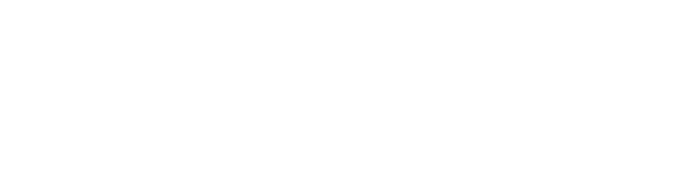
Where innovation becomes best practice
Derivatives and pricing, risk and hedging, alpha generation, machine learning, and more
+
senior quant professionals
+
Asset managers
150+
Speakers
35+
countries worldwide
The world's leading quant finance event
QuantMinds International returned to London!
We were joined by 650+ practitioners and academics from all over the globe, including 120+ asset managers for the anticipated return of the world's leading quant finance event.
It's your once a year opportunity to move quant models forward. Discuss pricing and volatility, alpha generation, machine learning plus and much more.
Expert research & practitioner insights
The agenda explored all areas of quant: derivatives & volatility, option pricing, hedging, portfolio optimisation, alpha investing, inflation & interest rates, computational finance, credit risk, regulation & clearing, model risk and much more.
Tap into the leading quant minds
QuantMinds International brought together some of the greatest minds in the industry. We picked their brains and shared insights on macro trends and micro developments to set you up for the future.
Our speakers included BlackRock, Fidelity International, Bank of America, Citigroup, University of Oxford, New York University and more...
Meet the Advisory Board
The top professionals from academia and industry are helping to advise on the developments of the 2024 programme.
This includes representatives from across banking, asset management, and academia.
Network like never before
We created new connections and catch up with industry peers.
Closed-door discussions, interactive Q&A with speakers, and dedicated networking breaks are just some of the ways you can exchange insights and build new relationships.
Plus the networking app which helps you reach them before, during and after the event.
QuantMinds mini workshops
Get specialists insights on different quant areas from top experts.
The 2024 agenda focused on machine learning, volatility, quantum computing, and climate finance
Highlights video
Take a look back at the 2024 event highlights...
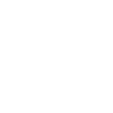
What else was on?
Get closer to the topics that mattered to you in one of the opening day summits or workshops.
LLMs & Advanced ML Summit
Join leading industry practitioners, academics, and engineers as they provide an exhaustive agenda of the most cutting-edge technologies in the field.
Stay current with the best applications, engage in interactive sessions, and contribute to innovation in the industry!
Quant Invest Summit
Back by popular demand, the Quant Invest Summit is here to help funds, insurers and banks navigate through future waters.
From Multi-Asset Class Investing to Tick Data Management, hear from leading funds and banks and shape your quant fund.
Machine Learning in Finance Workshop
This workshop is designed for participants who are new to machine learning and want to acquire skills in this area. Learn about the foundations and core issue.
Led by Blanka Horvath & Mikko Pakkanen.
Rough Volatility Workshop
Get up to speed on everything from volatility derivatives to variance curve models and rough volatility.
Led by Jim Gatheral.

Interested in managing risk better?
Share a unique networking and exhibition space with the industry professionals attending RiskMinds International and gain 100s of new connections by joining the shared end of day drinks while enjoying the stunning views of the London skyline.
Get the Latest Event Updates
The QuantMinds digital community
Keep up with the latest in quant finance from our global network of experts. Sign up to our newsletter to receive the latest articles, interviews, session recordings, and more, directly to your inbox.
Be seen. Be heard.
Make use of this once a year opportunity to meet the biggest, most senior quant community.
Whether you want to get your brand seen, meet the people that matter to you or get measurable ROI and engagement - we can help.
For information on sponsorship opportunities please contact josef.lanjri@informa.com or call direct on: +44 (0) 20 8052 0424.

Associate Partners
Exhibitors
Associations and Media Partners
CERTIFIED BY THE CPD CERTIFICATION SERVICE
QuantMinds International is now a CPD certified event.
Attendees can request a CPD certificate after attending the event. For further information on CPD certification please visit: www.cpduk.co.uk
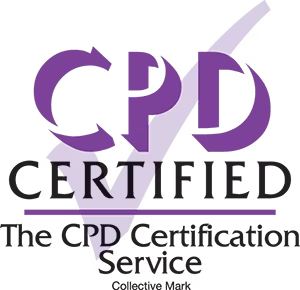