Seven women forging the future of healthcare with AI
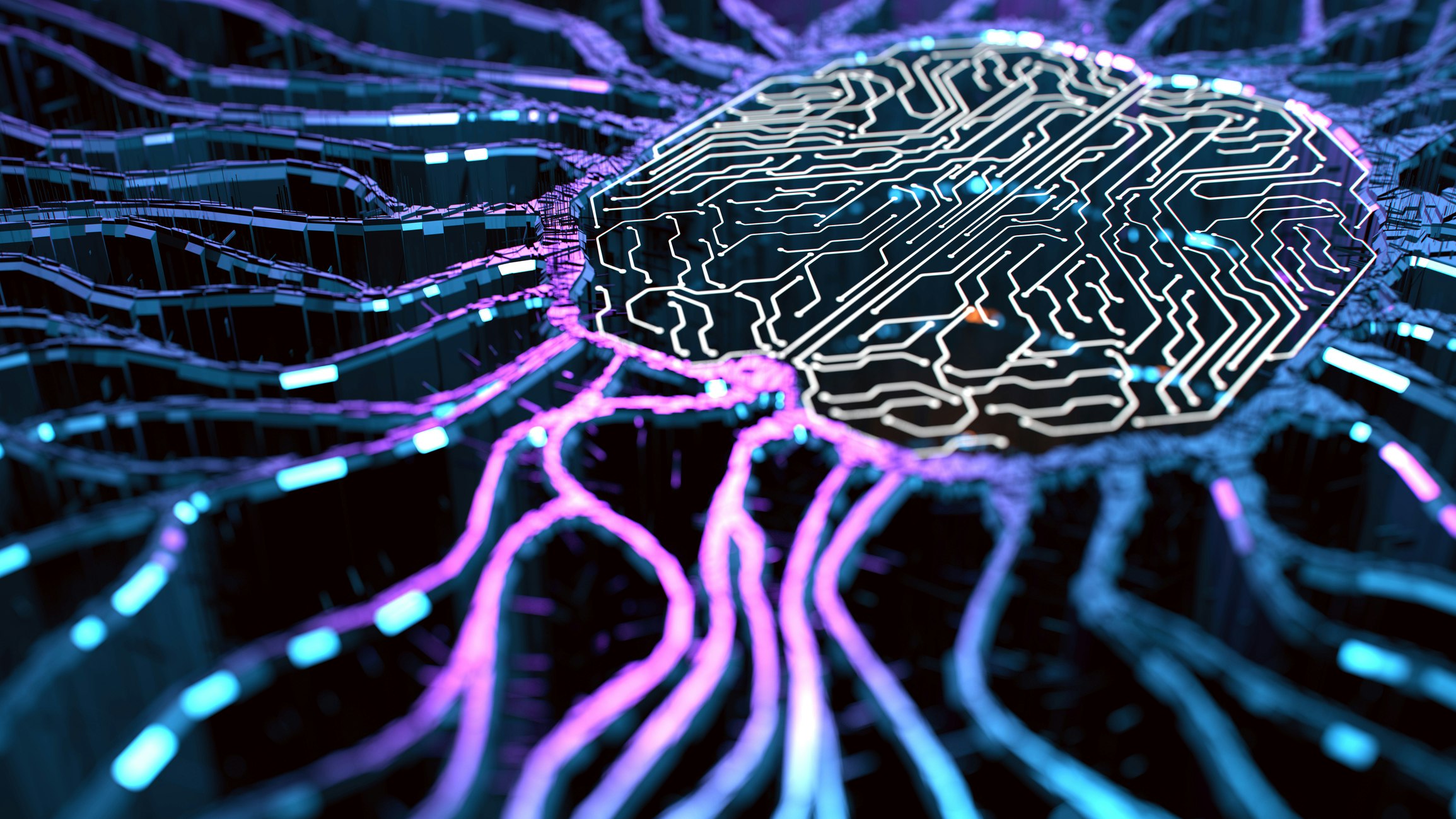
Of all the ramifications that artificial intelligence is beginning to have on our world, the most important by far - for obvious reasons - will be its impact on human health. AI has become a new tool that healthcare workers, biotech researchers, pharma professionals and now even consumers are utilizing. A TechEmergence study of healthcare industry executives “found that over 50 percent anticipate broad scale AI adoption by 2025”. Women, although only about 13.5% of the entire machine learning field, are making great strides in the field of Artificial Intelligence in pharma and healthcare. From CEOs of biotech companies to machine learning engineers, I profile seven women who are solving some of healthcare’s biggest problems ranging from Global physician shortages to the misdiagnosis of disease to making sense of big data.
