Machine Learning in Finance Workshop
Led by Blanka Horvath
Monday 18 November
Your workshop leader
Blanka Horvath
Associate Professor in Mathematical and Computational Finance
University of Oxford
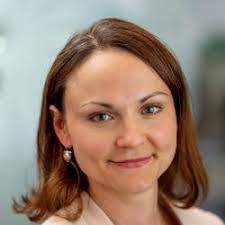
This site is part of the Informa Connect Division of Informa PLC
This site is operated by a business or businesses owned by Informa PLC and all copyright resides with them. Informa PLC's registered office is 5 Howick Place, London SW1P 1WG. Registered in England and Wales. Number 3099067.
Monday 18 November
Blanka Horvath
Associate Professor in Mathematical and Computational Finance
University of Oxford