This is Your Brain on AI: How Neural Networks are Powering the Drug Industry
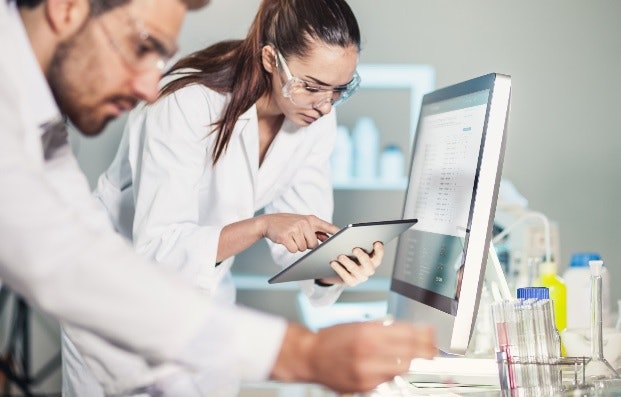
This is Your Brain on AI: How Neural Networks are Powering the Drug Industry - By Dekel Gelbman
A little information is a dangerous thing. But what about too much information? The healthcare industry is inundated with data–electronic medical records, genomic data, medical imaging, biometric data, scientific articles, study results–all representing opportunities to personalize disease prevention and treatment.
It can be admittedly overwhelming: researchers and clinicians at every level, including in the pharmaceutical chain, have endless options for where to focus their work, and endless dead ends as well.
Enter artificial intelligence. From identifying disease biomarkers to developing and commercializing therapies, artificial intelligence and machine learning is becoming indispensable for understanding and prioritizing the opportunities these mountains of data represent.
Deep learning platforms like Deep Patient have already shown their ability to efficiently analyze EHRs to predict schizophrenia and severe diabetes. Two different neural networks have been trained to work together to diagnose tuberculosis from x-rays. Face2Gene algorithms are using facial analysis and HPO terms to help doctors and pharma recognize patients with phenotypes related to diseases, such as Sanfilippo Syndrome (MPSIII). These technologies are improving the identification of biomarkers and phenotypes and accelerating the discovery of correlations between disease and the plethora of patient data available.
And once those correlations are made, AI can get to work researching treatment options. Iris.AI is effectively a research assistant–imagine Star Trek’s Data android rapidly scanning research from around the globe, but without the unnerving yellow eyes. These neural networks even go beyond examining the present to start theorizing. Atomwise’s AtomNet can design drug models to see if they’re likely to interact with relevant disease molecules. If they do in a 3D model, the models may be worth synthesizing and testing.
Of course, once AI helps research and proposes new drug treatments, they still need to be tested. Certainly none of these neural networks are swallowing the pills or wincing during injections, but they can help find patients for clinical trials. Systems like Trials.AI lighten the administrative load and guide patients. The same in-depth patient profiles AI helped humans create back in the discovery stage can be leveraged to find people most likely to respond to treatments–a huge benefit when 90 percent of blockbuster medicines only work for 30-50 percent of patients.
All told, AI’s application in pharma–more exactly, precision medicine– can reduce the cost of drug discovery to the tune of $26 billion worldwide. With humans at the helm, AI can help synthesize and analyze more data than researchers and patients could review in a lifetime.
As CERN’s Research and Computing Director Eckhard said, “Too much high-quality data may be a challenge, but it is a good problem to have.”
About Dekel Gelman:
Dekel Gelbman, CEO, FDNA, leads the corporate and business strategy of the company that turned an early stage computer vision technology into an innovative digital health platform and database used by thousands of clinicians, researchers and labs globally in the evaluation of genetic and other rare disease patients.