Barbara E Engelhardt PhD, Associate Professor, Department of Computer Science & Center for Statistics and Machine Learning, Princeton University - @BeEngelhardt
“AI has a profound ability to impact the way we think about healthcare. My group is broadly focused on a number of different areas in AI and healthcare. First, we are developing approaches to model the state of a patient at any time. What this means, in terms of the models we use, is that, for any patient at any time, we note the set of vital signs and lab results that were measured previously, and we use what we know of this patient and other similar patients to guess at the value of important patient traits, such as heart rate, blood pressure, or white blood cell counts, and also our uncertainty in those guesses. These patient state estimates will allow caregivers to make informed decisions about necessary tests, medical interventions, or procedures.
The second aspect of our work involves identifying treatment policies for patients. Currently hospitals provide guidelines for caregivers to treat specific patients, but those broad guidelines often miss patients with non-canonical presentations or positively diagnose and treat patients that do not require treatment. We use machine learning approaches to create new treatment policies. Importantly, these policies are not what we see from doctors, and an important aspect of this work is to find a way to test our policies in a hospital setting without putting patients at risk. A related thread of our work in this area involves considering how patients respond to specific interventions, which is an important predictive model to build because we do not want to expose patients to all possible interventions and put them at risk. This response model allows us to play out a number of different treatment options for patients to see which one will have the best outcome with the least toxicity.”
RELATED POST: Artificial intelligence's potential role in the revolution of drug discovery - WHITEPAPER
Veronika Cheplygina, Assistant Professor, Medical Image Analysis Group, Eindhoven University of Technology, The Netherlands - @vcheplygina
“I’m using machine learning to detect abnormalities in medical scans, for example CT scans of the lungs. The problem is that machine learning methods need a lot of examples – scans where abnormalities have been manually outlined – to do this well. This adds an extra burden for the experts, so I’m looking into ways that machine learning can learn from other types of (annotated) data that is available. I’m especially excited about the possibilities of crowdsourcing, as this could also help to raise awareness about health and what machine learning can or cannot do.”
Anne E. Carpenter, Ph.D., Senior Director, Imaging Platform, Broad Institute of Harvard and MIT - @DrAnneCarpenter
“My laboratory is dedicated to improving the efficiency of drug discovery. We create algorithms to get the most of biological images. Many biomedical researchers have automated microscopes that work 24/7 capturing images of cells. I’ve worked with hundreds of biomedical researchers, each devoted to a particular disease - our software “looks” at cells and determines whether any of the drugs they are testing have beneficial effects in treating the disease in those cells. Seeing the impact of our work is so satisfying - for example, Recursion Pharma is a company that launched based on the software and methods my group developed.”
Jackie Hunter, CEO BenevolentAI - @benevolent_bio
“BenevolentAI is transforming drug discovery and development. Using its proprietary AI platform, BenvolentAI’s in-house team of researchers are able to quickly propose new treatments for disease from fragmented and unstructured data sets. The technology delivers efficiency savings of 60% and reduces the early stage drug discovery process by up to four years against pharma industry benchmarks. We’ve already demonstrated ground-breaking progress in ALS, and the rapid progression of a further 18 drug research programmes. The potential applications of our AI are virtually limitless – even beyond drug discovery – it is just a case of focus, scale and urgency.”
Becks Simpson, Machine Learning Lead Engineer, Maxwell MRI - @simmoroos
“In a world where the demand for top-tier medical expertise is rapidly outstripping the supply and where data is not used to tailor disease prediction, diagnosis or treatment options to individuals, Maxwell is aiming to make healthcare personalised, high quality and always on when people need it. To facilitate that, my work at Maxwell involves developing various deep learning models ranging from ‘patient embeddings’ (a learned mapping between all of a person’s data to how healthy they are) to identifying early indicators and risk of disease from medical imaging, genetics and more.”
Professor Karin Verspoor, Deputy Head of Department, Dept of Computing and Information Systems, The University of Melbourne - @karinv
“My work involves the application of methods from natural language processing to biomedical and clinical texts. It aims to transform this very special kind of data, produced by people – researchers or healthcare workers or patients themselves – for other people to read and understand, into structured information that can be used in more typical analytical approaches or sophisticated predictive models. Because so much of healthcare involves careful observation of patients and their history or symptoms, and those observations are often captured in free text – and maybe nowhere else! – it is critical to dig into that text and pull out details that can help to build a more complete picture of a patient’s health.”
Maria Dyshel, Multi-disciplinary technologist and Forbes 30 under 30 entrepreneur, Digital Catalyst, Sanofi
“My path in AI started from a research initiative to use machine learning to quantify Parkinson's disease symptoms. Since then, I have pursued my passion for AI and for healthcare separately: while my thesis focused on AI applications in energy, I've been involved in public health projects. I co-founded Genesis, a non-profit that strives to decrease genetic diseases incidence among the Bedouin population, and worked on developing a mobile application for nurses caring for newborns in rural India. I believe AI will continue to transform how we care for each other - both to prevent and to treat medical conditions. Most recently, I have joined the multi-national pharmaceutical company Sanofi, where I hope to continue growing my knowledge in applying advanced technologies to improve patients' lives.”