The top trends that will impact asset management in the future
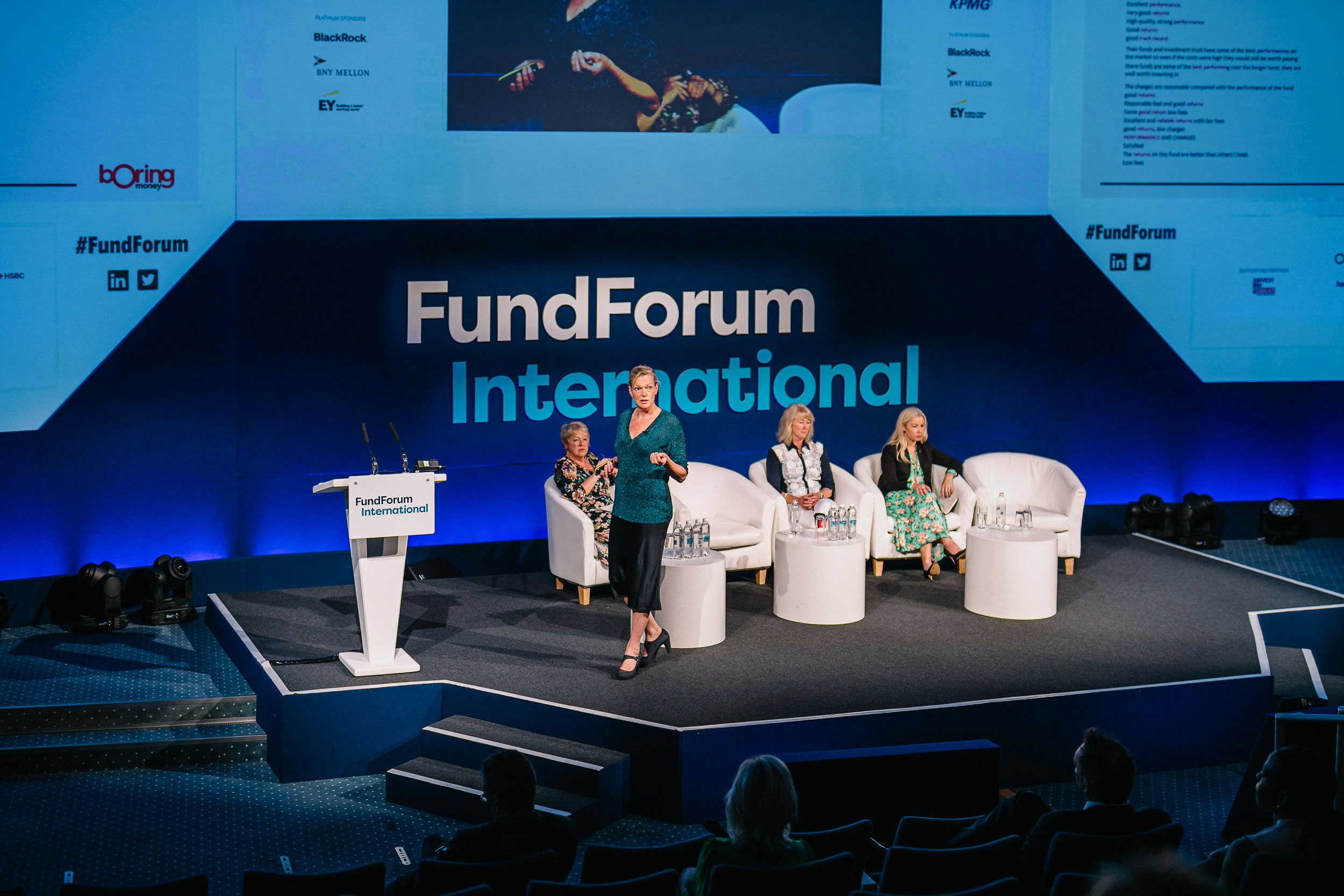
Asset managers are used to being told that the data revolution will transform their industry. At the FundForum International 2019 in Copenhagen they were given an insight into how. Our FundForum writer, Alex Thornton, was onsite to report first-hand.
A series of panels and presentations on Data and Next Gen Asset Management looked at examples of various ways digital technologies are being used to improve performance, reduce costs, and deliver more for investors.
From data analytics to deep learning, AI driven funds, and the always controversial blockchain, here are some of the trends to watch out for:
Data analytics exist: how to use them?
Fund managers, like everyone else, have habits and biases that are difficult to ignore. Often we are unaware of the assumptions and mental shortcuts that affect what we believe are entirely rational decisions.
Data analysis can help to identify those biases, so that it’s possible to overcome them. Clare Flynn Levy, founder and CEO of Essentia Analytics, has been helping funds to get the best out of their managers by holding a mirror up to their behaviour.
