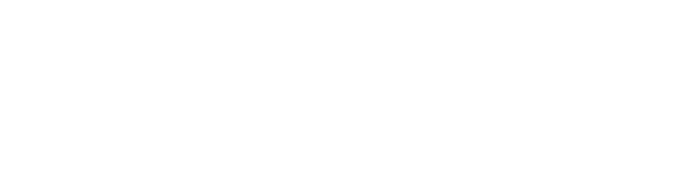
The nexus of finance and innovation
Alpha. Delta. Vega. Where the world of quant finance converges.
+
senior quant professionals
+
Asset managers
150+
Speakers
35+
countries worldwide
The #1 global quant finance event
QuantMinds International is back!
Join 650+ practitioners and academics from all over the globe, including 120+ asset managers for the world's leading quant finance event.
It's your once a year opportunity to move quant models forward. Discuss pricing and volatility, alpha generation, machine learning plus and much more.
Expert research & practitioner insights
The agenda explores all areas of quant: derivatives & volatility, option pricing, hedging, portfolio optimisation, alpha investing, inflation & interest rates, computational finance, credit risk, regulation & clearing, model risk and much more.
Tap into the leading quant minds
QuantMinds International proudly assembles the most brilliant quantitative finance minds from across the globe.
This prestigious gathering offers an unparalleled opportunity to engage with thought leaders who shape the industry's future.
Meet the Advisory Board
The top professionals from academia and industry are helping to advise on the developments of the 2025 programme.
This includes representatives from across banking, asset management, and academia.
Network like never before
The programme creates multiple pathways for meaningful professional engagement and knowledge exchange.
Closed-door discussions foster candid conversations on sensitive industry topics in a confidential environment. Interactive Q&A sessions with speakers enable direct engagement with thought leaders and experts. Dedicated networking breaks strategically positioned throughout the agenda maximise opportunities for spontaneous connections.
QuantMinds mini workshops
Get specialists insights on different quant areas from top experts.
The 2025 agenda focuses on machine learning, volatility, quantum computing, and climate finance
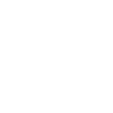
Highlights video
Hear firsthand how 600+ practitioners and academics from all over the globe, including 120+ asset managers, gathered to share the freshest theories and models in quant finance.
Specialist Summits. Specialist Solutions.
Get closer to the topics that mattered to you in one of the opening day summits or workshops.
Quant Tech Summit
Join leading industry practitioners, academics, and engineers as they provide an exhaustive agenda of the most cutting-edge technologies in the field.
Stay current with the best applications, engage in interactive sessions, and contribute to innovation in the industry!
Quant Invest Summit
Back by popular demand, the Quant Invest Summit is here to help funds, insurers and banks navigate through future waters.
From Multi-Asset Class Investing to Tick Data Management, hear from leading funds and banks and shape your quant fund.
Machine Learning in Finance Workshop
This workshop is designed for participants who are new to machine learning and want to acquire skills in this area. Learn about the foundations and core issue.
Led by Blanka Horvath & Mikko Pakkanen.
Rough Volatility Workshop
Get up to speed on everything from volatility derivatives to variance curve models and rough volatility.
Led by Jim Gatheral.
Be seen. Be heard.
Make use of this once a year opportunity to meet the biggest, most senior quant community.
Whether you want to get your brand seen, meet the people that matter to you or get measurable ROI and engagement - we can help.
For information on sponsorship opportunities please contact josef.lanjri@informa.com or call direct on: +44 (0) 20 8052 0424.


Interested in managing risk better?
Share a unique networking and exhibition space with the industry professionals attending RiskMinds International and gain 100s of new connections by joining the shared end of day drinks while enjoying the stunning views of the London skyline.
The QuantMinds digital community
Keep up with the latest in quant finance from our global network of experts. Sign up to our newsletter to receive the latest articles, interviews, session recordings, and more, directly to your inbox.
Get the Latest Event Updates
CERTIFIED BY THE CPD CERTIFICATION SERVICE
QuantMinds International is now a CPD certified event.
Attendees can request a CPD certificate after attending the event. For further information on CPD certification please visit: www.cpduk.co.uk
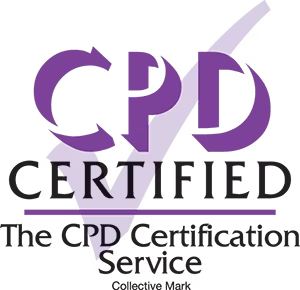