Data driven insights can make biofuels more competitive
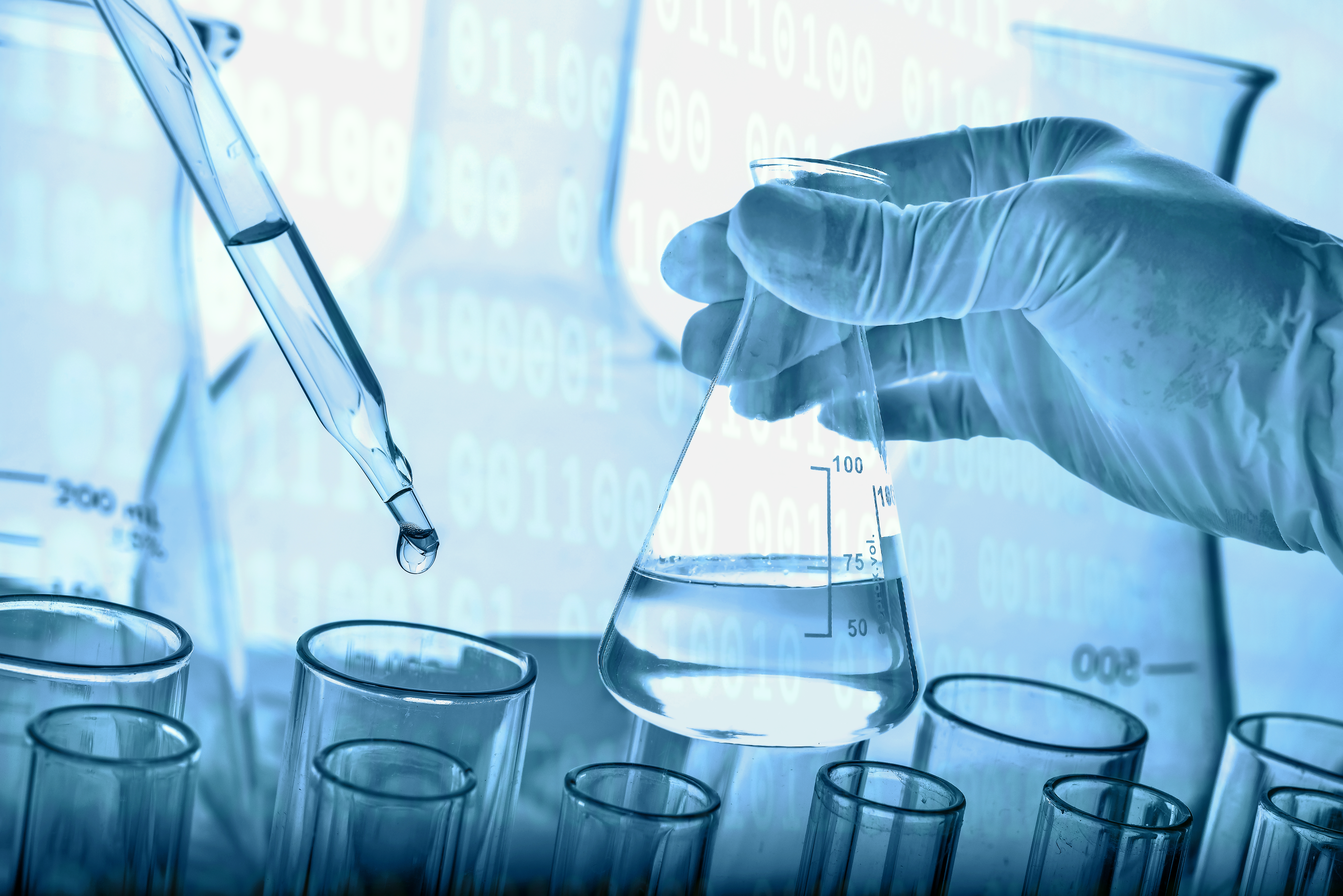
With the introduction of more sophisticated data driven processes, biofuels can be produced more efficiently, at greater scale, and at reduced cost. These types of solutions can be implemented at each stage of a biofuel’s development and production:
- In the laboratory - researchers are able to use data intensive automated processes to accelerate the pace at which useful feedstock strains can be identified and selected for.
- In the field - large data sets gathered from hundreds of individual farms can be used to help growers better understand how crops respond to varying conditions, thus improving yields.
- In the refinery - statistical analysis of data gathered from sensors in biorefineries can help to counteract human error, reduce waste and increase volume of output.
In this article, we consider each of these three stages in turn, showing how data analytics and biofuels can work together to compete against, and to some extent replace oil derived fuels.
Research & Development
A key indicator of a feedstock’s suitability for biofuels production is known as a crop’s fermentable yield. Fermentable yield takes into account the total fermentable sugar content of a crop, alongside the quantity of biomass that can be grown on a given land area, and the digestibility of a plant’s cell walls. While individually identifying sugar content or biomass yield is comparatively easy to do, gaining a holistic idea of how each of these factors together influence a crop’s fermentable yield is somewhat trickier, particularly in large feedstock populations.
