Artificial Intelligence for Data Analytics: Believe the Hype
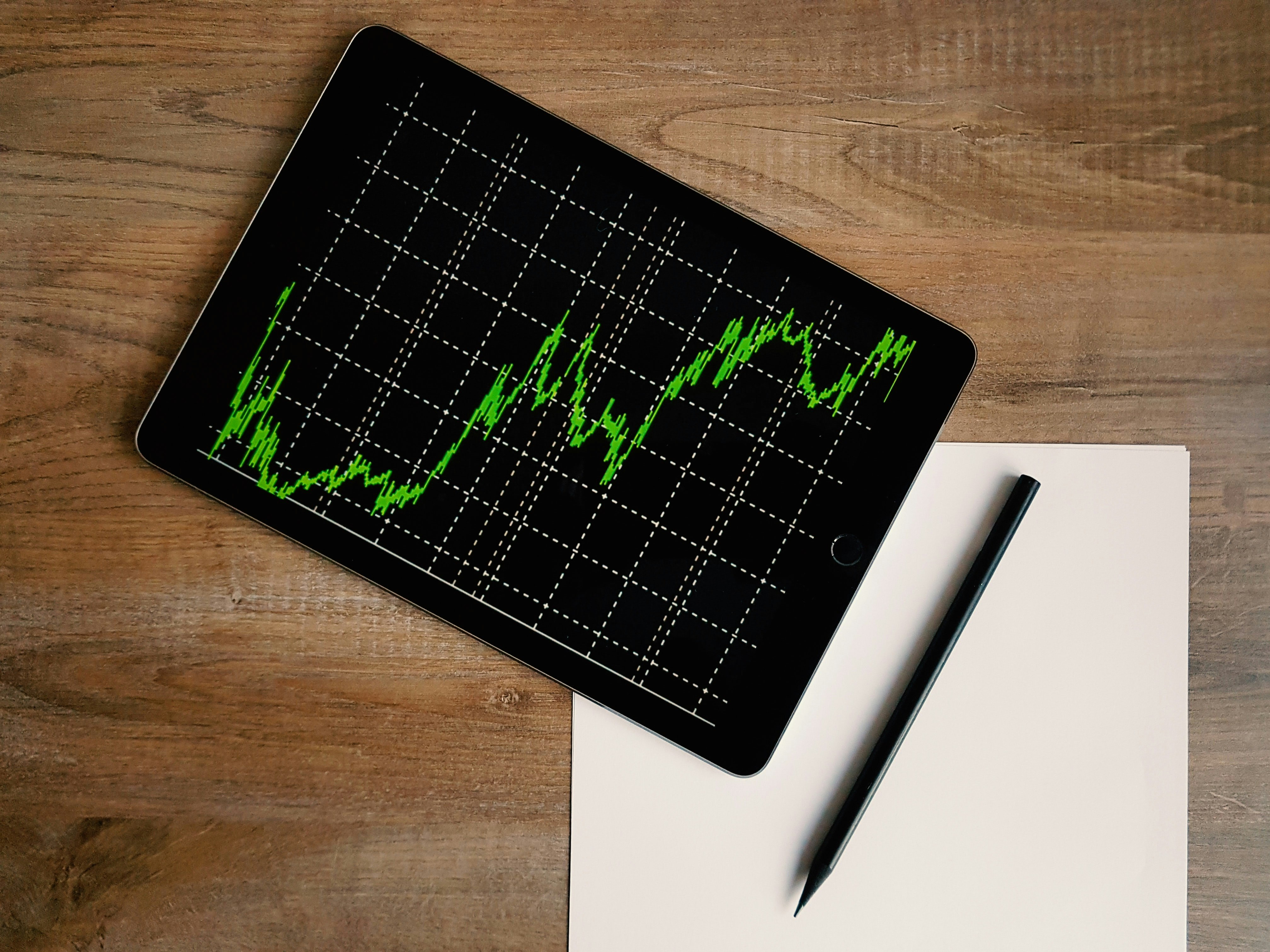
Believe it or not, artificial intelligence (AI) for data analytics is here to stay. Some technologies that have had such a quick upward climb can seem like a fad, take the smartphone, for example -- but can truly have transformational effects on business.
According to PwC, AI is poised to become the biggest growth opportunity in the fastest growing economies, projected to add $15.7 trillion in ten years. And, Gartner states that by 2020, augmented analytics will be a dominant driver of new purchases of analytics and business intelligence as well as data science and machine learning platforms, and of embedded analytics[1].
Large enterprises will be aggressively adopting automated business analysis
Why? Because it helps fulfill strategic goals around deploying AI to the enterprise, and due to existing competitive pressures in their industries.
Here's how I’ve come to that conclusion:
Businesses have hit a ceiling with the adoption of big data and analytics
After the adoption of big data and analytics, businesses today have hit a ceiling where they are no longer getting as much value out of their investments in these areas. This is the result of a combination of factors, including the explosion in the amount of data, and the complexity of data.
Consider "big data": the addition of more data sources, better (and more expensive) data storage, aggregation, and unification technologies has not yielded value in proportion to the amount of data stored. Or consider "analytics tools": the additional investment in tools has not kept up with the increasing amount and complexity of data either. For example, with dashboarding tools (and the like), there are only a fixed number of dashboards, no matter how pretty, or easy to set up, or intelligent, that we as human decision-makers can absorb and use.
The problem is us humans
So what is the underlying cause for these diminishing returns? In short, the problem is us humans. In particular, as humans, we have a limited amount of time and attention, and that fixed amount is already vastly outmatched by the data businesses have. And while there are many tools focused on helping analysts be more time efficient, we are hitting diminishing returns - there's only so much faster a data analyst can issue queries (and get responses) or make dashboards. That leaves the limited amount of attention as the remaining bottleneck that we can address. Phrased another way, the most effective path to greater value from analytics must come from improving what human analysts focus on.
Paralysis is in the analysis
Today, what a human analyst focuses on is largely either scheduled (e.g., reports due on a regular cadence) or reactive (i.e., in response to some change). The common thread to both of these sources of work is knowing in advance what to ask. Without a motivating question, analysts are usually paralyzed by the scale and complexity of the data in front of them. It is not uncommon to hear this refrain: What should I look for? And you can't blame them! The alternative is to repeatedly come up with hopefully promising questions, and attempt to answer those questions, until, hopefully, something interesting comes up that survives the scrutiny of deeper investigation. And again, with the scale and complexity of modern data environments, this is a task that is beyond human limits. To solve this problem, we must turn to AI to automate business analysis.
Automation to the rescue
Many businesses have developed strategic initiatives around the deployment of AI to their enterprises, and just as many have struggled to find solutions that deliver value quickly. Applying AI to business analytics (e.g., deploying automated business analysis) is an exciting area where businesses might find such an opportunity, because of the groundwork previously laid by existing big data efforts and analytics.
With automated business analysis deployed, business analysts will have a fresh set of promising investigations for their data every day, uncovering new potential opportunities and helping to resolve issues before they become larger. Businesses with automated business analysis will be able to operate in more experimental and exploratory ways, knowing that they have technology that will help monitor the changes they expected and, more importantly, surface the unexpected changes that inevitably happen in any complex system. In competitive (and only increasingly so) markets, the ability to find new data-inspired opportunities and more quickly resolve problems will be a strategic advantage that will be indispensable for businesses.
[1] Gartner, “Top 10 Data and Analytics Technology Trends That Will Change Your Business” Published April 11, 2019.