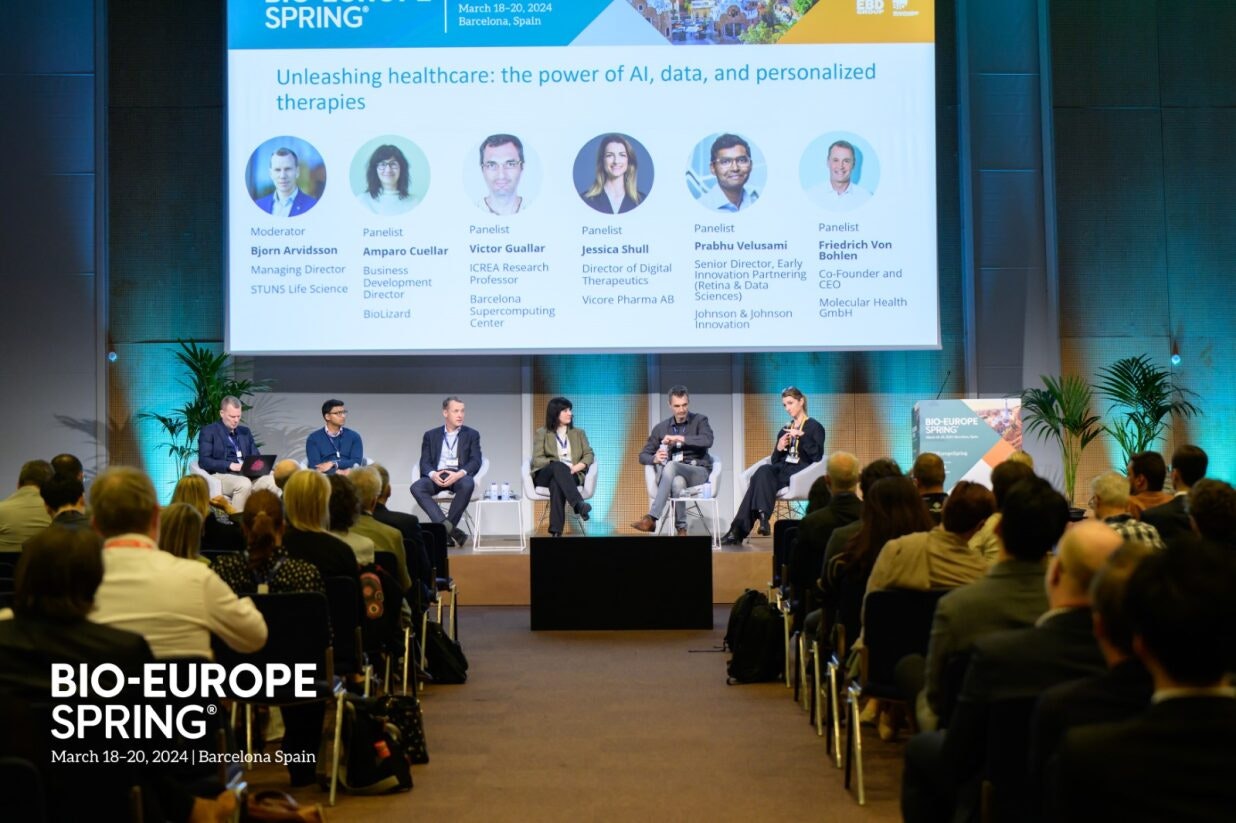
At the 2024 BIO-Europe Spring conference in Barcelona, panel participants discussed the movement towards data-driven decisions in healthcare and what it means for industry partnerships.
Medical decisions made purely based on a specialist’s opinion—or that of any prominent health professional—rather than on a critical appraisal of scientific evidence, have largely become obsolete. According to participants in the “Unleashing Healthcare: the power of AI, data, and personalized therapies” panel at 2024 BIO-Europe Spring, the same trend applies to decisions made in the discovery and development of new drugs and diagnostics.
Outlining the development of healthcare technology over the last century, panel moderator Bjorn Arvidsson, Managing Director, STUNS Life Science, touched on the evolution of medical diagnosis and treatment to a point where it is becoming largely data driven.
“We are moving from what I call eminence-based healthcare to evidence-based,” he told the audience. “Development is often carried out by small and agile companies with little or no power to take their products or services to the healthcare market and thus need to partner with larger launching machines like big pharma or at least larger companies.”
High-quality data for use with AI systems is the prevailing currency-in-trade for pharma industry growth. What does this mean for biotech and medtech companies with AI-enabled research projects and platforms ready for partnering?
Finding the Right Use Case
“Use case is everything,” said Prabhu Velusami, Senior Director, Early Innovation Partnering at Johnson & Johnson Innovation. “You've got to figure out what your technology or platform is offering that your potential partner can't do by themselves.” J&J has a lot of data scientists, Velusami said but there are still many things that large pharma organizations can’t do well.
For example, curating data is important because accessing lots of different data sets such as federated data is hard for large organizations to do, he said. “My advice is to engage early, be flexible on use cases, have an idea what use cases you may be applicable for, or where you feel your greatest strength is, and then have that open discussion with a partner to see if it docks into their interests at that given moment in time,” Velusami said.
Data Integration
Friedrich von Bohlen, Co-Founder and CEO of Molecular Health agreed that when it comes to AI, both the right use case and data integration are key. The use of AI in orthogonal data sets, or uncorrelated data, for example is still difficult.
“AI can learn very, very fast on a single data set in healthcare, such as text data, image data, numbers, DNA sequences and protein sequences,” he said. “But to get an answer in integrating such data, you need to very carefully apply AI in subsets of a use case or question. Will there be a time in the future when AI can integrate and do more than that? Absolutely, yes. But that time is not yet today,” he added.