By analysing the alpha life cycle, she has identified four distinct personalities. Every manager will be a mix of all four, but will tend towards one more than the others.
The coaster steadily accumulates alpha until about halfway through a position, at which point it flattens off – a sign that the position has been held too long.
The hopeless romantic starts with very rapid alpha growth at the beginning, which then falls away dramatically to destroy value. The position is held in a desperate hope that the initial gains will repeat, and is typical of loss averse behaviour.
The round tripper builds alpha until about 60% of the way through a position, at which point the gains go into reverse and are lost. This is the most common pattern even among very good managers, consistent with getting comfortable with a position and being slow to get out.
The rarest is the linear accumulator, where alpha just keeps going up, and is still rising when the position is sold – possibly too early, or possible just at the right time.
When these signs appear, the manager can be alerted, and prompted examine the investment. The patterns are only aggregates, so will not tell a manager what to do in any given situation. But they will be spurred into making active decisions about what to do with that position, and think hard about whether they are genuinely want to stick with it, or whether they are being driven by their natural biases.
A manager willing to be honest with themselves will learn a huge amount about their own behaviour from data, and can use that to improve their performance. Other biases, such as the endowment effect (a tendency to value something too highly just because you own it) and confirmation bias (giving greater weight to evidence that supports what you already believe).
Similar use of data can show managers whether they are losing out on gains by scaling positions too slowly because of a natural caution. As data analytics can spot these trends in real time, managers can see a significant performance boost.
Another promising use of data analytics is in assessing an investor’s risk tolerance. People are notoriously unreliable when stating their tolerance for risk, with significant gaps between what they think they will accept, and what past behaviour shows they actually feel comfortable with. Managers can use analysis of past transactions to close that gap, and reduce regulatory liability by more accurately assessing whether a product or strategy is suitable.
The machines are here to stay
The principles of machine learning are not new, but the capabilities have been dramatically enhanced in recent years by developments in deep learning through complex neural networks. Computers are trained with large data sets and identify their own corresponding signals and rules. Many of these will be the same signals that people use. But intriguingly some are not.
"While many of the technological innovations on show were warmly welcomed, there was a decidedly mixed response to blockchain."
In either case, computers can be used to sift through vast amounts of data very quickly and identify potential patterns. Several uses were showcased at the FundForum International, including attempts to analyse news stories, predict which types of balance sheet information were more relevant to particular sectors, and screening fundamental data for weak signals that could be turned into strong signals.
Sandrine Ungari, Head of Cross-Asset Quantitative Research at Société Générale, pointed out the work being done on machine learning in alternative risk premia.
It is also being used in ESG for sentiment analysis, screening for controversies in real time, and analysing real-world data such as satellite photos.
As with any tool, machine learning can be used in the right way and in the wrong way. Used appropriately it empowers managers to make better decisions.
AI-driven funds
Funds are being developed that take the decisions out of managers’ hands altogether. The founders of a number of AI driven funds shared their experiences of technology that makes quant seem traditional by comparison. They represented a diverse range of strategies, from a Paris based fund that has trained a computer to spot short term price fluctuations in intra-day trading, to Sweden’s first AI hedge fund that uses reinforcement learning to add single digit improvements to alpha.
Fund managers, like everyone else, have habits and biases that are difficult to ignore.
Unlike quant funds, the AI is not coded with a fixed set of rules. Instead, it develops its own, which are then traced back to a sound economic hypothesis to see if they can be explained.
There are lessons in their common approach that are relevant to all asset managers in the face of new technology. The key feature was that all were willing to try something, fail, learn from the experience, and try again. This scientific, experimental approach is key to their ability to innovate.
The talent needed for this kind of work does not come cheap. It was revealed that among machine learning and AI graduates, there is a perception that finance doesn’t get the cream of the crop – they are being scooped up by the tech giants at eyewatering initial salaries.
The current state of Blockchain
While many of the technological innovations on show were warmly welcomed, there was a decidedly mixed response to blockchain. Digital Ledger Technology is suffering badly from its association with cryptocurrency scams and the hype of recent years, with many of blockchain’s proponents keen to point out the difference between the underlying technology, and its less savoury uses.
Kate Webber, Head of Product Development at Calastone, is among those who believe that the benefits of blockchain are undeniable. Calastone puts all its transactions through digital ledger technology, and is rolling out early adopter programs to interested clients.
Blockchain has the potential to make transactions cheaper, quicker, more secure and more transparent. It can create secondary markets in new areas, reduce operational risk, and be used to build trust in financial transactions. But first it needs to shake off the lingering association with cryptocurrencies.
See Edward Glyn, Managing Director, Head of Global Markets, Calastone, discuss below how the company became the largest financial community operating on DLT overnight.