That’s where data analysis can come in handy. In a paper published in the journal Biotechnology for Biofuels, researchers from Australia’s School of Environmental and Life Sciences outline what they describe as a “high-throughput screening framework for biofuel feedstock assessment” for the crop sorghum bicolor. In essence, the method amounts to taking small samples from the stalks of a selection of different sorghum plants, analysing them, and collating the results. By measuring a sample’s radius, the approximate height of the plant can be derived, while a process of spectral analysis and enzymatic hydrolysis is used to determine a sample’s sugar content and the digestibility of its cell walls. Each of these values is fed through a complex statistical analysis, allowing the total fermentable yield of a sorghum crop to be determined with accuracy.
A similar process can also be applied to high lipid feedstocks used in the production of biodiesels, such as bacteria, algae and fungi. Identifying more valuable strains (those with higher lipid content) through manual processes such as optical density measurements, bicinchoninic acid assays and gas chromatography assays is both time consuming and prone to error. Automating this process with high throughput screening allows the most desirable strains to be selected and propagated rapidly, accelerating the pace of research and reducing its costs. The result is that feedstock research is opening out to smaller competitors, each able to bring their product to market faster than previously thought possible.
In the Field
Developing high yield feedstocks is the first step in producing more competitive biofuels. The second step is optimising the conditions in which the crops are grown. Precision agriculture is a familiar catch-all phrase used to describe a range of different practices employed by some modern farmers. What each practice shares in common is that it involves the use of technology to assist farmers in making decisions that will influence the yield, quality and resilience of their crops. Such techniques include variable rate seeding & fertilisation, spectroscopic measurement of manure, GPS soil sampling, remote sensing technology, and drone photography, to mention a few.
All of these processes are also highly data intensive. While data about the conditions in a single patch of land can be very useful to the individual farmer, analysis of hundreds or even thousands of subsets from a large number of growing areas allows for the identification of patterns that will be overlooked on a smaller scale. One example is weed identification. For machine learning algorithms to recognise the subtle differences that distinguish weeds from food or fuel crops, thousands of images must be submitted for the algorithms to compare and contrast. Using such algorithms then allows farmers to automate the process of herbicide application, employing ‘smart’ agricultural equipment such as the See & Spray system developed by Blue River technology.
Another leader in the field of big data agriculture is the Climate Corporation, a digital agriculture company bought by agrochemical behemoths Monsanto in 2013. Corn feedstock farmers in the US can make use of the company’s Climate FieldView app to track key variables in their fields, such as temperature and soil moisture content. The app also pulls in data from satellites and weather monitoring stations to deliver predictions about the days ahead, and can provide real time recommendations about the application of water and fertilisers. What this means for biofuels is higher yields, reduced conflict with food crops, and more competitive prices at the pump.
During Production
Biorefineries already gather large amounts of data from each stage of the production process, including measurements of chemical composition during the fermentation process, data about flow rates, and continuous temperature tracking. Typically, this information moves through a centralised control system, allowing plant operators to monitor conditions and make any necessary adjustments.
Two developments are set to transform this approach: big data analytics applied to the fermentation process, and decentralisation of monitoring through IIoT (the Industrial Internet of Things). The first allows for classification of a large number of individual batches according to their performance. Once statistical outliers have been eliminated (as well as batches in which an unusual result is achieved for known reasons), the success factors for the remainder can be analysed in greater depth, and applied to future batches.
An example of a company successfully applying big data analysis to biorefineries is Xylogenics, a self-described provider of “plant fermentation modelling services”. Xylogenics utilise historical data and analysis of plant materials to build “lab scale” models of a biorefinery's fermentation process. By communicating with plant managers, and comparing data gathered about each refinery's fermentation process with data gathered from thousands of other refineries, Xylogenics are able to provide statistically informed recommendations for enhancing efficiency. Another advantage of this approach is that decisions made by operators responding to information gathered about individual batches can be analysed in a broader context, allowing for errors and procedural inconsistencies to be detected.
Introducing the IIoT to biorefineries eliminates human error in a different way – by delegating decisions currently made by human operators to inter-connected machinery. Efficiencies accrued through applying IIoT to biorefineries include reducing electrical costs through smarter load dispersal, and reducing staff costs through improved automation. IIoT connected systems are also better able to detect and respond to possible snarls in the production process, for instance by automatically shutting off critical systems to prevent damage in the case of an accident.
There is one caveat, however: big data analysis and IIoT systems will not be limited in their impact to the biofuels industry. As stated at the start of this article, data is beginning to play a central role in economic activity of all kinds. That includes biofuels’ competitors, from the petroleum industry, to electric battery manufacturers and producers of CNG for transport. If the biofuels industry is to gain an advantage from data driven solutions, it will need to step up its investment in them – and quickly.