Data Interpretation and Application
Building on these examples, Amparo Cuellar, Business Development Director at BioLizard explained that as more and more data are being generated, there is a gap between the vast amount of data companies can access and how to interpret it, how to make actionable outputs out of the data.
“It's very important to be able to explore what data you have, identify the use cases, and the quick wins in terms of bringing value into the data that has been generated,” she explained. “Also very important is to make sure that there is alignment, not only with partners that you will have, but also internally within the company, to make sure that everyone is at the same page and there is a clear data driven strategy in organizations,” Cuellar said.
The limits of AI are raising awareness of the need for alternatives such as better machine learning techniques, said Victor Guallar, ICREA Research Professor, Barcelona Supercomputing Center. “We are just starting to learn how to apply data, and every day there's one new layer of data that we want to integrate in our multi-layer of data, and then suddenly these things make you completely reorder everything and new,” he said.
Jessica Shull, Director of Digital Therapeutics, Vicore Pharma agreed. “It's very new, and a lot of regulators don't quite know how to approach it,” she said. “They're coming up with the regulations now, and this is constantly evolving. But the best practices are being established now.”
Although not represented on the panel, we got the chance to catch up with Syntekabio, an AI drug discovery pioneer, in Barcelona as well. “Scalable and efficient AI tools require the seamless integration of algorithms, big data, and robust supercomputing capabilities,” commented Jonathan Witztum, PhD, CTO of Syntekabio USA Inc. “When this synergy is achieved, AI can help to significantly streamline the drug discovery and development process.”
AI driven drug discovery and drug development is surely the future, but there is still a lot of ordinary strategy and thinking that needs to be applied before you can understand how to use AI and machine learning technologies to answer questions. This is where partnering comes in, said Victor Guallar of ICREA.
The Role of Partnering
Standalone AI based on data very rarely will work unless the data is very good and well curated, which it is not true in most cases, Victor Guallar said. “You need to really improve it, reinforce it, and get second opinions that can help AI to do the job that it does, which is super-fast analysis of all this data.”
Dr. Hubert Birner, Managing Partner at TVM Capital Life Science, who attended the conference but not this panel, agreed on the limitations of standalone AI. “AI alone is not a magic silver bullet for research progress,” Birner said. “Innovative startups need people with core expertise in the areas where AI is used.” For example, capability building by research scientists is critical as AI is merely an enabler and not an independent value driver. For this reason, TVM Capital Life Science invests in companies where AI serves the core product development. ROI expectations remain the same as for other VC investments, but AI offers the advantage of accelerating growth and reducing time to significant data, all while decreasing costs, Birner explained.
Coming back to how partnerships can be instrumental in getting the best out of AI and machine learning technologies, Victor Guallar said partnering helps provide balance. “What I see quite often through talking to big pharma is that they may have five data scientists, but they don't have the pressure to be at the edge. When you contract a company that lives off that, they are really on the edge of making this work,” he said.
In a large organization, you normally have data scientists who know data science, and people in the business, the biologists, who really understand the biology, said Prabhu Velusami of J&J Innovation. “But honestly, one doesn't necessarily know what the other one actually wants and can do. Ideally, you have someone who's an integrator, someone who has a good enough knowledge of the biology, and someone who has an understanding of what data science can do,” he stated.
Amparo Cuellar of BioLizard agreed that it is paramount when looking for partners and collaborators that there are stakeholders on both sides to be able to translate. “What the scientist in the lab is running and what end result he or she wants may be very different from how a computer scientist might be thinking,” she said. “It's very, very important to agree on the big picture and have all stakeholders aligned.”
What’s Next?
As the volume of healthcare data continues to grow, much of the way drug R&D currently works will change. Primarily, all life science companies need to jump into bioinformatics either directly or through a partnership or service provider. Beyond that, the accumulation of data will eventually get to a point where new data become unnecessary.
In a somewhat provocative statement, Prabhu Velusami argued that as AI tools predict the successful or unsuccessful outcome of clinical studies with increased accuracy, they will fundamentally inform R&D investment decisions in the next year or two. Particularly, funding for clinical trials. “I understand all the hard work and all the emotions around giving up a clinical trial where one has worked for ten years of their life,” he said, “but this will be part of the new reality in this industry.”
Improved patient stratification and biomarkers either pre-clinically or in the clinic are expected to improve clinical trial rates of success at J&J Innovation. “You're applying this to make sure it gets to the right people,” Velusami said. “And it also helps with your regulatory market access case.”
Victor Guallar of ICREA said that based on what is already being seen with the expanding use of patient data, the use of synthetic data will become more common. “Because of all this data, these different layers of data, and because of machine learning techniques, not everything is AI, not everything is artificial,” Guallar said.
So called 'real' data, won’t matter in five or ten years because there will be so many ways of developing synthetic data. This will shift predictions slowly, in an iterative manner, and lead to better and better and better results, he explained. “You're going to have such good predictions that you won't need more data at some point,” he concluded.
Bjorn Arvidsson ended the panel with a reference to Buckminster Fuller, a US-born architect and systems theorist who first identified the "knowledge-doubling curve" in 1982. Fuller calculated that human knowledge doubled approximately every 100 years until about 1900. Knowledge continues to increase at an ever-accelerating pace, he said. "IBM also did a prediction with the Internet of things that eventually we will double information every 12 hours," he said. Clearly, we are in the midst of a transition from linear to exponential growth of human knowledge.